How do sEMG segmentation parameters influence pattern recognition process? An approach based on wearable sEMG sensor
Biomedical Signal Processing and Control(2023)
摘要
Processing surface electromyography (sEMG) data in real-time to control robotic devices in applications involving upper-limb prostheses is challenging, especially when the problem involves multi-class recognition. A typical processing pipeline in this kind of system presents the following steps: segmentation, feature extraction, and classification. An under-exploited issue is the segmentation step, usually a windowing technique whose parameters are mostly heuristically defined. In this context, this work examines some segmentation parameters and how it affects the accuracy in the classification of hand gestures using a commercial sEMG wearable device. The main contribution of the work is to point out recommendations and insights about the segmentation step. Our findings show that for most feature sets and classifiers, there is no significant difference in using window lengths over 500 ms; however, for some cases, this value can be smaller without depreciating the accuracy. Moreover, there is no significant difference in using the truncated signal, which means using the first half of the signal in relation to the whole signal; no gesture is privileged in relation to the others due to the change in the segmentation parameters; data augmentation caused by changes on segmentation could affect the classification error; and the best performance was observed using TD9 feature set, SVMR classifier with an overlap fraction of 25%.
更多查看译文
关键词
Surface electromyography,Pattern recognition,Windowing parameters,Hand gesture,Upper-limb prosthesis
AI 理解论文
溯源树
样例
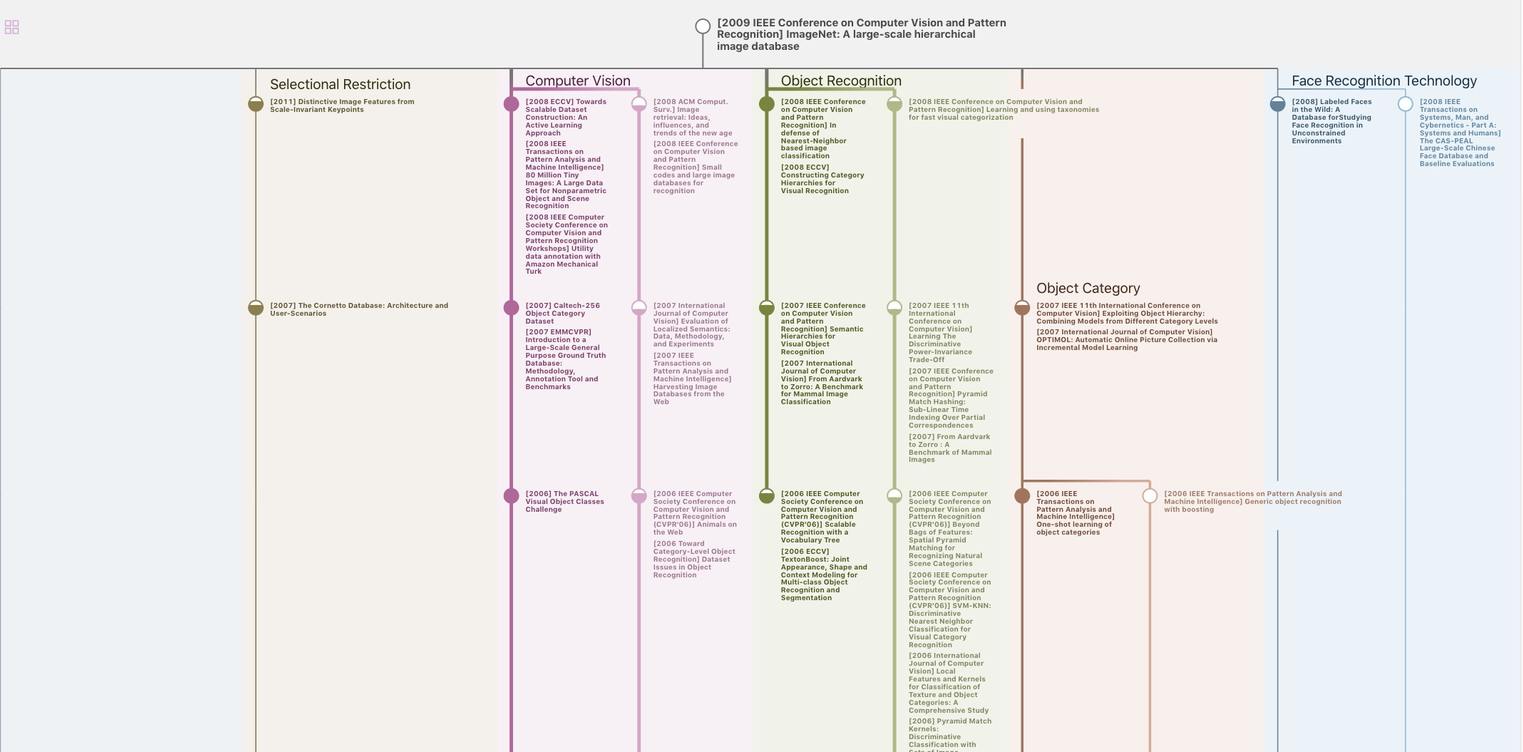
生成溯源树,研究论文发展脉络
Chat Paper
正在生成论文摘要