An improved cyclic multi model-eXtreme gradient boosting (CMM-XGBoost) forecasting algorithm on the GNSS vertical time series
Advances in Space Research(2023)
摘要
The study of GNSS vertical coordinate time series forecasting is helpful for monitoring the crustal plate movement, dam or bridge deformation monitoring, and global or regional coordinate system maintenance. The eXtreme Gradient Boosting (XGBoost) algorithm is a machine learning algorithm that can evaluate features, and it has a great potential and stability for long-span time series forecasting. This study proposes a multi-model combined forecasting method based on the XGBoost algorithm. The method constitutes a new time series as features through the fitting and forecasting results of the forecasting model. The XGBoost model is then used for forecasting. In addition, this method can obtain higher precision forecasting results through circulation. To verify the performance of the forecasting method, 1095 epochs of data in the Up coordinate of 16 GNSS stations are selected for the forecasting test. Compared with the CNN-LSTM model, the experimental results of our forecasting method show that the mean absolute error (MAE) values are reduced by 30.23 %∼52.50 % and the root mean square error (RMSE) values are reduced by 31.92 %∼54.33 %. The forecasting results have higher accuracy and are highly correlated to the original time series, which can better forecast the vertical movement of the GNSS stations. Therefore, the forecasting method can be applied to the up component of the GNSS coordinate time series.
更多查看译文
关键词
XGBoost,LSTM,GNSS,Time series,Forecasting
AI 理解论文
溯源树
样例
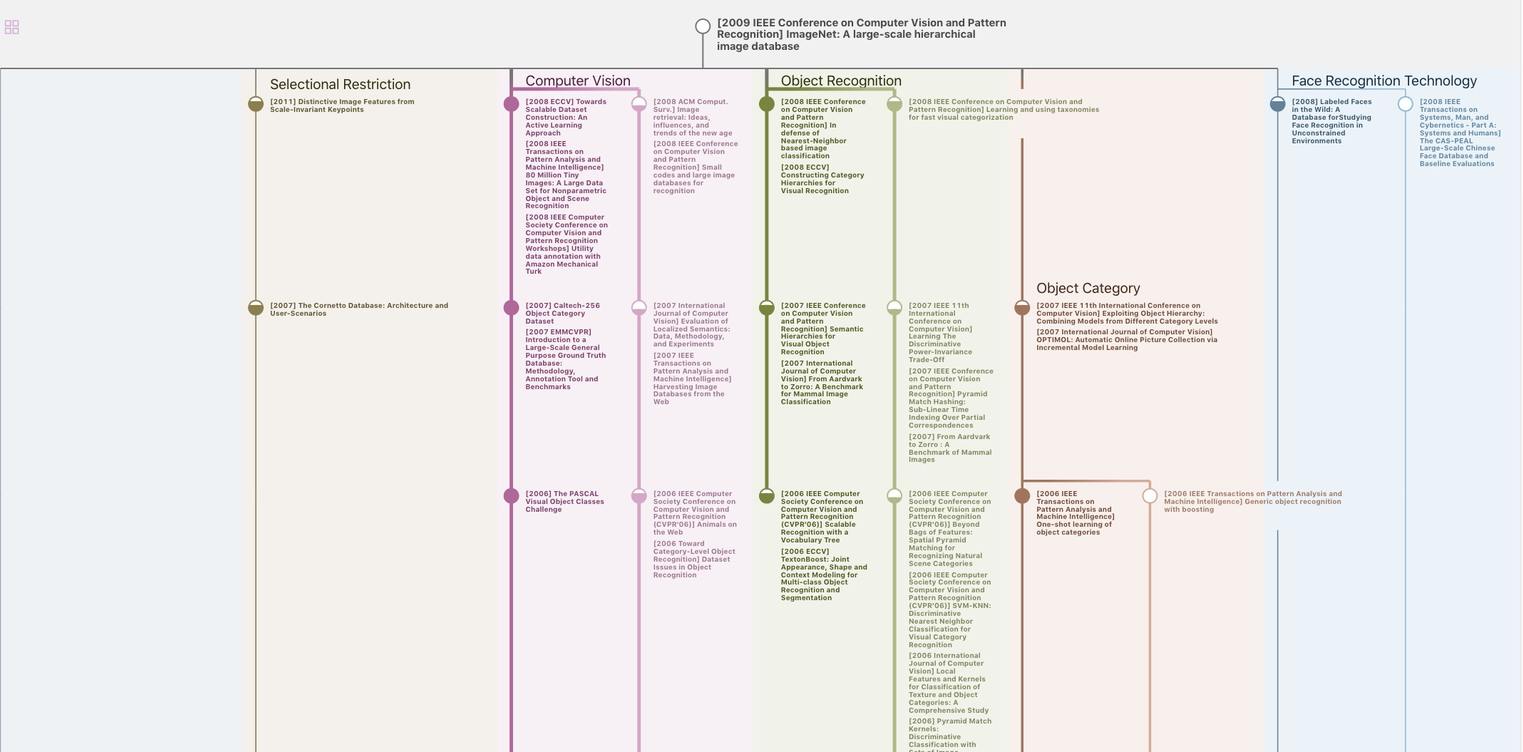
生成溯源树,研究论文发展脉络
Chat Paper
正在生成论文摘要