Machine learning versus human learning in predicting glass-forming ability of metallic glasses (vol 243, 118497, 2023)
ACTA MATERIALIA(2023)
摘要
Complex materials science problems such as glass formation must consider large system sizes that are many orders of magnitude too large to be solved by first-principles calculations. The successful application of machine learning (ML) in various other fields suggests that ML could be useful to address complex problems in materials science. To test its efficacy, we attempt to predict bulk metallic glass formation using ML. Surprisingly, we find that a recently developed ML model based on 201 alloy features constructed using simple combinations of 31 elemental features is indistinguishable from models that are based on unphysical features. The 201ML-model performs better than the unphysical model only when significant separation of training and testing data is achieved. However, it performs significantly worse than a human-learning based three-feature model. The limited performance of the 201ML-model originates from the inability to accurately represent alloy features through elemental features, showing that physical insights about mixing behavior are required to develop predictable ML models.
更多查看译文
关键词
Machine learning,Human learning,Materials design,Metallic glass,Glass-forming ability
AI 理解论文
溯源树
样例
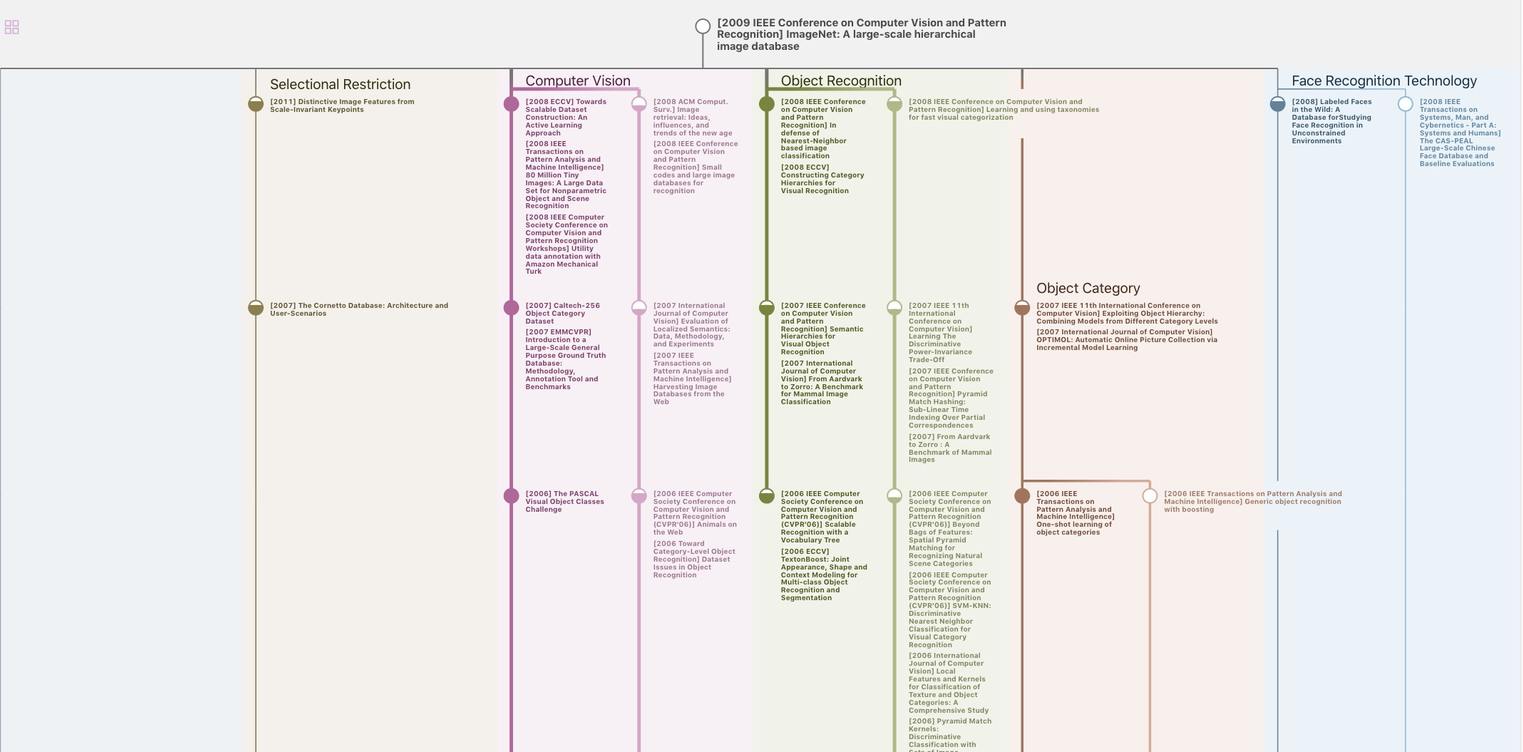
生成溯源树,研究论文发展脉络
Chat Paper
正在生成论文摘要