CoMBiNED: Multi-Constrained Model Based Planning for Navigation in Dynamic Environments
IEEE/RJS International Conference on Intelligent RObots and Systems (IROS)(2022)
摘要
Recent deep reinforcement learning (DRL) approaches have achieved high success rate in map-less dynamic obstacle avoidance tasks. However, navigation in unseen dynamic scenarios without a pre-built map in the presence of dynamic obstacles still remains an open challenge. Since, learning accurate models for complex robotic scenarios such as navigation directly from high dimensional sensory measurements requires a large amount of data and training. Furthermore, even a small change on robot configuration such as kino-dynamics or sensor in the inference time requires re-training of the policy. In this paper, we address these issues in a principled fashion through a multi-constraint model based online planning (CoMBiNED) framework that does not require any retraining or modifications on the existing policy. We disentangle the given task into sub-tasks and learn dynamical models for them. Treating these dynamical models as soft-constraints, we employ stochastic optimisation to jointly optimize these sub-tasks on-the-fly at the inference time. We consider navigation as central application in this work and evaluate our approach on publicly available benchmark with complex dynamic scenarios and achieved significant improvement over recent approaches both in the cases of with-and-without given map of the environment.
更多查看译文
关键词
navigation,planning,dynamic environments,multi-constrained
AI 理解论文
溯源树
样例
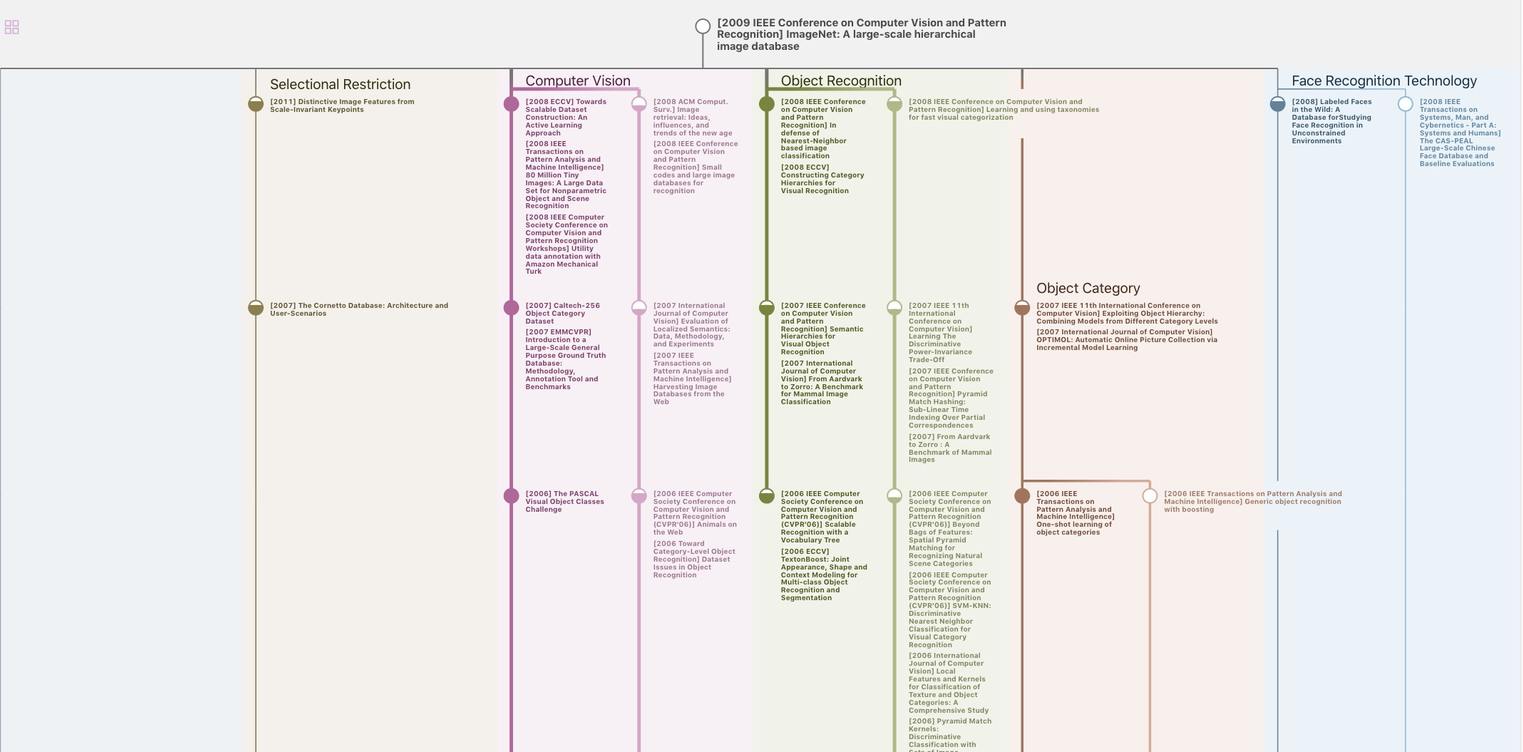
生成溯源树,研究论文发展脉络
Chat Paper
正在生成论文摘要