Semi-MAE: Masked Autoencoders for Semi-supervised Vision Transformers
arxiv(2023)
摘要
Vision Transformer (ViT) suffers from data scarcity in semi-supervised learning (SSL). To alleviate this issue, inspired by masked autoencoder (MAE), which is a data-efficient self-supervised learner, we propose Semi-MAE, a pure ViT-based SSL framework consisting of a parallel MAE branch to assist the visual representation learning and make the pseudo labels more accurate. The MAE branch is designed as an asymmetric architecture consisting of a lightweight decoder and a shared-weights encoder. We feed the weakly-augmented unlabeled data with a high masking ratio to the MAE branch and reconstruct the missing pixels. Semi-MAE achieves 75.9% top-1 accuracy on ImageNet with 10% labels, surpassing prior state-of-the-art in semi-supervised image classification. In addition, extensive experiments demonstrate that Semi-MAE can be readily used for other ViT models and masked image modeling methods.
更多查看译文
关键词
masked autoencoders,vision,semi-mae,semi-supervised
AI 理解论文
溯源树
样例
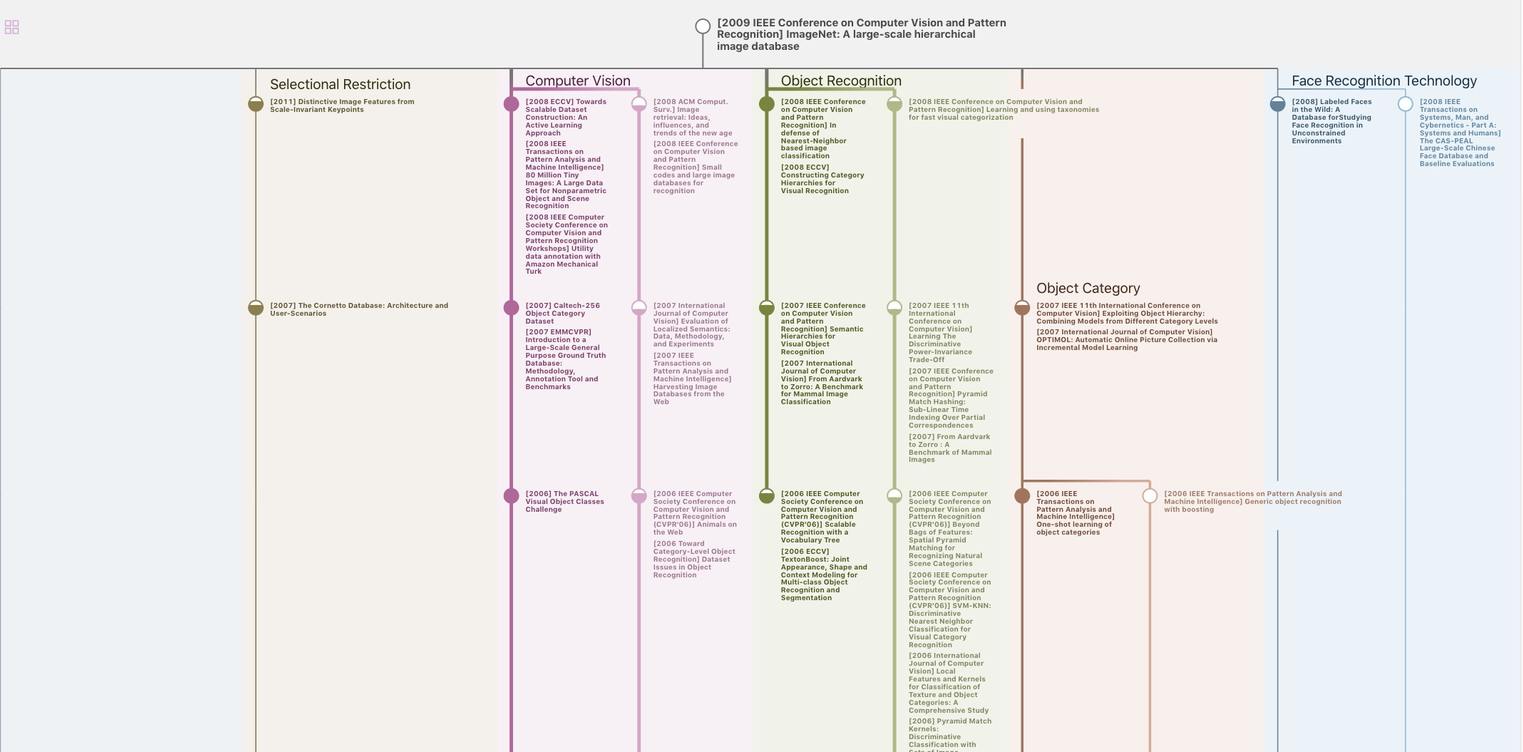
生成溯源树,研究论文发展脉络
Chat Paper
正在生成论文摘要