Fine-Grained Hard Negative Mining: Generalizing Mitosis Detection with a Fifth of the MIDOG 2022 Dataset
arxiv(2023)
摘要
Making histopathology image classifiers robust to a wide range of real-world variability is a challenging task. Here, we describe a candidate deep learning solution for the Mitosis Domain Generalization Challenge 2022 (MIDOG) to address the problem of generalization for mitosis detection in images of hematoxylin-eosin-stained histology slides under high variability (scanner, tissue type and species variability). Our approach consists in training a rotation-invariant deep learning model using aggressive data augmentation with a training set enriched with hard negative examples and automatically selected negative examples from the unlabeled part of the challenge dataset. To optimize the performance of our models, we investigated a hard negative mining regime search procedure that lead us to train our best model using a subset of image patches representing 19.6% of our training partition of the challenge dataset. Our candidate model ensemble achieved a F1-score of .697 on the final test set after automated evaluation on the challenge platform, achieving the third best overall score in the MIDOG 2022 Challenge.
更多查看译文
关键词
generalizing mitosis detection,mining,fine-grained,hard-negative
AI 理解论文
溯源树
样例
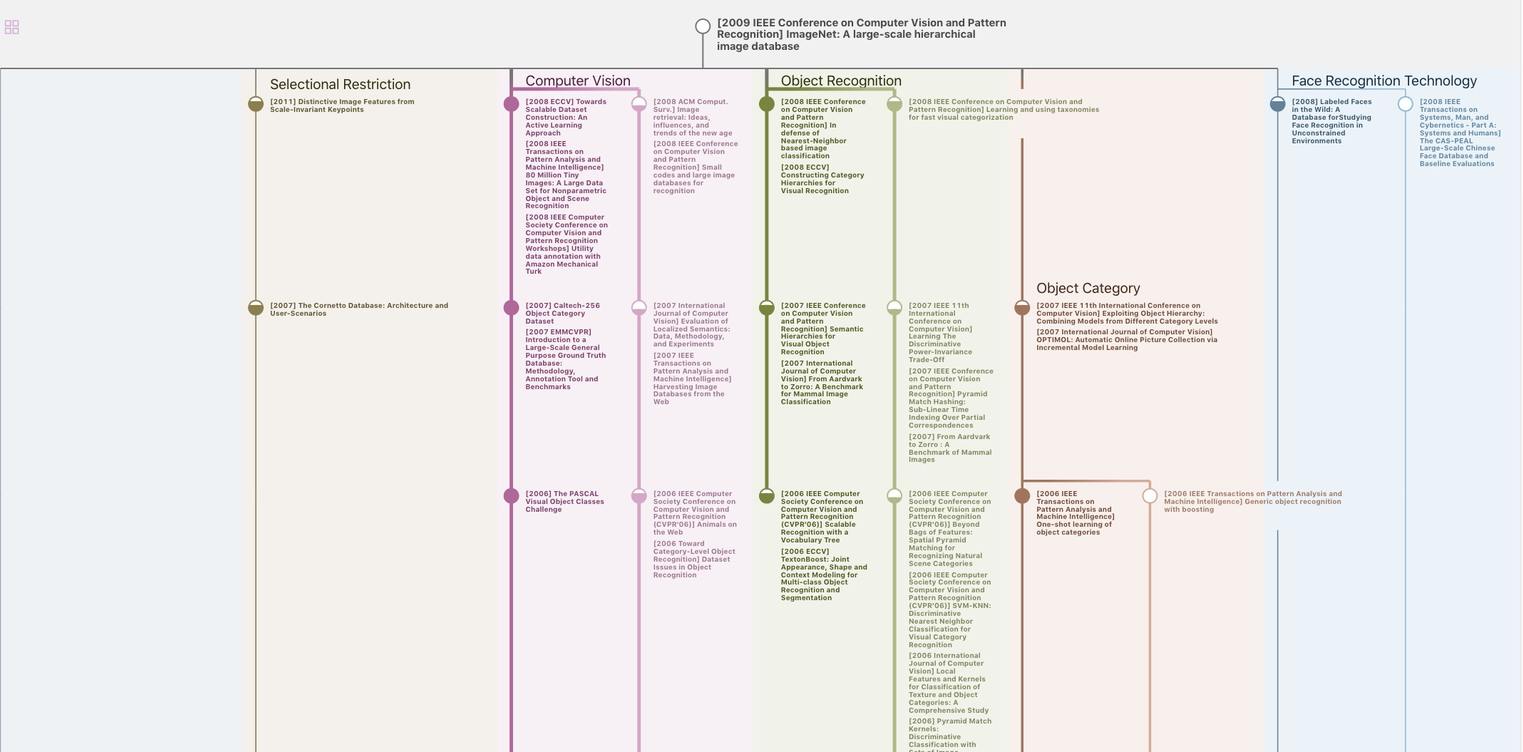
生成溯源树,研究论文发展脉络
Chat Paper
正在生成论文摘要