Data Augmentation and Classification of Sea-Land Clutter for Over-the-Horizon Radar Using AC-VAEGAN
arxiv(2023)
摘要
In the sea-land clutter classification of sky-wave over-the-horizon-radar (OTHR), the imbalanced and scarce data leads to a poor performance of the deep learning-based classification model. To solve this problem, this paper proposes an improved auxiliary classifier generative adversarial network~(AC-GAN) architecture, namely auxiliary classifier variational autoencoder generative adversarial network (AC-VAEGAN). AC-VAEGAN can synthesize higher quality sea-land clutter samples than AC-GAN and serve as an effective tool for data augmentation. Specifically, a 1-dimensional convolutional AC-VAEGAN architecture is designed to synthesize sea-land clutter samples. Additionally, an evaluation method combining both traditional evaluation of GAN domain and statistical evaluation of signal domain is proposed to evaluate the quality of synthetic samples. Using a dataset of OTHR sea-land clutter, both the quality of the synthetic samples and the performance of data augmentation of AC-VAEGAN are verified. Further, the effect of AC-VAEGAN as a data augmentation method on the classification performance of imbalanced and scarce sea-land clutter samples is validated. The experiment results show that the quality of samples synthesized by AC-VAEGAN is better than that of AC-GAN, and the data augmentation method with AC-VAEGAN is able to improve the classification performance in the case of imbalanced and scarce sea-land clutter samples.
更多查看译文
关键词
Clutter classification,data augmentation,deep learning,generative adversarial network (GAN),imbalanced and scarce samples,over-the-horizon-radar (OTHR)
AI 理解论文
溯源树
样例
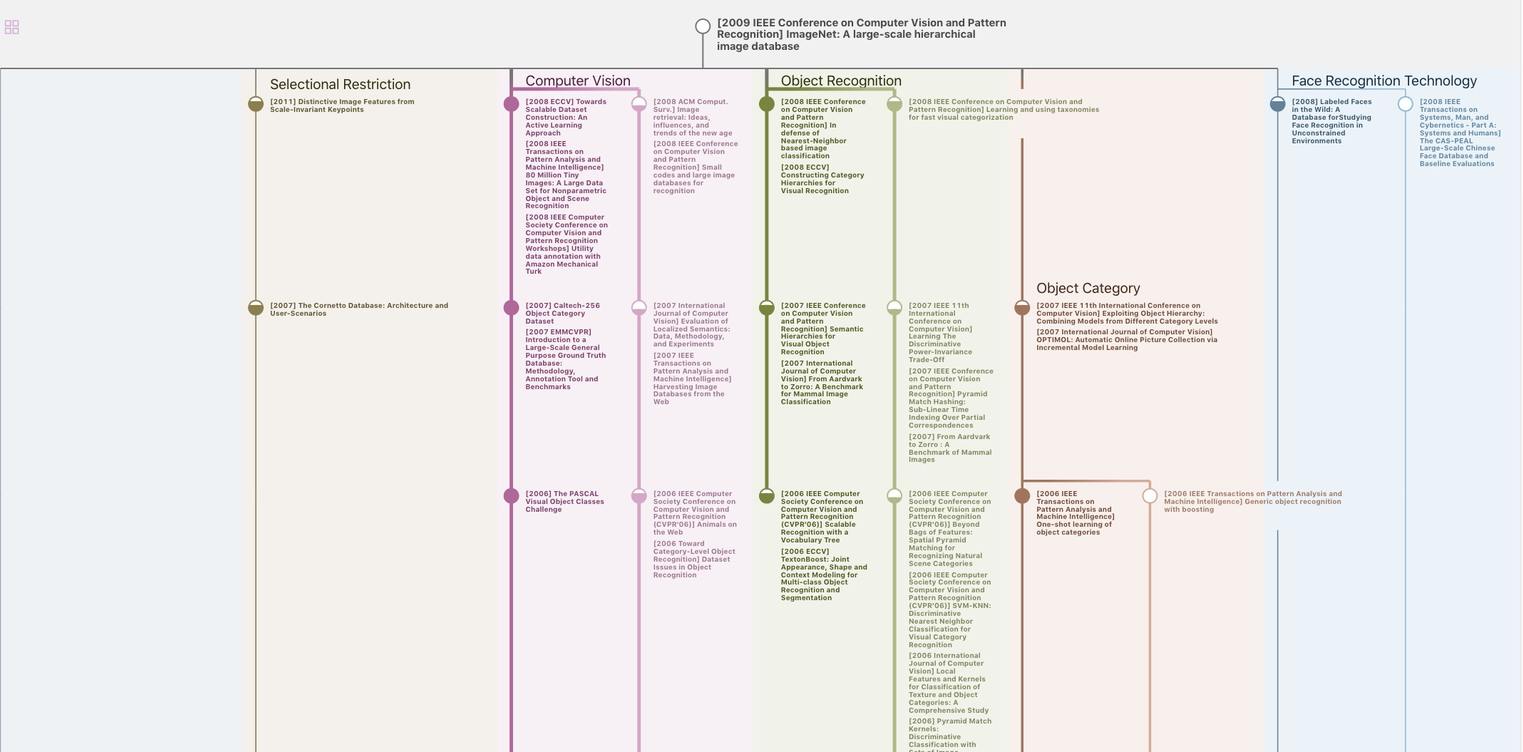
生成溯源树,研究论文发展脉络
Chat Paper
正在生成论文摘要