Can We Predict How Challenging Spoken Language Understanding Corpora Are Across Sources, Languages, and Domains?
International Workshop on Spoken Dialogue Systems Technology (IWSDS)(2021)
摘要
State-of-the art Spoken Language Understanding models of Spoken Dialog Systems achieve remarkable results on benchmark corpora thanks to the winning combination of pretraining on large collection of out-of-domain data with contextual Transformer representations and fine tuning on in-domain data. On average, performances are almost perfect on benchmark datasets such ATIS. However some phenomena can affect greatly these performance, like unseen events or ambiguities. They are the major sources of errors in real-life deployed systems although they are not necessarily equally represented in benchmark corpora. This paper aims to predict and characterize error-prone utterances and to explain what makes a given corpus more or less challenging. After training such a predictor on benchmark corpora from various languages and domains, we confront it to a new corpus collected from a French deployed vocal assistant with different distributional properties. We show that the predictor can highlight challenging utterances and explain the main complexity factors even though this corpus was collected in a completely different setting.
更多查看译文
关键词
language,languages
AI 理解论文
溯源树
样例
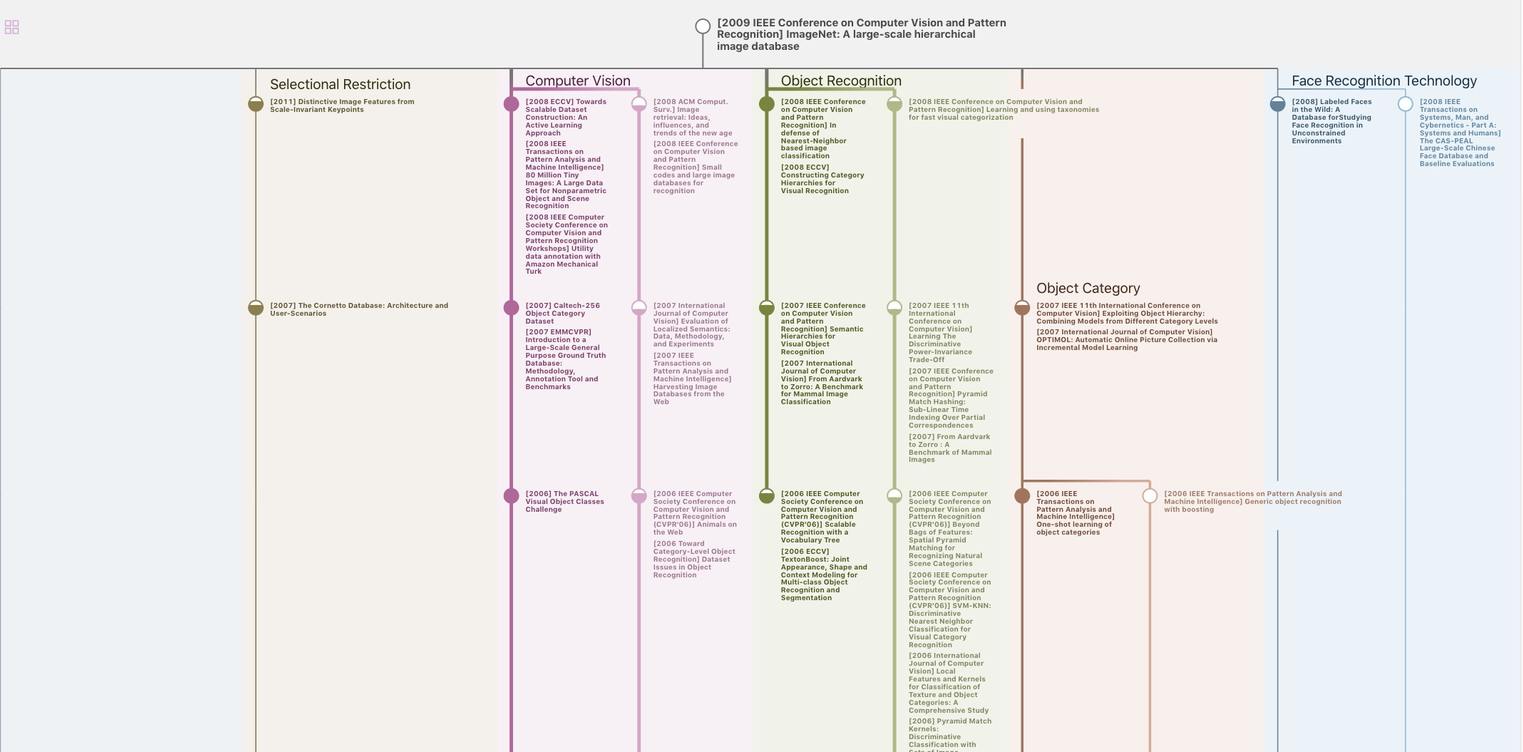
生成溯源树,研究论文发展脉络
Chat Paper
正在生成论文摘要