Efficient Cache Utilization via Model-aware Data Placement for Recommendation Models.
International Symposium on Memory Systems (MEMSYS)(2021)
摘要
Deep neural network (DNN) based recommendation models (RMs) represent a class of critical workloads that are broadly used in social media, entertainment content, and online businesses. Given their pervasive usage, understanding the memory subsystem behavior of these models is crucial, particularly from the perspective of future memory subsystem design. To this end, in this work, we first do an in-depth memory footprint and traffic analysis of emerging RMs. We observe that emerging RMs will severely stress future (and possibly larger) caches and memories. To address this challenge, we make the key observation that a data placement strategy that is aware of the components within these models (as opposed to one that considers the entire model as a whole) stands a better chance of relieving the stress on the memory subsystem. Specifically, of the two key components of these models, namely, embedding tables and multi-layer perceptron layers, we show how we can exploit the locality of memory accesses to embedding tables to come up with a more nuanced data placement scheme. We demonstrate how our proposed data placement strategy can reduce overall memory traffic (approximately 32%) while improving performance (up to 1.99 ×). We argue that memory subsystems that are more amenable to residency controls stand a better chance to address the needs of emerging models.
更多查看译文
AI 理解论文
溯源树
样例
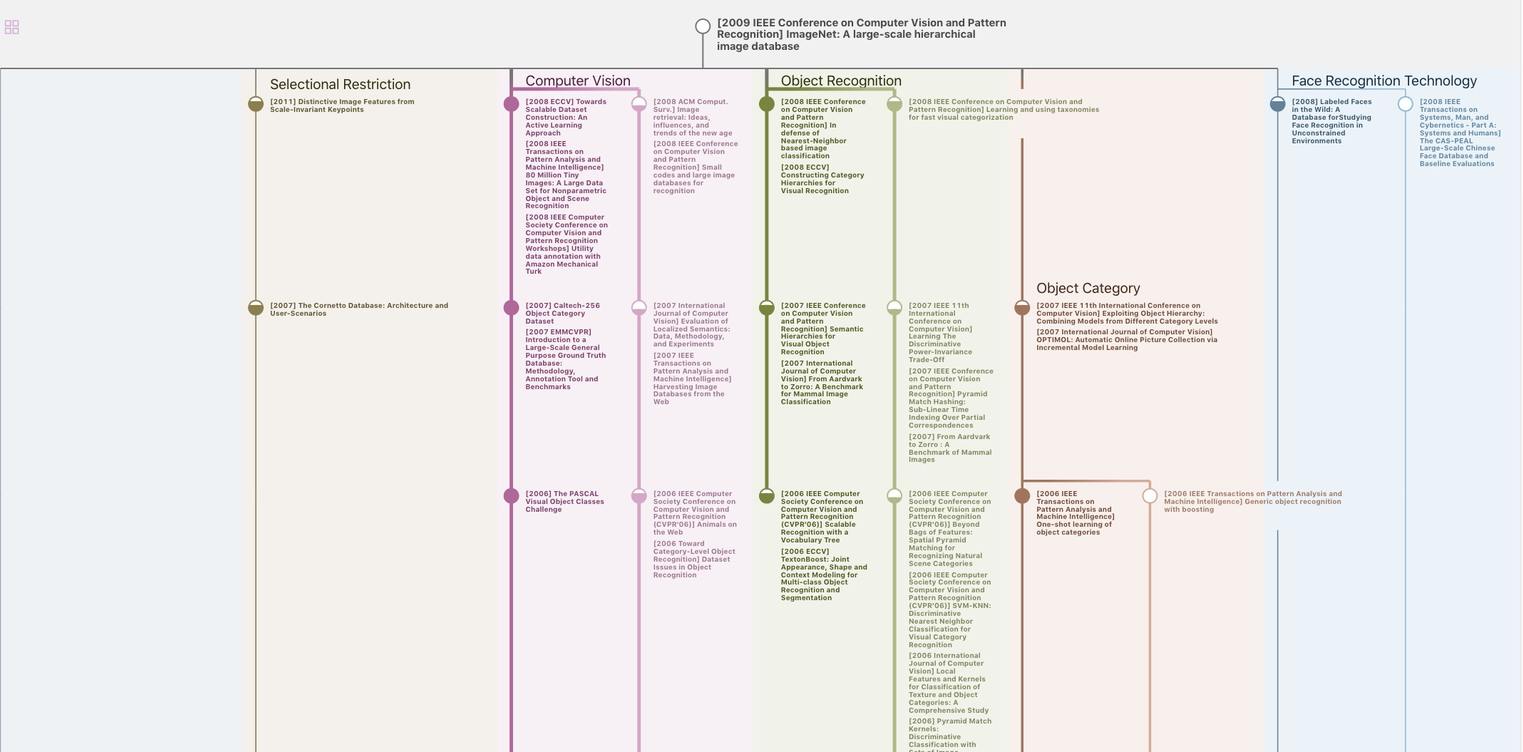
生成溯源树,研究论文发展脉络
Chat Paper
正在生成论文摘要