On Large-Batch Training of Residual Networks with SignSGD.
International Conference on Advances in Artificial Intelligence (ICAAI)(2021)
摘要
Large-batch training of deep neural networks (DNN) has recently been widely studied, since traversing the optimization landscape is faster with large batches and the emergence of parallel computing has made large-batch training feasible. However, its generalization is still worse compared to small-batch training, which is commonly attributed to the low gradient variance with large batches. We show that sign stochastic gradient descent (signSGD) is a suitable candidate for large-batch ResNet training with improved generalization compared to vanilla SGD. We further modify signSGD to improve the convergence for large batches while retaining its generalization properties. In particular, we propose r-signSGD, which restricts certain parameter updates of signSGD—r-signSGD updates a parameter of the neural network only if its gradient sign matches its gradient momentum sign. This prevents unnecessary/wrong updates given by signSGD especially for parameters with small gradients. We show that r-signSGD improves the convergence of signSGD while also performing better than signSGD with momentum, vanilla SGD, and even SGD with momentum in terms of test accuracy in the CIFAR10 image classification task.
更多查看译文
AI 理解论文
溯源树
样例
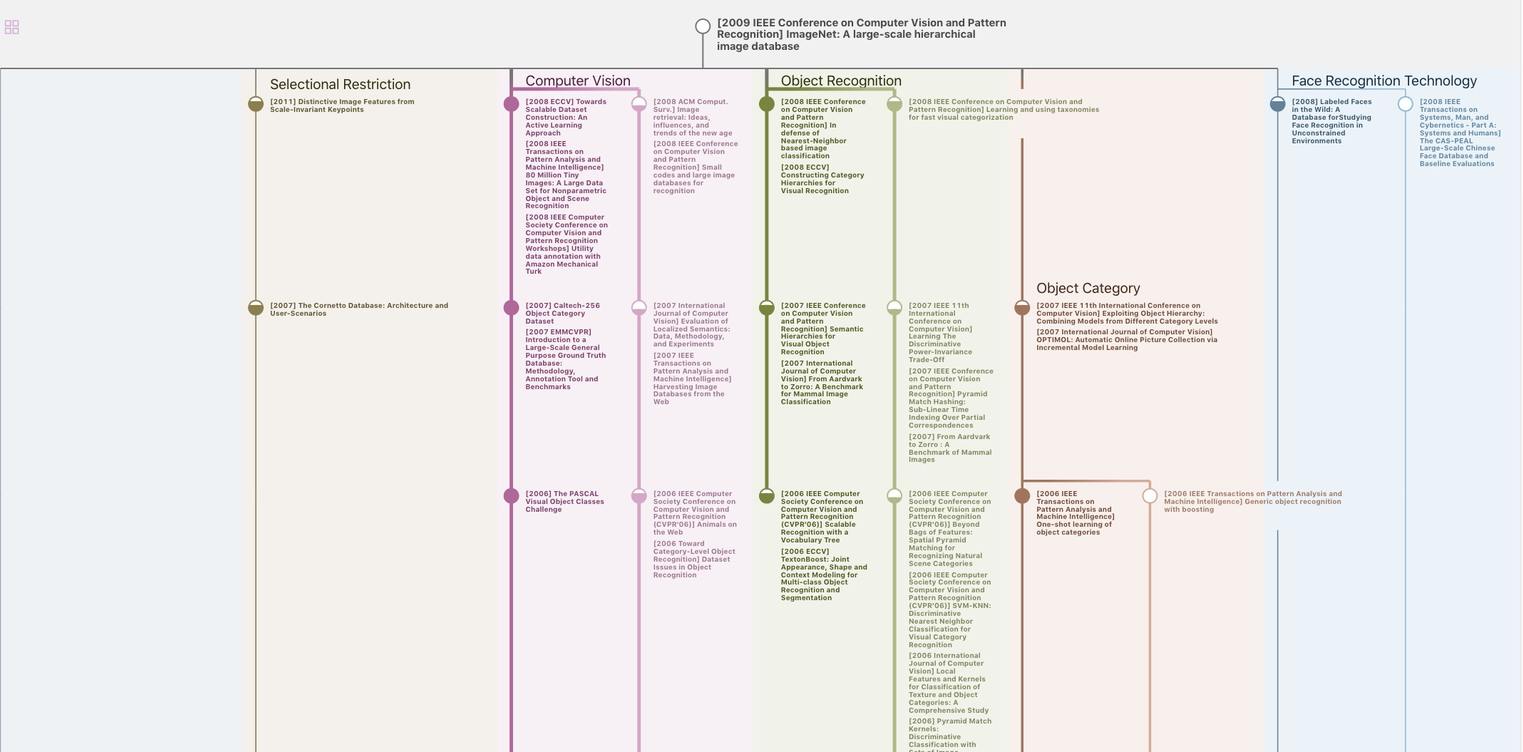
生成溯源树,研究论文发展脉络
Chat Paper
正在生成论文摘要