Privacy-Preserving Distributed Support Vector Machines.
International Workshop on Data Management and Analytics for Medicine and Healthcare (DMAH)(2021)
摘要
Federated machine learning is a promising paradigm allowing organizations to collaborate toward the training of a joint model without the need to explicitly share sensitive or business-critical datasets. Previous works demonstrated that such paradigm is not sufficient to preserve confidentiality of the training data, even to honest participants. In this work, we extend a well-known framework for training sparse Support Vector Machines in a distributed setting, while preserving data confidentiality by means of a novel non-interactive secure multiparty computation engine, that preserves data confidentiality. We formally demonstrate the security properties of the engine and provide, by means of extensive empirical evaluation, the performance of the extended framework both in terms of accuracy and execution time.
更多查看译文
关键词
Distributed support vector machines, Privacy-preserving machine learning, Secure federated learning
AI 理解论文
溯源树
样例
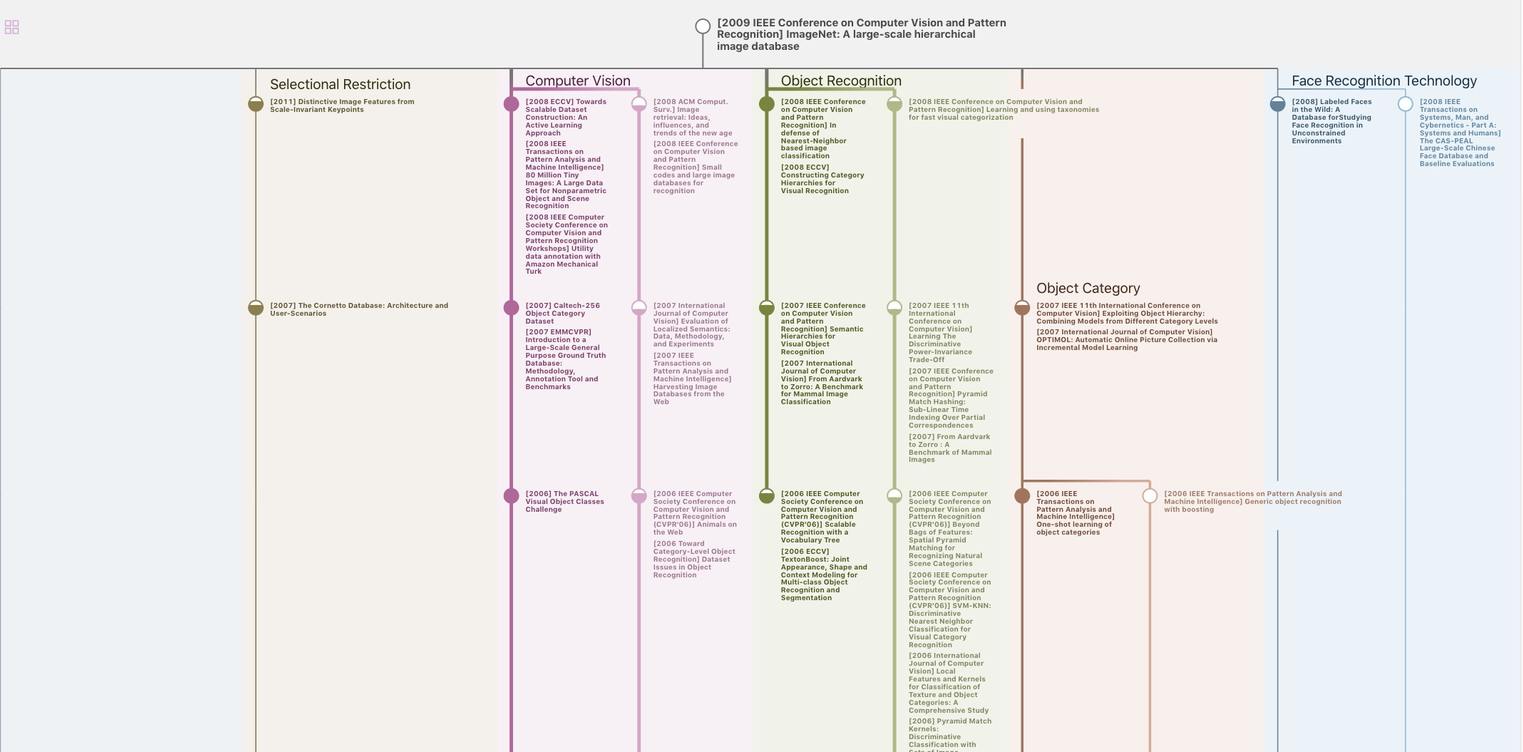
生成溯源树,研究论文发展脉络
Chat Paper
正在生成论文摘要