Head and Neck Tumor Segmentation with Deeply-Supervised 3D UNet and Progression-Free Survival Prediction with Linear Model.
International Conference on Medical Image Computing and Computer-Assisted Intervention (MICCAI)(2021)
摘要
Accurate segmentation of Head and Neck (H&N) tumor has important clinical relevance in disease characterization, thereby holding a strong potential for better cancer treatment planning and optimized patient care. In recent times, the development in deep learning-based models has been able to effectively and accurately perform medical images segmentation task that eliminates the problems associated with manual annotation of region of interest (ROI) such as significant human efforts and inter-observer variability. For H&N tumors, FDG-PET and CT carry complementary information of metabolic and structural details of tumor and the fusion of those modalities were explored in this study, which led to significant enhancement in performance level with combined data. Furthermore, deep supervision technique was applied to the segmentation network, where the computation of loss occurs at multiple layers that allows for gradients to be injected deeper into the network and facilitates the training. Our proposed segmentation methods yield promising result, with a Dice Similarity Coefficient (DSC) of 0.731 in our cross-validation experiment. Finally, we developed a linear model for progression-free survival prediction using extracted imaging and non-imaging features.
更多查看译文
关键词
neck tumor segmentation,deeply-supervised,progression-free
AI 理解论文
溯源树
样例
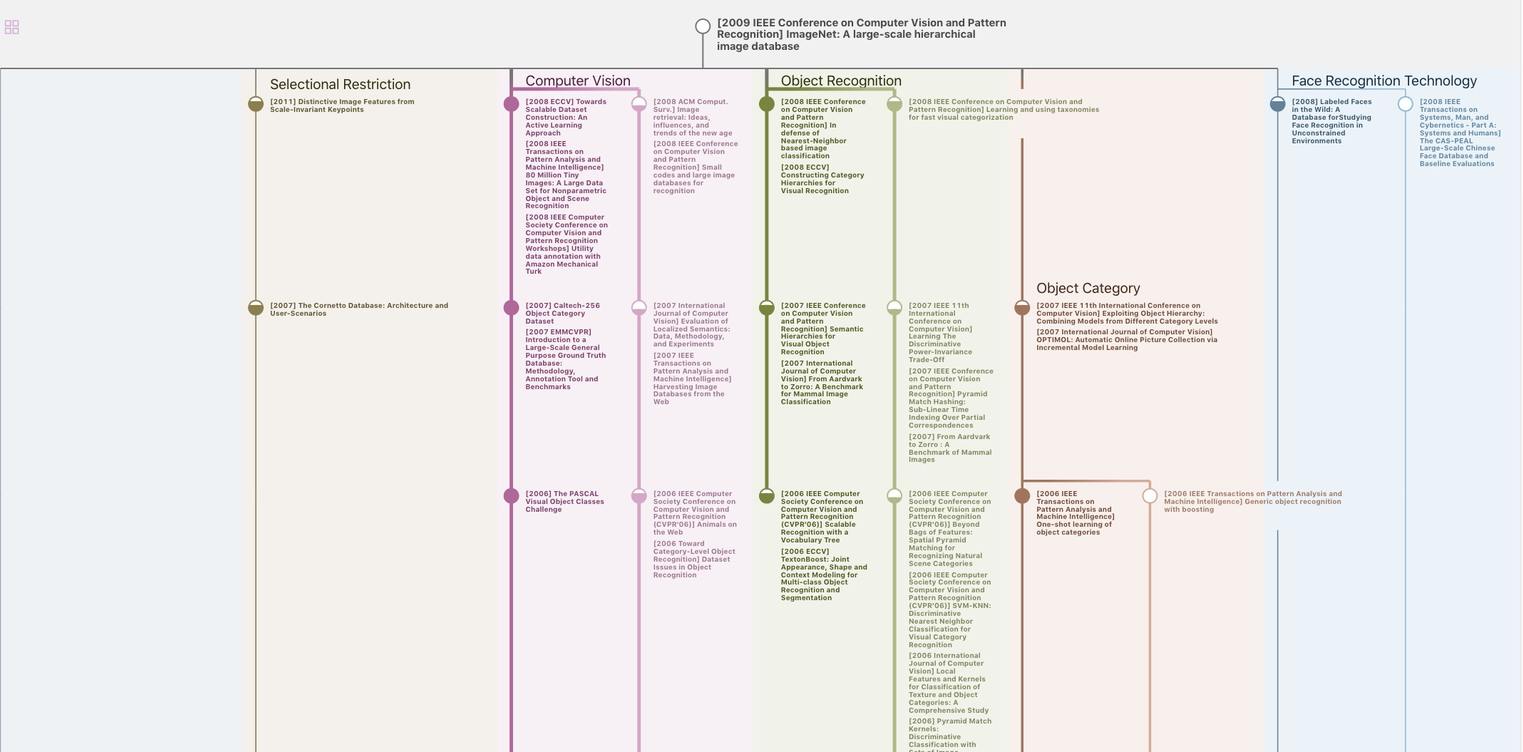
生成溯源树,研究论文发展脉络
Chat Paper
正在生成论文摘要