ADASemSeg: An Active Learning Based Data Adaptation Strategy for Improving Cross Dataset Breast Tumor Segmentation
Machine Learning, Image Processing, Network Security and Data Sciences(2023)
摘要
Highly efficient breast ultrasound (BUS) segmentation models trained and tested on samples from one dataset do not usually have a high performance when inference is done on samples from other datasets. The solution to this data adaptation problem is crucial when it comes to deploying a trained model in a new diagnostic center. In this work, a novel active learning-based strategy ADASemSeg is proposed for dealing with this problem, where a model adapts itself from a source dataset to a target dataset via active interactions with an Expert/Oracle. Experimental analysis on two publicly available datasets suggests that the proposed approach can achieve (12–25)% improvements over baselines. Moreover, ADASemSeg can ensure a quick adaptation to the target dataset while requiring a very small amount of Oracle feedback. With such a generic approach, any segmentation model can be effectively adapted to new data distributions, improving cross-center BUS tumor segmentation performances.
更多查看译文
关键词
Breast cancer, Breast ultrasound, Semantic segmentation, Active learning, Data adaptation
AI 理解论文
溯源树
样例
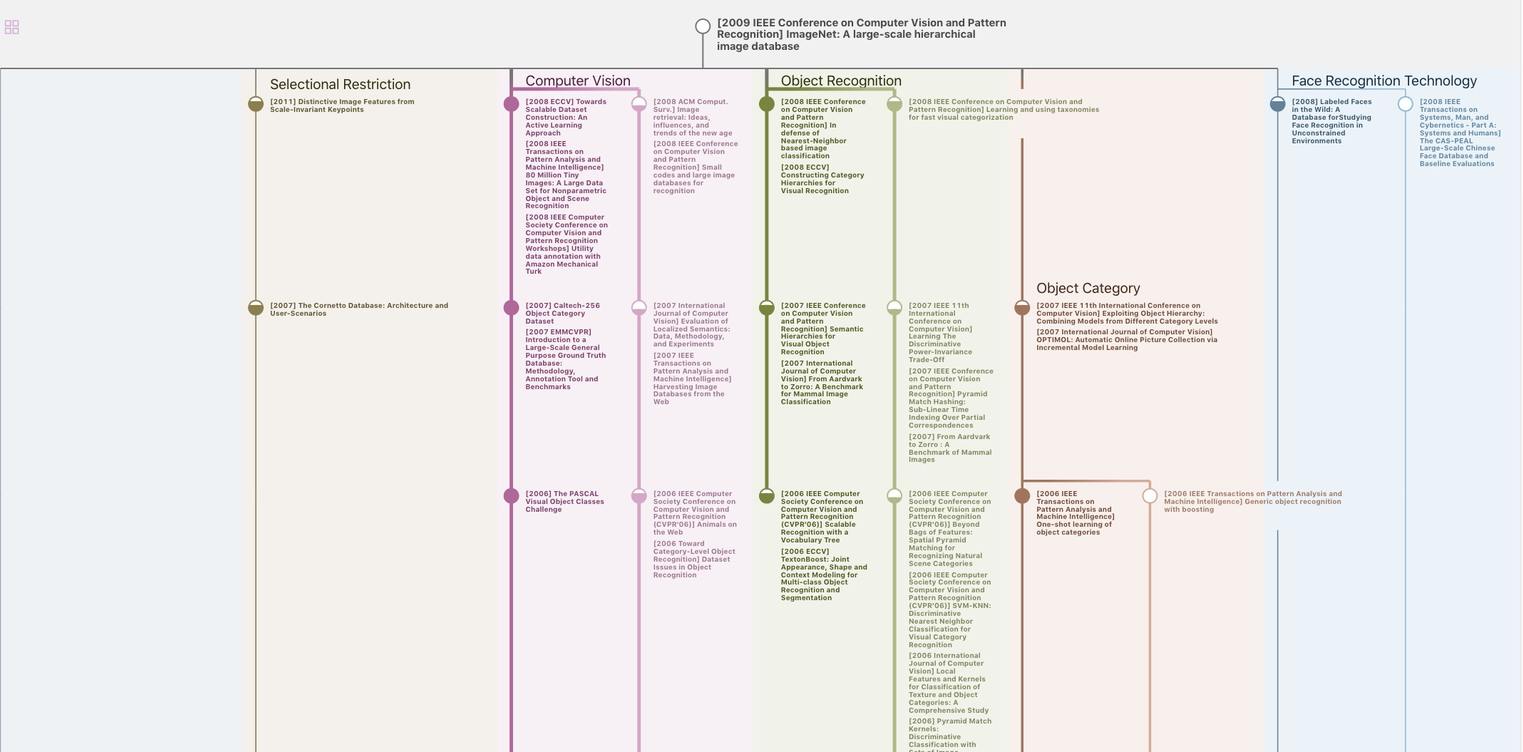
生成溯源树,研究论文发展脉络
Chat Paper
正在生成论文摘要