AMLP-Conv, a 3D Axial Long-range Interaction Multilayer Perceptron for CNNs
Machine Learning in Medical Imaging(2022)
摘要
While Convolutional neural networks (CNN) have been the backbone of medical image analysis for years, their limited long-range interaction restrains their ability to encode long distance anatomical relationships. On the other hand, the current approach to capture long distance relationships, Transformers, is constrained by their quadratic scaling and their data inefficiency (arising from their lack of inductive biases). In this paper, we introduce the 3D Axial Multilayer Perceptron (AMLP), a long-range interaction module whose complexity scales linearly with spatial dimensions. This module is merged with CNNs to form the AMLP-Conv module, a long-range augmented convolution with strong inductive biases. Once combined with U-Net, our AMLP-Conv module leads to significant improvement, outperforming most transformer based U-Nets on the ACDC dataset, and reaching a new state-of-the-art result on the Multi-Modal Whole Heart Segmentation (MM-WHS) dataset with an almost 1.1% Dice score improvement over the previous scores on the Computed Tomography (CT) modality.
更多查看译文
关键词
MLP, Axial attention, Convolutional neural network, Multi-label, 3D semantic segmentation, Heart segmentation
AI 理解论文
溯源树
样例
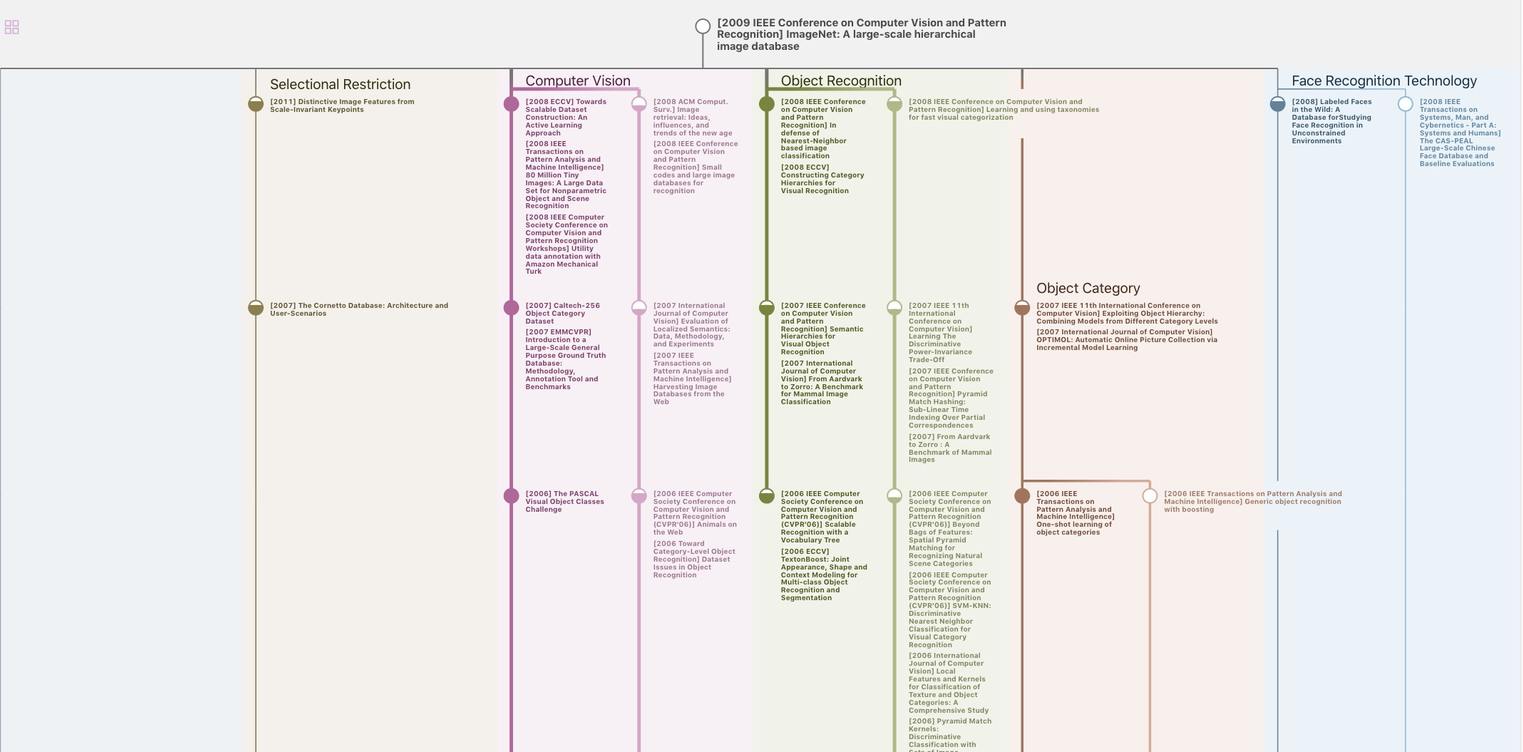
生成溯源树,研究论文发展脉络
Chat Paper
正在生成论文摘要