Multivariate Functional Approximation of Scientific Data
In Situ Visualization for Computational Science(2022)
摘要
While our computational capability to generate raw data grows, the ability to store, transform, and draw conclusions from scientific data is lagging. Beginning with the introductory chapter and continuing throughout much of this book, we see numerous examples of how in situ processing can help close the gap between data generation and data analysis. This chapter expands the discussion of in situ methods beyond when and where data are processed, to how data are represented. Rethinking the way that scientific data are represented can empower subsequent visualization and analysis, especially when such data transformations are performed in situ. Scientific data may be transformed by recasting to a data model fundamentally different from the discrete pointwise or element-wise datasets produced by computational models. In Multivariate Functional Approximation, or MFA, scientific datasets are redefined in a hypervolume of piecewise-continuous basis functions. Compared with existing discrete models, the continuous functional model can save space while affording many of the same spatiotemporal analyses without reverting back to the discrete form. In this chapter, modeling the MFA, in situ, is presented. The data model and modeling approach are parallelized for high-performance computing. A lightweight and efficient method of enforcing high-degree continuity across subdomains in the parallel decomposition is also included. The MFA can subsequently be used post hoc to evaluate points and derivatives anywhere in the domain, facilitating numerous analysis and visualization applications.
更多查看译文
AI 理解论文
溯源树
样例
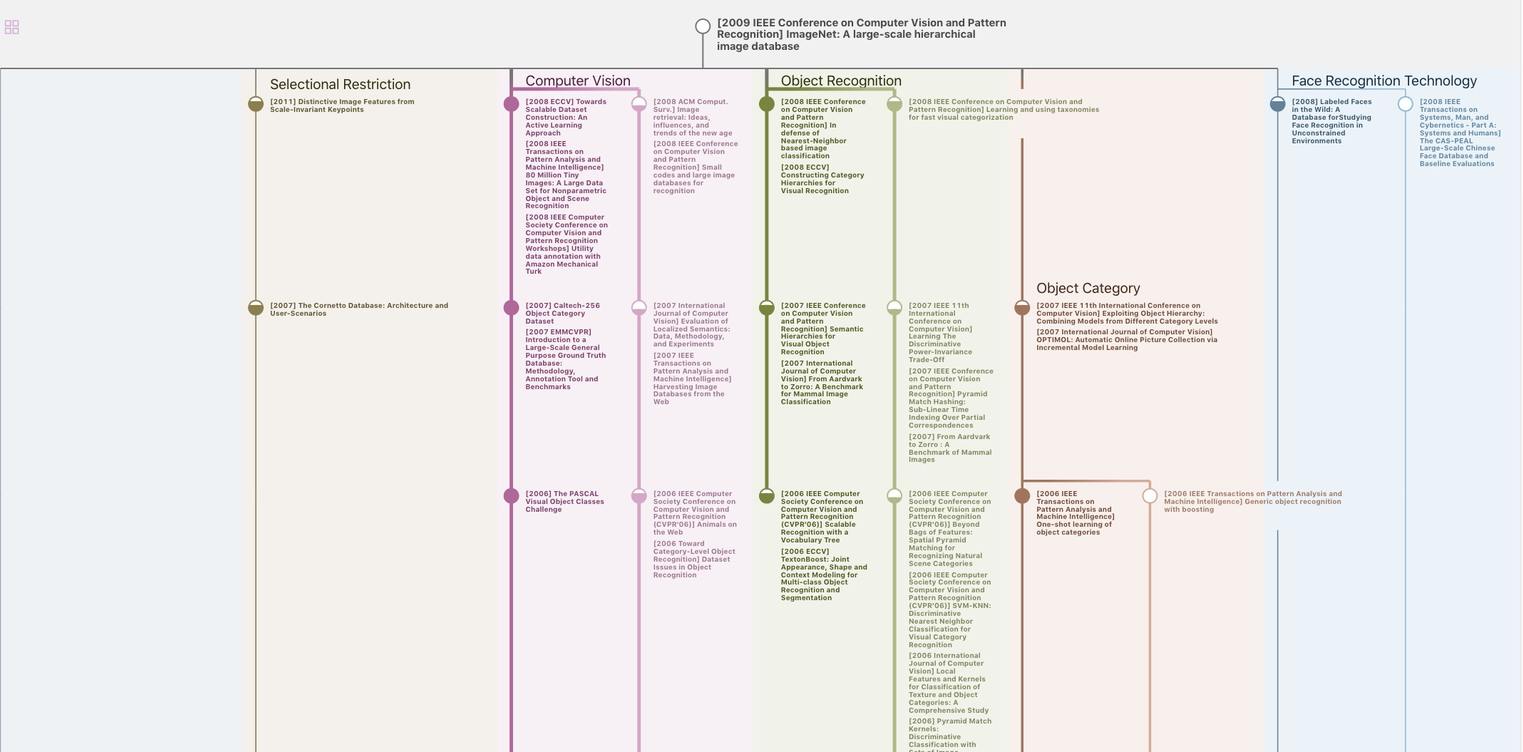
生成溯源树,研究论文发展脉络
Chat Paper
正在生成论文摘要