NIDN: Medical Code Assignment via Note-Code Interaction Denoising Network
Bioinformatics Research and Applications(2023)
摘要
Clinical records are files that contain detailed information about a patient's health status. Clinical notes are typically complex, and the medical code space is large, so medical code assignment from clinical text is a long-standing challenge. The traditional manual coding method is inefficient and error-prone. Incorrect coding may lead to adverse consequences. With machine learning and computer hardware development, the deep neural network model has been widely applied in the medical care domain. However, noise in lengthy documents, complex code association, and the imbalanced class problem urgently need to be solved. Therefore, we propose a Note-code Interaction Denoising Network (NIDN). We exploit the self-attention mechanism to identify the most relevant context of the medical code in the clinical document. We leverage the label attention mechanism to learn code-specific text representation. We utilize the correlation between labels in multi-task learning to assist the model in prediction. To better learn from lengthy texts and improve the performance of long-tail distribution, we develop a denoising module to reduce the influence of noise in medical code prediction. Experimental results show that our proposed model outperforms competitive baselines on a real-world MIMIC-III dataset.
更多查看译文
关键词
Medical Code Assignment, Attention Mechanism, Denoising Mechanism
AI 理解论文
溯源树
样例
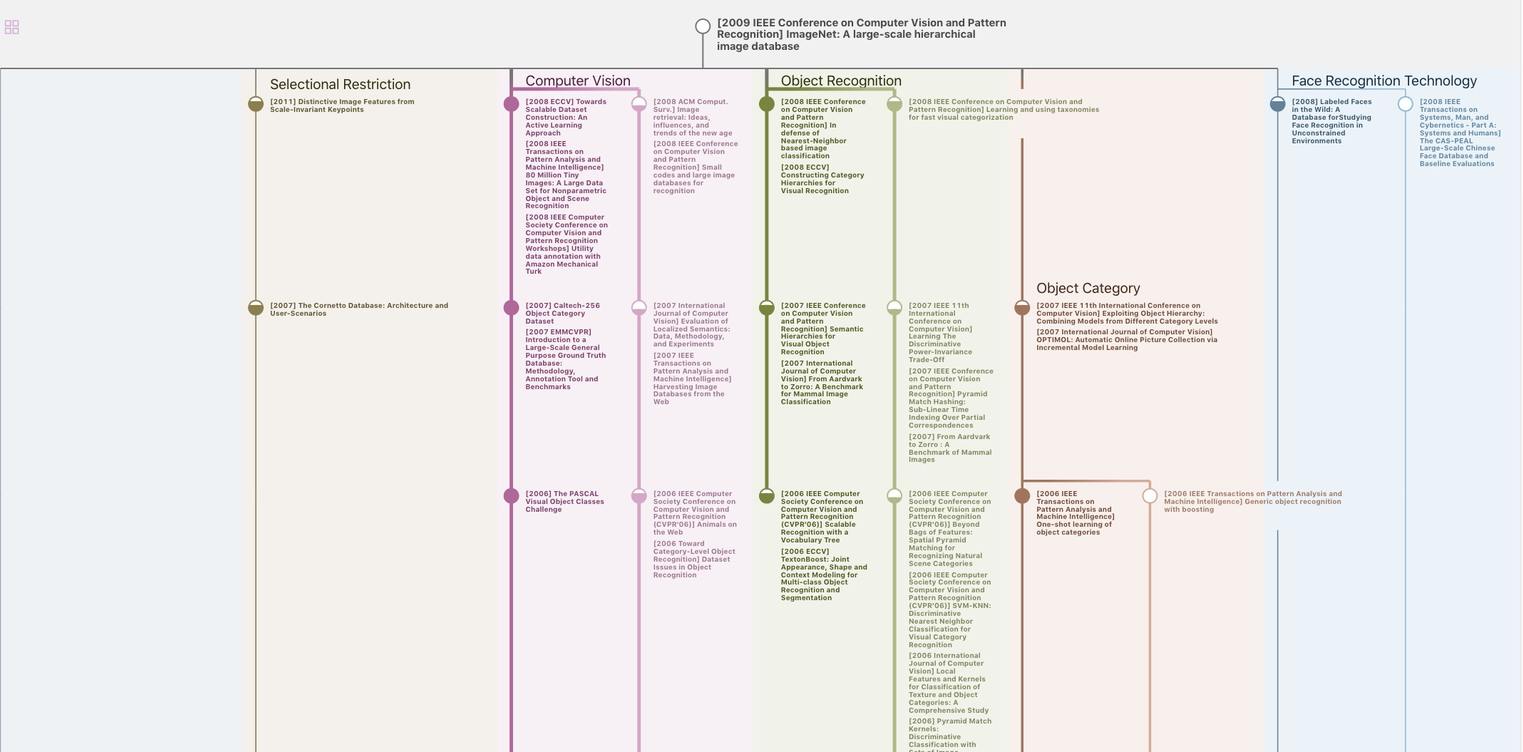
生成溯源树,研究论文发展脉络
Chat Paper
正在生成论文摘要