Predicting Hourly Street-Scale NO2 and PM2.5 Concentrations Using Machine Learning at One of the Danish Traffic Hotspots
Air Pollution Modeling and its Application XXVIII(2023)
摘要
Road transport is one of the significant sources of ambient air pollution. Machine learning approaches are becoming increasingly popular to predict and forecast air quality. In conjunction, this study aims to explore the potential of commonly used machine learning algorithms, Artificial Neural Networks (ANN), Random Forests (RF), and Support Vector Machines (SVM) to accurately predict hourly, street-scale NO2 (ppb) and particulate matter (PM2.5) (µg/m3) at one of the Danish traffic hotspots, H. C. Andersens Boulevard (HCAB), in Copenhagen (2013–2015). Results suggest that the RF, overall, outperforms others [RF: RMSE = 11.2–13.5, R2 = 0.73–0.81; ANN: RMSE = 13.5–14.1, R2 = 0.69–0.75; SVM: RMSE = 14.7–15.1, R2 = 0.64–0.65]. One strength of this study is that it addresses the research gaps concerning the unexplored potential of machine learning techniques for air quality prediction in Denmark. Future work will include more predictor variables (e.g. traffic attributes) and testing of other techniques (e.g. Extreme Gradient Boosting) (XGBoost).
更多查看译文
关键词
Road traffic, Air pollution, Machine learning, Prediction exposure
AI 理解论文
溯源树
样例
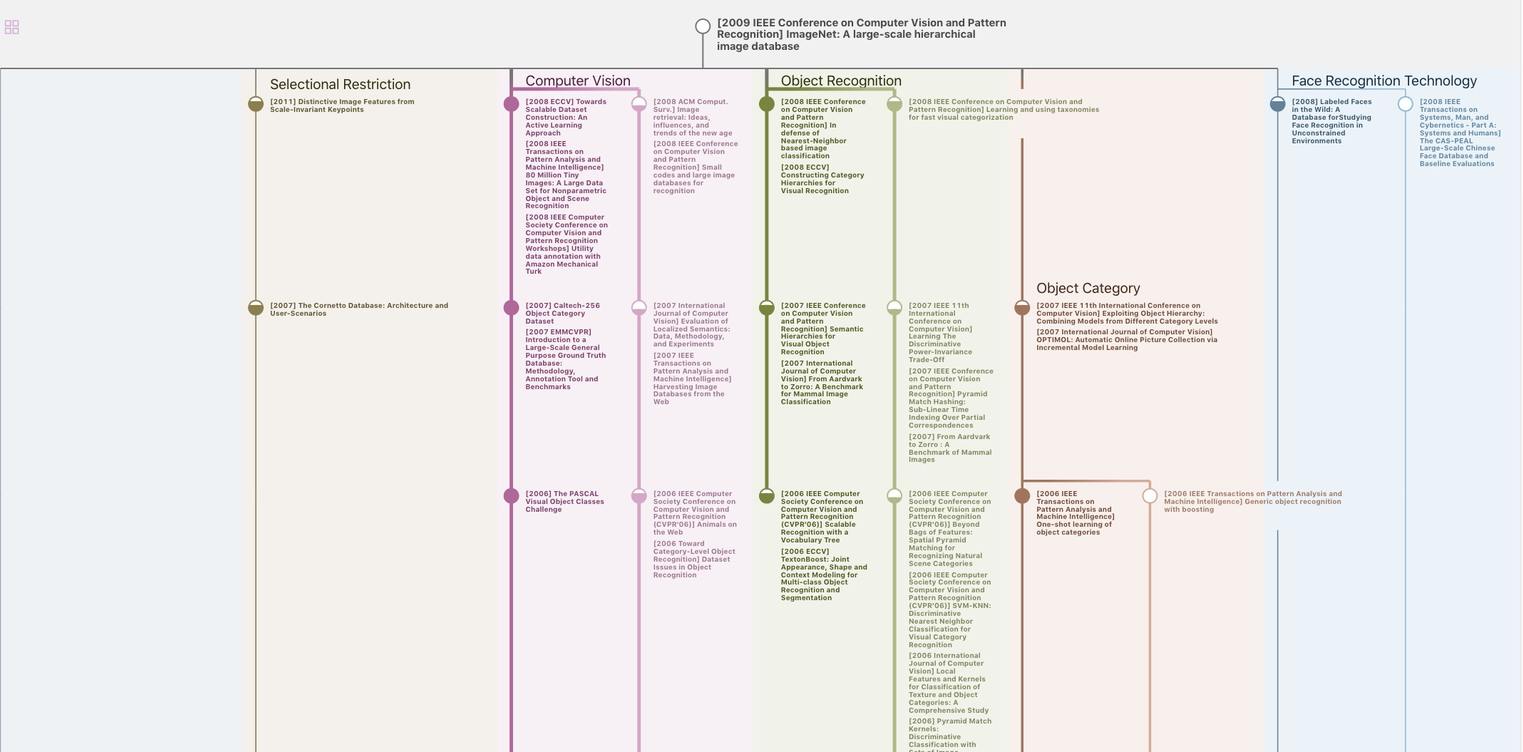
生成溯源树,研究论文发展脉络
Chat Paper
正在生成论文摘要