Exploring Expressed Emotions for Neural News Recommendation.
User Modeling, Adaptation, and Personalization (UMAP)(2022)
摘要
Due to domain-specific challenges such as short item lifetimes and continuous cold-start issues, news recommender systems rely more on content-based methods to deduce reliable user models and make personalized recommendations. Research has shown that alongside the content of an item, the way it is presented to the users also plays a critical role. In this work, we focus on the effect of incorporating expressed emotions within news articles on recommendation performance. We propose a neural news recommendation model that disentangles semantic and emotional modeling of news articles and users. While we exploit the textual content for the semantic representation, we extract and combine emotions of different information levels for the emotional representation. Offline experiments on a real-world dataset show that our approach outperforms non-emotion-aware solutions significantly. Finally, we provide a future outline, where we plan to investigate a) the online performance and b) the explainability/explorability of our approach.
更多查看译文
AI 理解论文
溯源树
样例
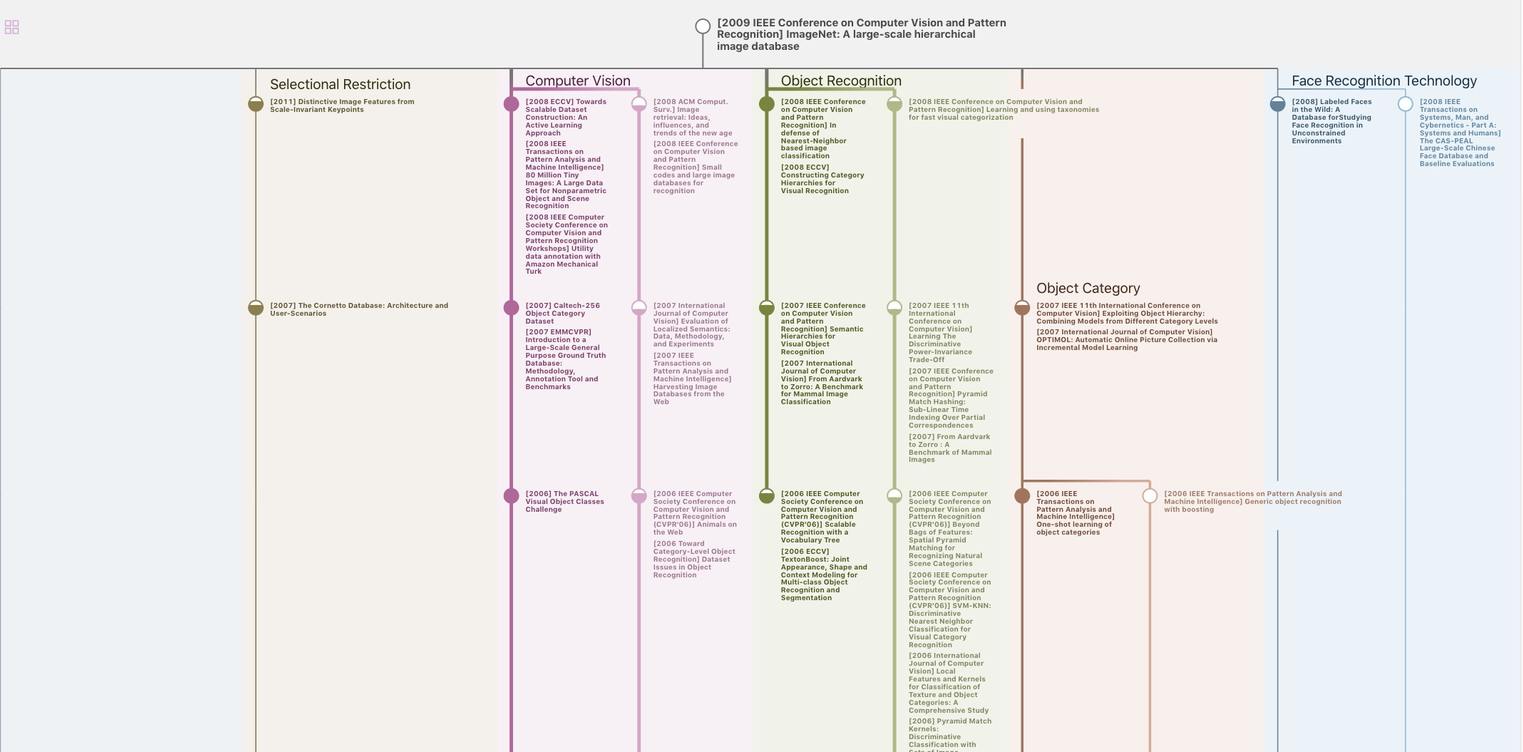
生成溯源树,研究论文发展脉络
Chat Paper
正在生成论文摘要