Maximum Likelihood Estimators on MCMC Sampling Algorithms for Decision Making.
Professional Practice in Artificial Intelligence (PPAI)(2022)
摘要
Monte Carlo simulations using Markov chains as the Gibbs sampler and Metropolis algorithm are widely used techniques for modelling stochastic problems for decision making. Like all other Monte Carlo approaches, MCMC exploits the law of large numbers via repeated random sampling. Samples are formed by running a Markov Chain that is constructed in such a way that its stationary distribution closely matches the input function, which is represented by a proposal distribution. In this paper, the fundamentals of MCMC methods are discussed, including the algorithm selection process, optimizations, as well as some efficient approaches for utilizing generalized linear mixed models. Another aim of this paper is to highlight the usage of the EM method to get accurate maximum likelihood estimates in the context of generalized linear mixed models.
更多查看译文
关键词
MCMC methods, Gibbs sampler, Maximum likelihood, Estimators, Generalized linear mixed models, Decision making
AI 理解论文
溯源树
样例
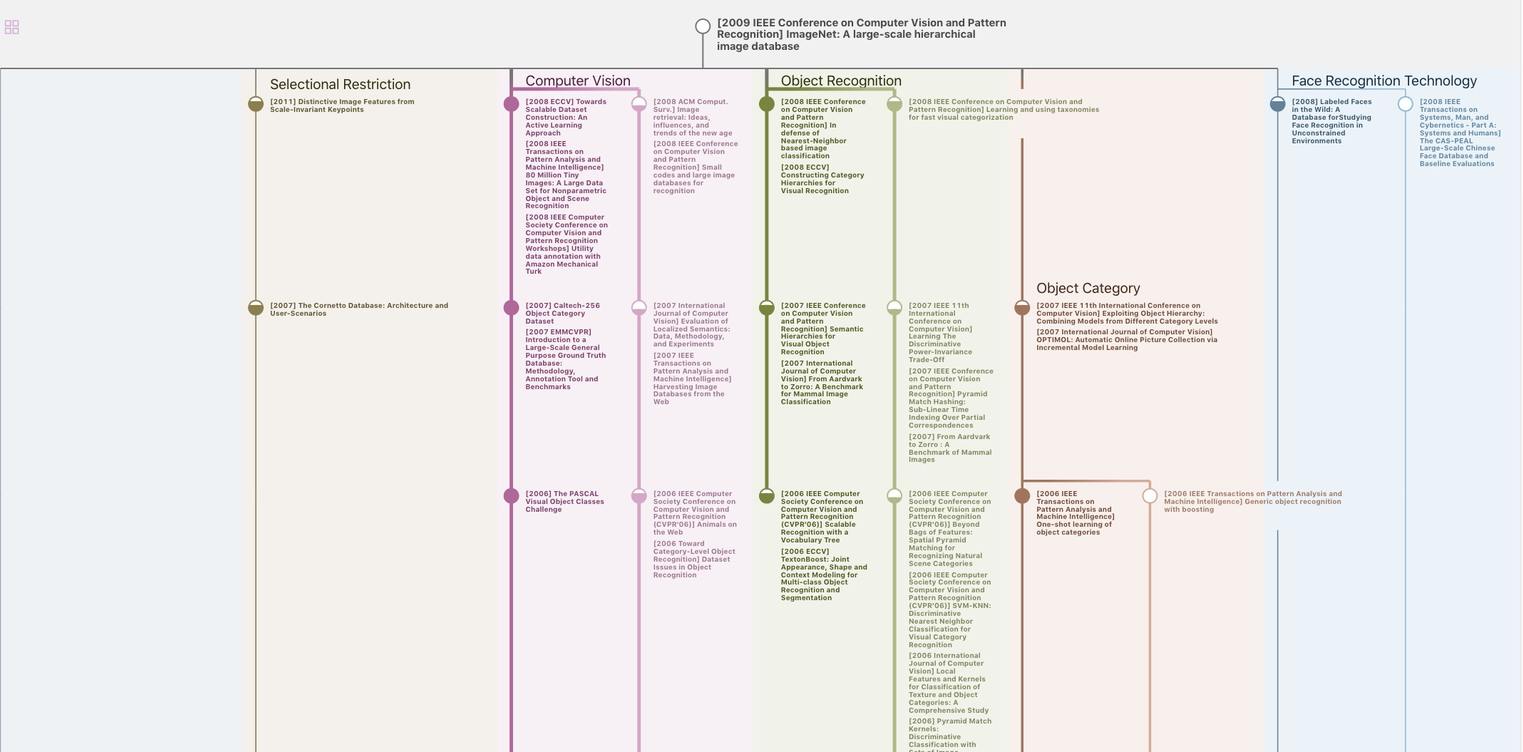
生成溯源树,研究论文发展脉络
Chat Paper
正在生成论文摘要