Computationally efficient physics approximating neural networks for highly nonlinear maps.
Research in Adaptive and Convergent Systems (RACS)(2022)
摘要
This paper puts forth a method that is easily transposable to a realtime environment by utilising a "Physics Approximating Neural Network" to predict 1D output signal. The technique described in this paper is inspired by Physics Informed Neural Networks put forth by Raissi et al. The model demonstrated in this paper makes use of a recurrent input. This is passed to a "preprocessing" stage of 2 layers by 8 neurons wide. The output of the preprocessing stage is passed to the "approximation layer". Lastly, the output of the "approximation layer" is passed to a "postprocessing" layer of 5 layers by 8 neurons wide. The architecture of the model is explained and tested on a specially developed dataset. The results show closer similarity to the ground truth compared to a linear model or a dense-layer neural network.
更多查看译文
AI 理解论文
溯源树
样例
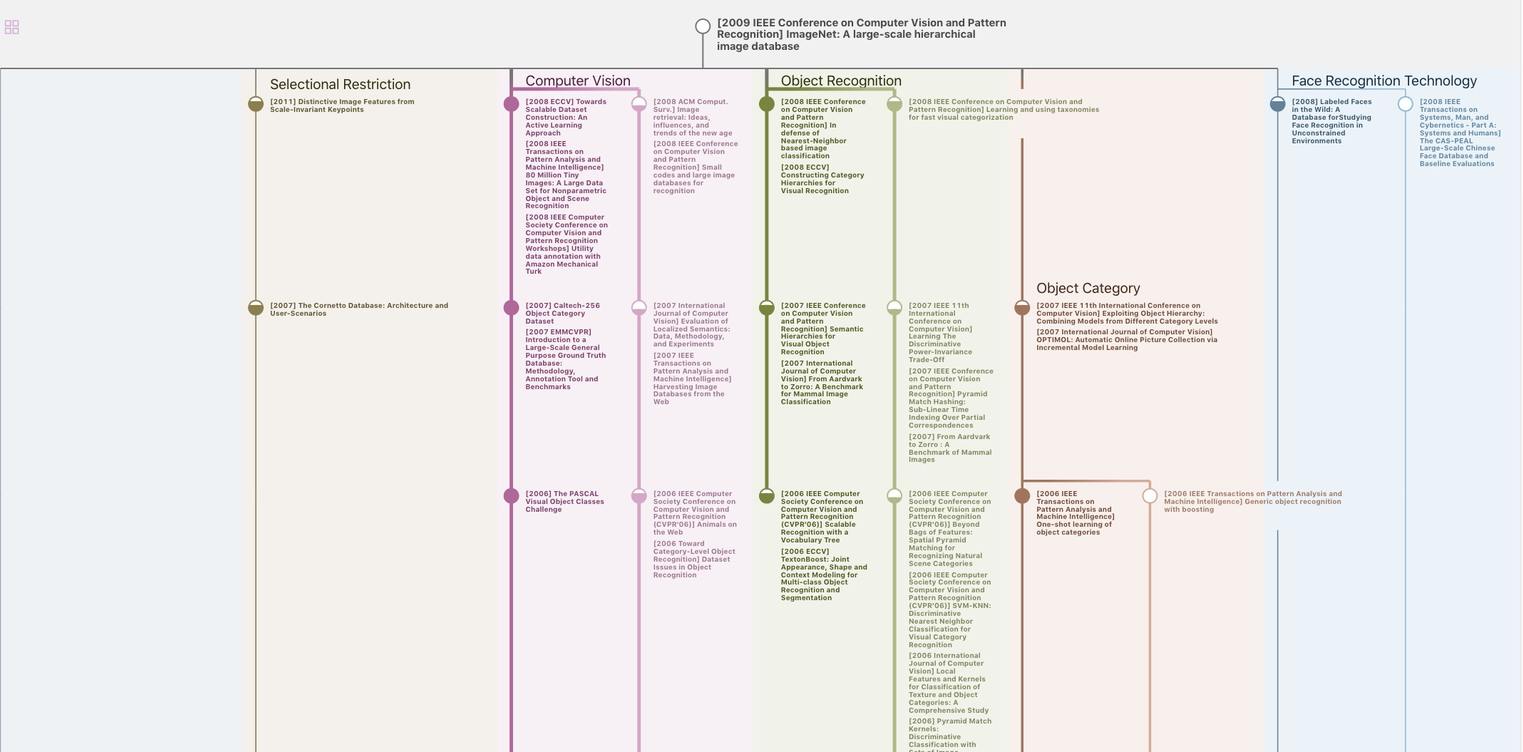
生成溯源树,研究论文发展脉络
Chat Paper
正在生成论文摘要