TensorHE: a homomorphic encryption transformer for privacy-preserving deep learning.
Research in Adaptive and Convergent Systems (RACS)(2022)
摘要
In recent years, deep learning (DL) techniques have grown rapidly and facilitated advancements in different applications. With the concerns about information security increasing, the privacy sensitivity of machine learning algorithms needs to be taken into consideration. Homomorphic encryption (HE) based solutions have been proposed to preserve data privacy while enjoying the benefits of deep learning technologies. However, the existing approaches require extensive expert knowledge and human attention, which hampers the opportunities for promoting technologies in different application domains. In this work, we developed a transformer, called TensorHE , to automatically convert a pre-trained convolutional neural network (CNN) into the HE-based version. A systematical method is proposed to facilitate automatic transformation, enabling to build and deploy domain-specific applications. Our experimental results demonstrate the applicability and scalability of TensorHE by converting the CNN models for handling the MNIST and CIFAR-10 datasets. The delivered accuracies of the transformed models are as close as (less than 3%) those of the input CNN models.
更多查看译文
AI 理解论文
溯源树
样例
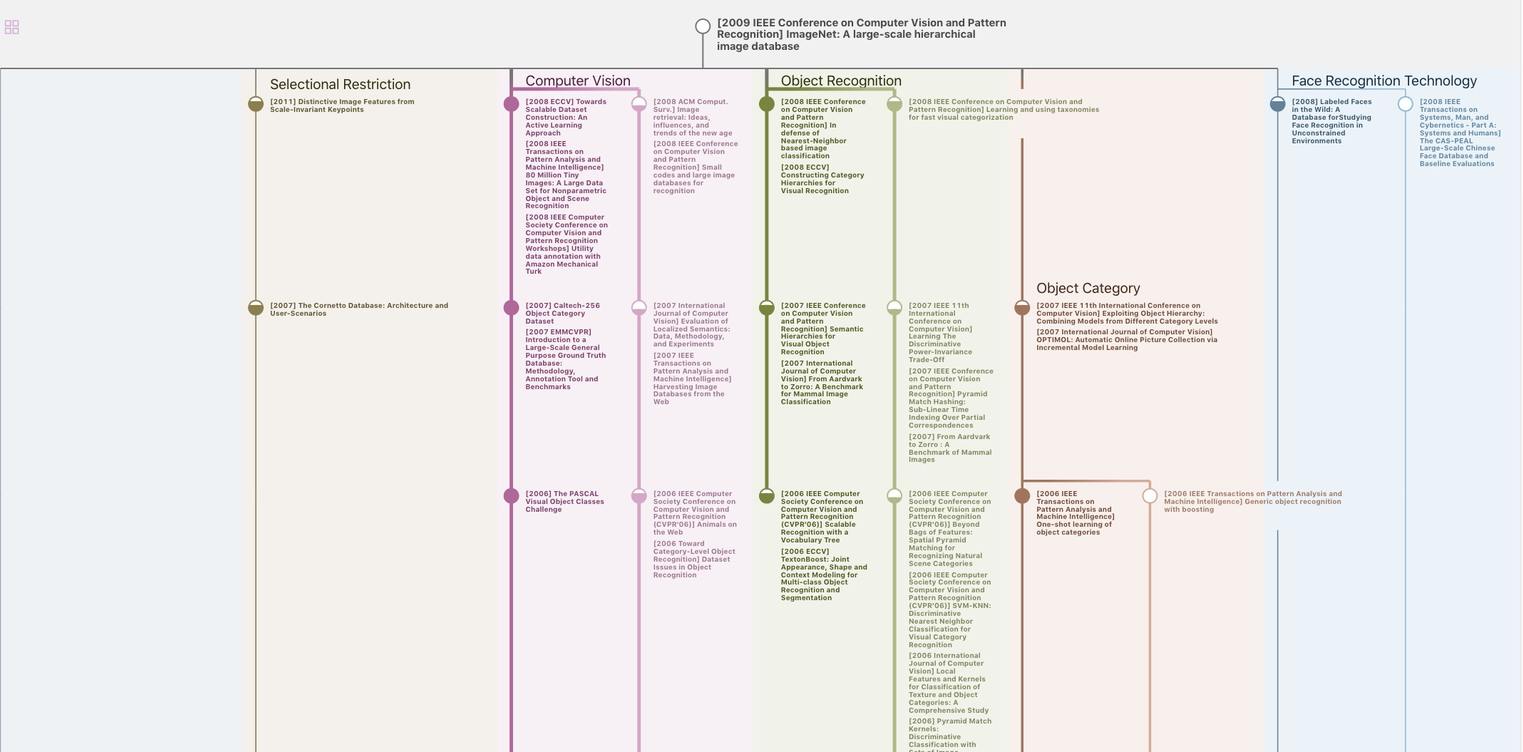
生成溯源树,研究论文发展脉络
Chat Paper
正在生成论文摘要