District-Coupled Epidemic Control via Deep Reinforcement Learning.
Knowledge Science, Engineering and Management (KSEM)(2022)
摘要
The rapid spread of the Coronavirus (COVID-19) poses an unprecedented threat to the public health system and social economy, with approximately 500 million confirmed cases worldwide. Policymakers confront with high-stakes to make a decision on interventions to prevent the pandemic from further spreading, which is a dilemma between public health and a steady economy. However, the epidemic control problem has vast solution space and its internal dynamic is driven by population mobility, which makes it difficult for policymakers to find the optimal intervention strategy based on rules-of-thumb. In this paper, we propose a Deep Reinforcement Learning enabled Epidemic Control framework (DRL-EC) to make a decision on intervention to effectively alleviate the impacts of the epidemic outbreaks. Specifically, it is driven by reinforcement learning to learn the intervention policy autonomously for the policymaker, which can be adaptive to the various epidemic situation. Furthermore, District-Coupled Susceptible-Exposed-Infected-Recovered (DC-SEIR) model is hired to simulate the pandemic transmission between inter-district, which characterize the spatial and temporal nature of infectious disease transmission simultaneously. Extensive experimental results on a real-world dataset, the Omicron local outbreaks in China, demonstrate the superiority of the DRL-EC compared with the strategy based on rules-of-thumb.
更多查看译文
关键词
COVID-19,Epidemic control,Intervention strategy,Deep reinforcement learning,Epidemiological model
AI 理解论文
溯源树
样例
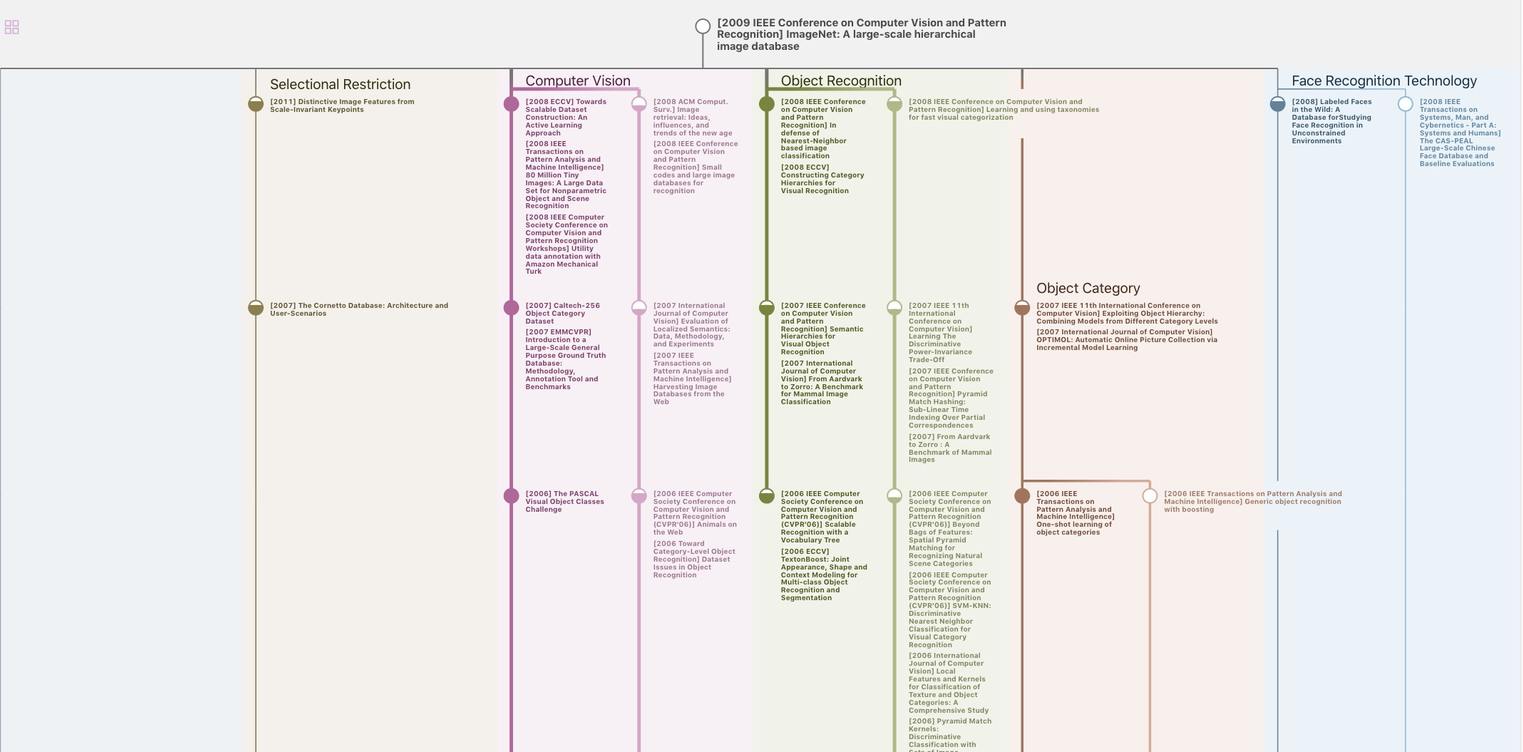
生成溯源树,研究论文发展脉络
Chat Paper
正在生成论文摘要