Improve Calibration Robustness of Temperature Scaling by Penalizing Output Entropy.
International Syposium on Methodologies for Intelligent Systems (ISMIS)(2022)
摘要
Data corruption is usually encountered in captured images due to the light condition, weather condition, or the quality of devices. Calibrating deep neural network (DNN) based image classifiers under data corruption is crucial, especially in safety-critical applications. A recent study shows that the widespread post-hoc calibration method temperature scaling (TS) performs poorly under corrupted shifts because it is easy to overfit the validation set. Three issues need to be addressed when improving the calibration robustness of TS: (1) How to measure the data shifts due to corruption? (2) How to reformulate TS with measured metrics? (3) How to improve the robustness of TS? Observing that output entropy increases with data shift intensity caused by corruption, we incorporate an entropy term into TS' optimizing Negative Log-likelihood (NLL) problem (Q1). Since the two terms of loss function are mutually exclusive, we reformulate TS as a multi-objective optimization (MOO) problem (Q2). By solving the MOO problem, a set of scaling parameters can thus be obtained and integrated to improve the calibration robustness of TS (Q3). We propose a novel TS method named MOO-ETS based on the above solutions, consisting of two integration strategies. Experimental results on the corrupted versions of CIFAR-10, CIFAR-100, and TinyImageNet demonstrate that: (1) entropy can measure corrupted data shifts accurately; (2) MOO-ETS can achieve competitive performance under corrupted shifts compared with the state-of-the-art method Deep Ensemble and beat the TS family baselines remarkably.
更多查看译文
关键词
Confidence calibration,Multi-objective optimization,Temperature scaling,Image classification
AI 理解论文
溯源树
样例
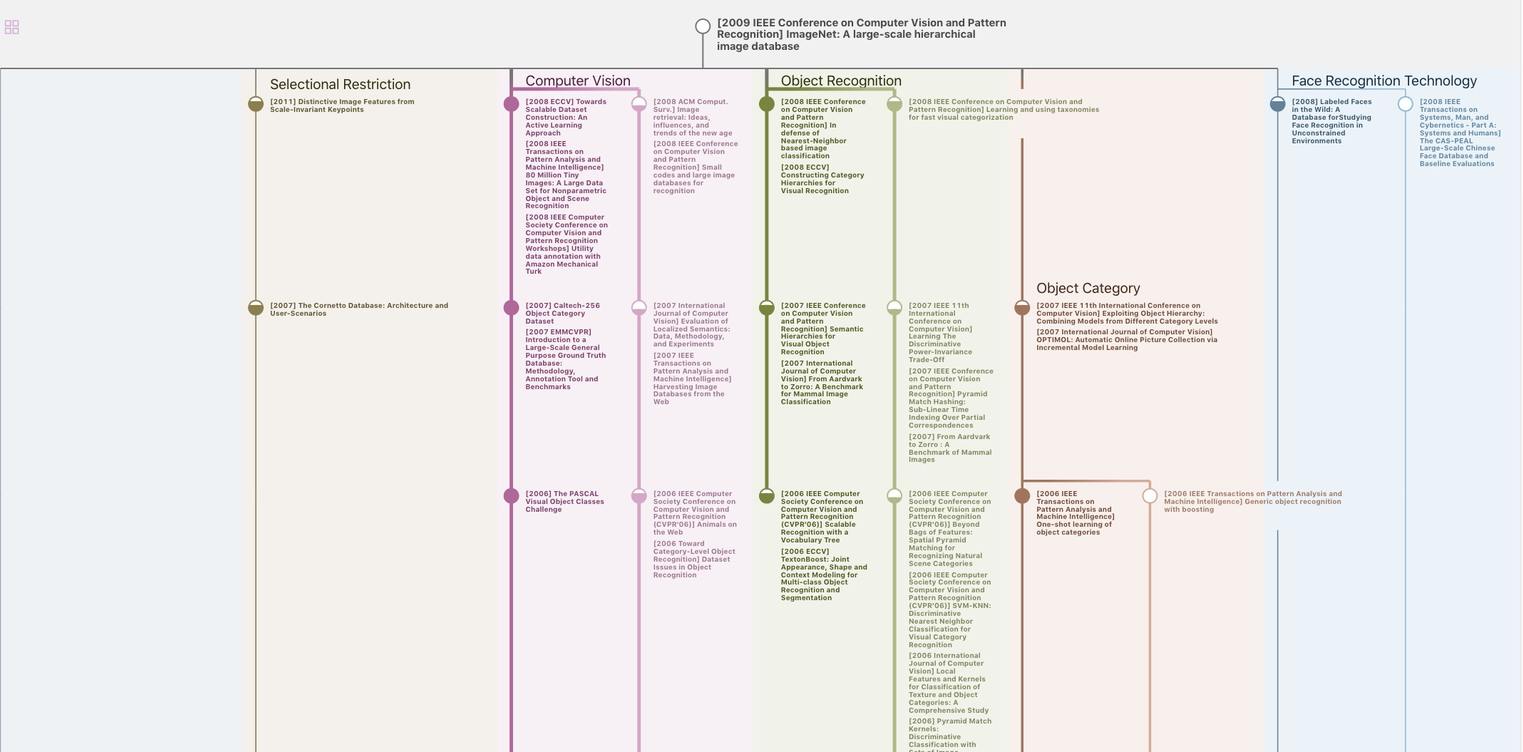
生成溯源树,研究论文发展脉络
Chat Paper
正在生成论文摘要