Simple Binary Hypothesis Testing under Communication Constraints.
International Symposium on Information Theory (ISIT)(2022)
摘要
We study simple binary hypothesis testing under communication constraints, a.k.a. “decentralized detection”. Here, each sample is mapped to a message from a finite set of messages via a channel before being revealed to a statistician. In the absence of communication constraints, it is well known that the sample complexity is characterized by the Hellinger distance between the distributions. We show that the sample complexity of hypothesis testing under communication constraints is at most a logarithmic factor larger than in the unconstrained setting, and demonstrate that distributions exist in which this characterization is tight. We also provide a polynomial-time algorithm which achieves the aforementioned sample complexity. Our proofs rely on a new reverse data processing inequality and a reverse Markov’s inequality, which may be of independent interest.
更多查看译文
关键词
simple binary hypothesis testing,communication constraints,aforementioned sample complexity,reverse Markov inequality
AI 理解论文
溯源树
样例
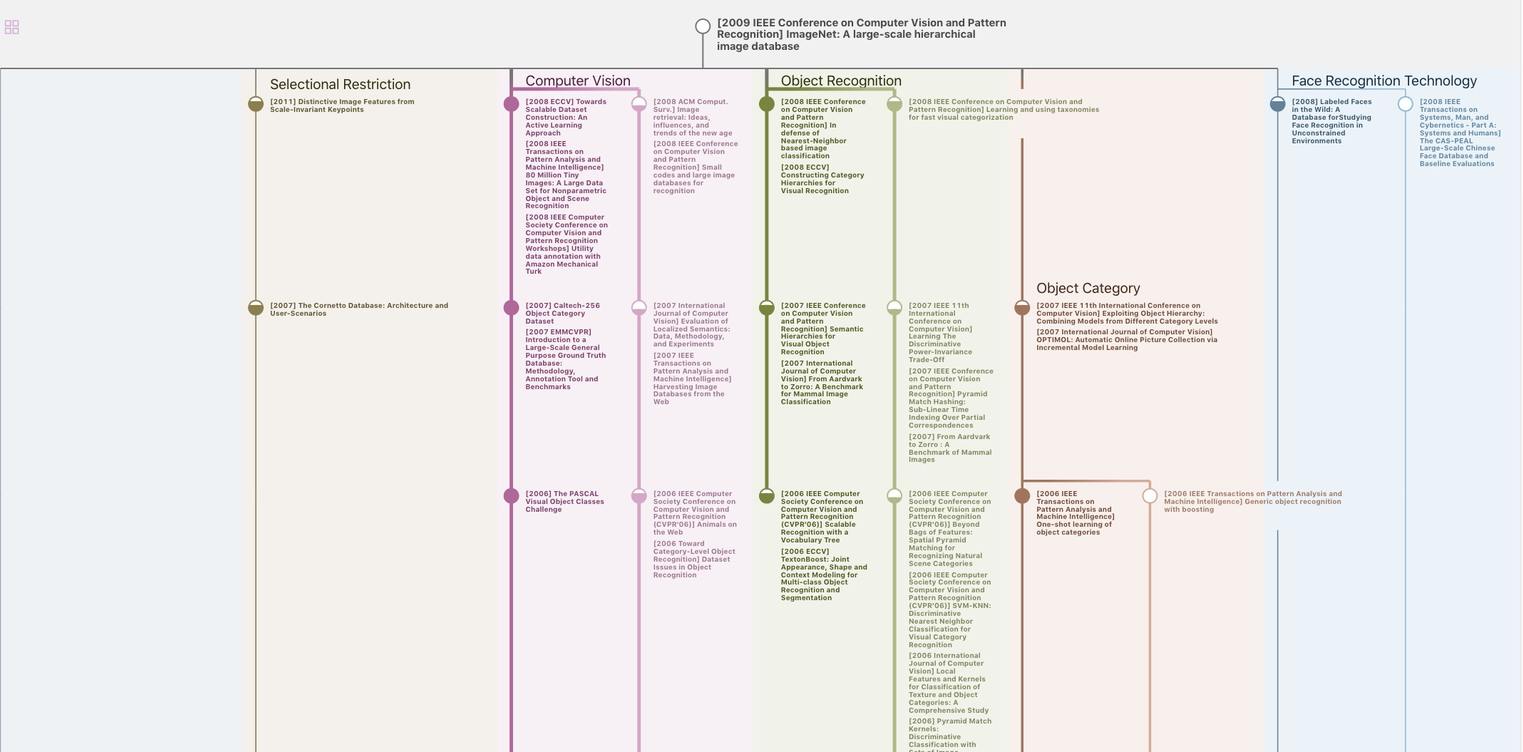
生成溯源树,研究论文发展脉络
Chat Paper
正在生成论文摘要