Simultaneous Sensing and Channel Access based on Partial Observations via Deep Reinforcement Learning.
International Symposium on Information Theory (ISIT)(2022)
摘要
This paper is eligible for the Jack Keil Wolf ISIT Student Paper Award. In this paper we study dynamic spectrum access (DSA) in cognitive wireless networks, consisting of primary users (PUs) and a secondary user (SU) which has only partial observations. The traffic patterns of the PUs are modeled as finite-memory Markov chains, and are unknown to the SU. It is noted that as observations are partial, then both channel sensing and channel access actions affect the throughput. Our objective in this work is to design a DSA algorithm such that the SU’s long-term throughput is maximized. To that aim, we show theoretically that the DSA problem can be formulated as a single-agent problem with a single policy for both sensing and access, and propose a novel algorithm that learns both the optimal access policy and the optimal sensing policy via deep Q-learning, which is referred to as Double Deep Q-network for Sensing and Access (DDQSA). To the best of our knowledge, this is the first instance of a deep Q-learning-based DSA algorithm, which learns both sensing and access policies. Our results show that the DDQSA algorithm learns a policy that implements both sensing and channel access, and achieves significantly better performance compared to existing approaches.
更多查看译文
关键词
Cognitive radio networks,deep reinforcement learning,dynamic spectrum access,wireless channels
AI 理解论文
溯源树
样例
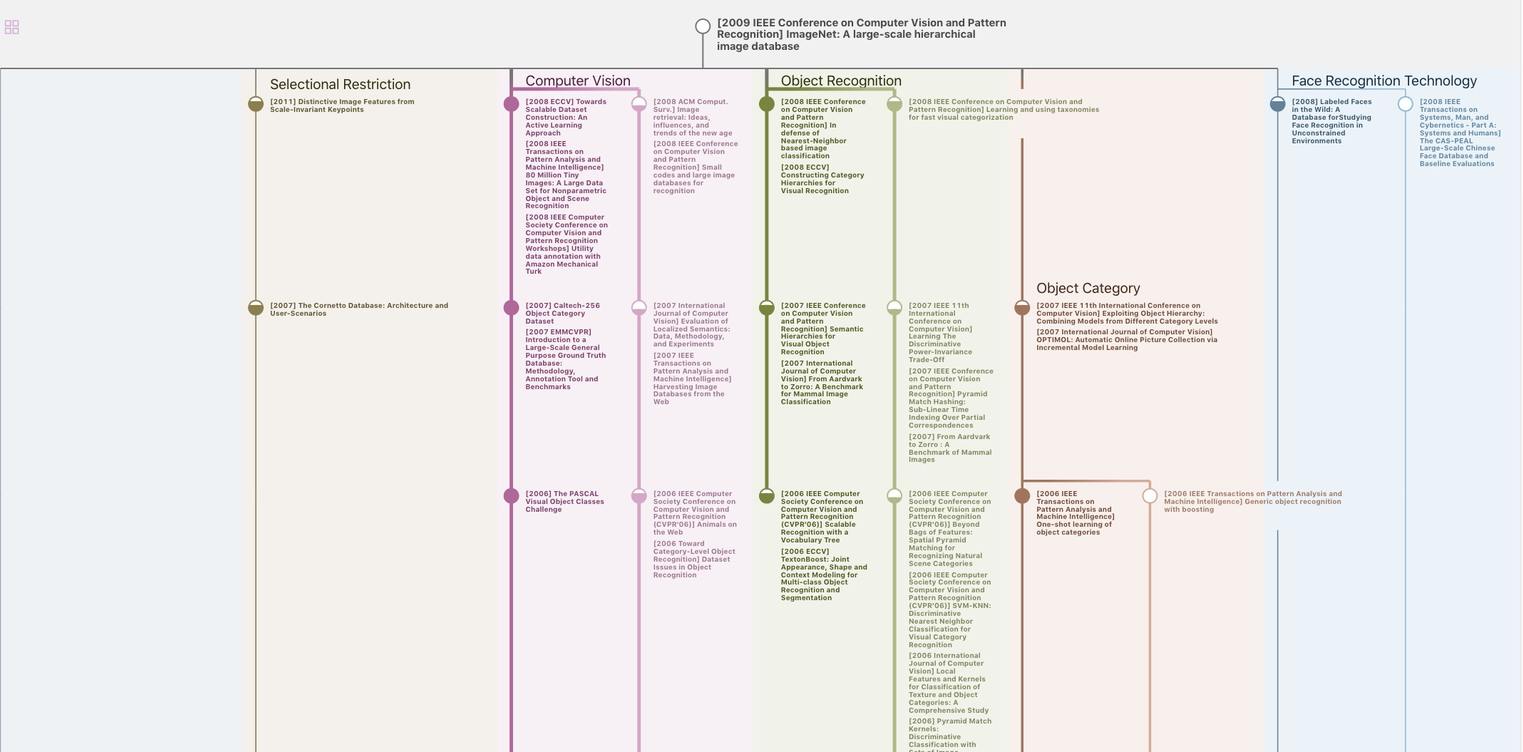
生成溯源树,研究论文发展脉络
Chat Paper
正在生成论文摘要