Mitigating Noise in Ensemble Classification with Real-Valued Base Functions.
International Symposium on Information Theory (ISIT)(2022)
摘要
In data-intensive applications, it is advantageous to perform some partial processing close to the data, and communicate to a central processor the partial results instead of the data itself. When the communication medium is noisy, one must mitigate the resulting degradation in computation quality. We study this problem for the setup of binary classification performed by an ensemble of functions communicating real-valued confidence levels. We propose a noise-mitigation solution that works by optimizing the aggregation coefficients at the central processor. Toward that, we formulate a post-training gradient algorithm that minimizes the error probability given the dataset and the noise parameters. We further derive lower and upper bounds on the optimized error probability, and show empirical results that demonstrate the enhanced performance achieved by our scheme on real data.
更多查看译文
关键词
ensemble classification,real-valued base functions,data-intensive applications,partial processing close,central processor,communication medium,computation quality,binary classification,real-valued confidence levels,noise-mitigation solution,aggregation coefficients,post-training gradient algorithm,noise parameters,lower bounds,upper bounds,optimized error probability
AI 理解论文
溯源树
样例
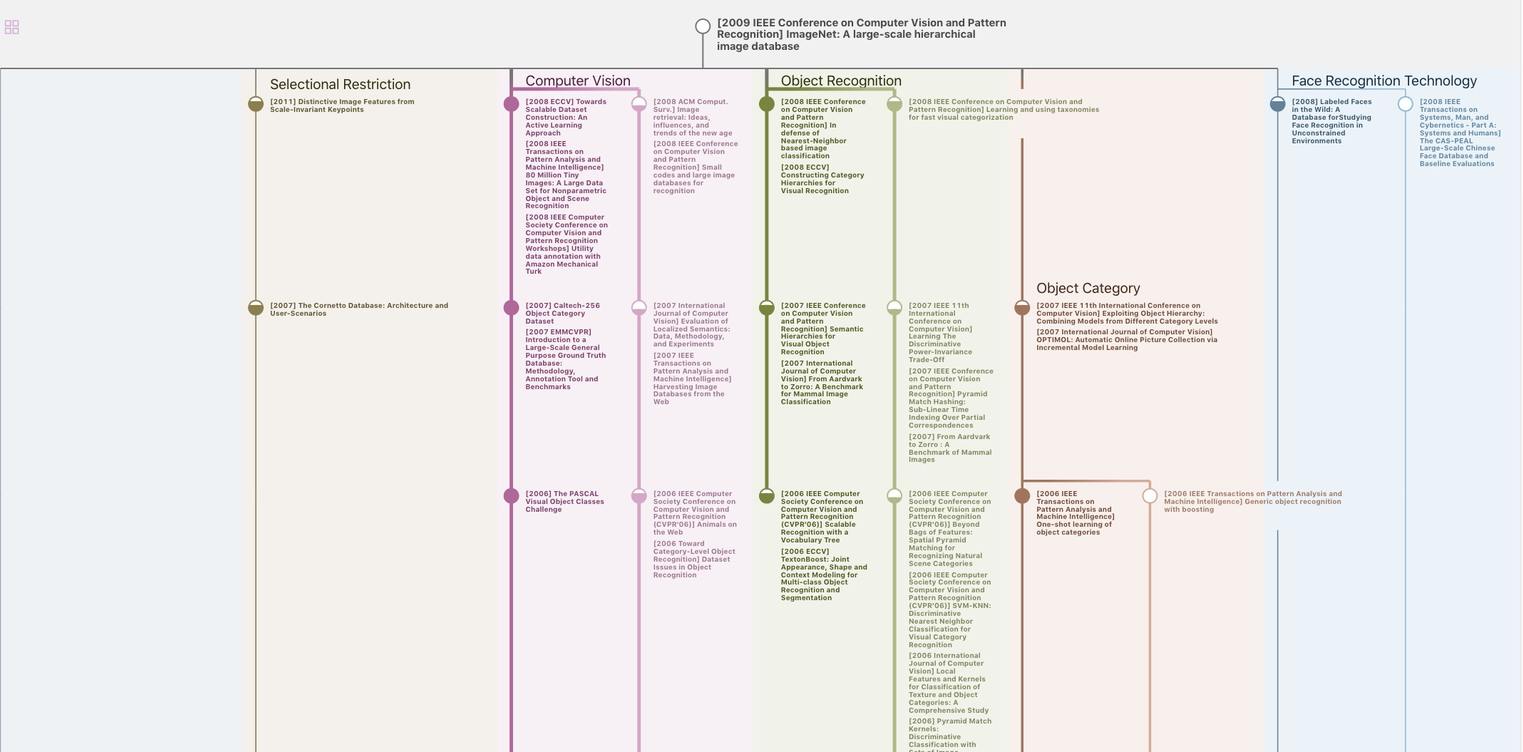
生成溯源树,研究论文发展脉络
Chat Paper
正在生成论文摘要