An improvement of Random Node Generator for the uniform generation of capacities
ANNALS OF MATHEMATICS AND ARTIFICIAL INTELLIGENCE(2023)
摘要
Capacity is an important tool in decision-making under risk and uncertainty and multi-criteria decision-making. When learning a capacity-based model, it is important to be able to generate uniformly a capacity. Due to the monotonicity constraints of a capacity, this task reveals to be very difficult. The classical Random Node Generator (RNG) algorithm is a fast-running speed capacity generator, however with poor performance. In this paper, we firstly present an exact algorithm for generating a n elements’ general capacity, usable when n < 5 . Then, we present an improvement of the classical RNG by studying the distribution of the value of each element of a capacity. Furthermore, we divide it into two cases, the first one is the case without any conditions, and the second one is the case when some elements have been generated. Experimental results show that the performance of this improved algorithm is much better than the classical RNG while keeping a very reasonable computation time.
更多查看译文
关键词
Random generation,Capacity,Linear extension
AI 理解论文
溯源树
样例
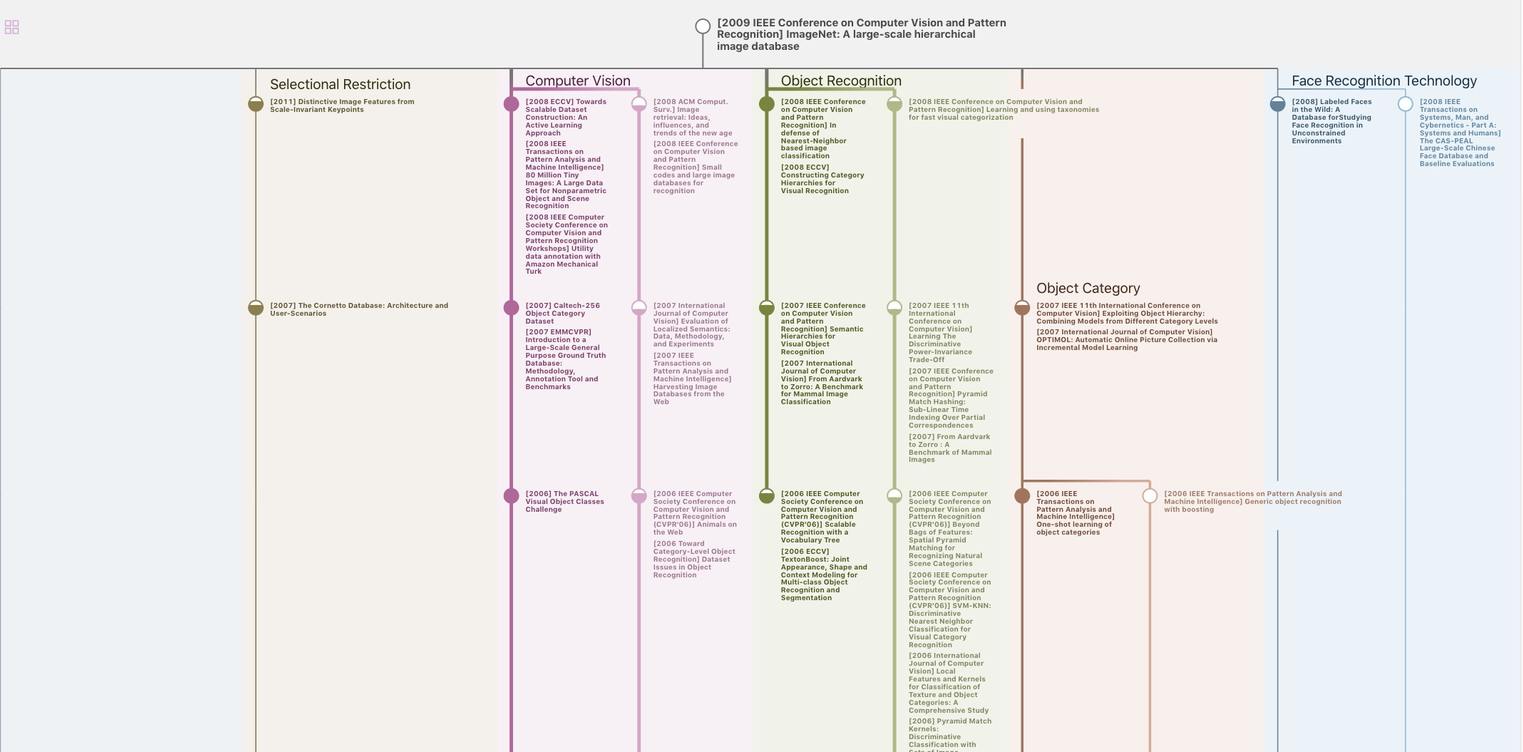
生成溯源树,研究论文发展脉络
Chat Paper
正在生成论文摘要