Comparing Approaches to Language Understanding for Human-Robot Dialogue: An Error Taxonomy and Analysis.
International Conference on Language Resources and Evaluation (LREC)(2022)
摘要
In this paper, we compare two different approaches to language understanding for a human-robot interaction domain in which a human commander gives navigation instructions to a robot. We contrast a relevance-based classifier with a GPT-2 model, using about 2000 input-output examples as training data. With this level of training data, the relevance-based model outperforms the GPT-2 based model 79% to 68%, and an Oracle combination set an upper-bound of 85%. We also present a taxonomy of types of errors made by each model, indicating that they have somewhat different strengths and weaknesses, so we also examine the potential for a combined model.
更多查看译文
关键词
Dialogue, Human-Robot Interaction, Evaluation, Error Taxonomy
AI 理解论文
溯源树
样例
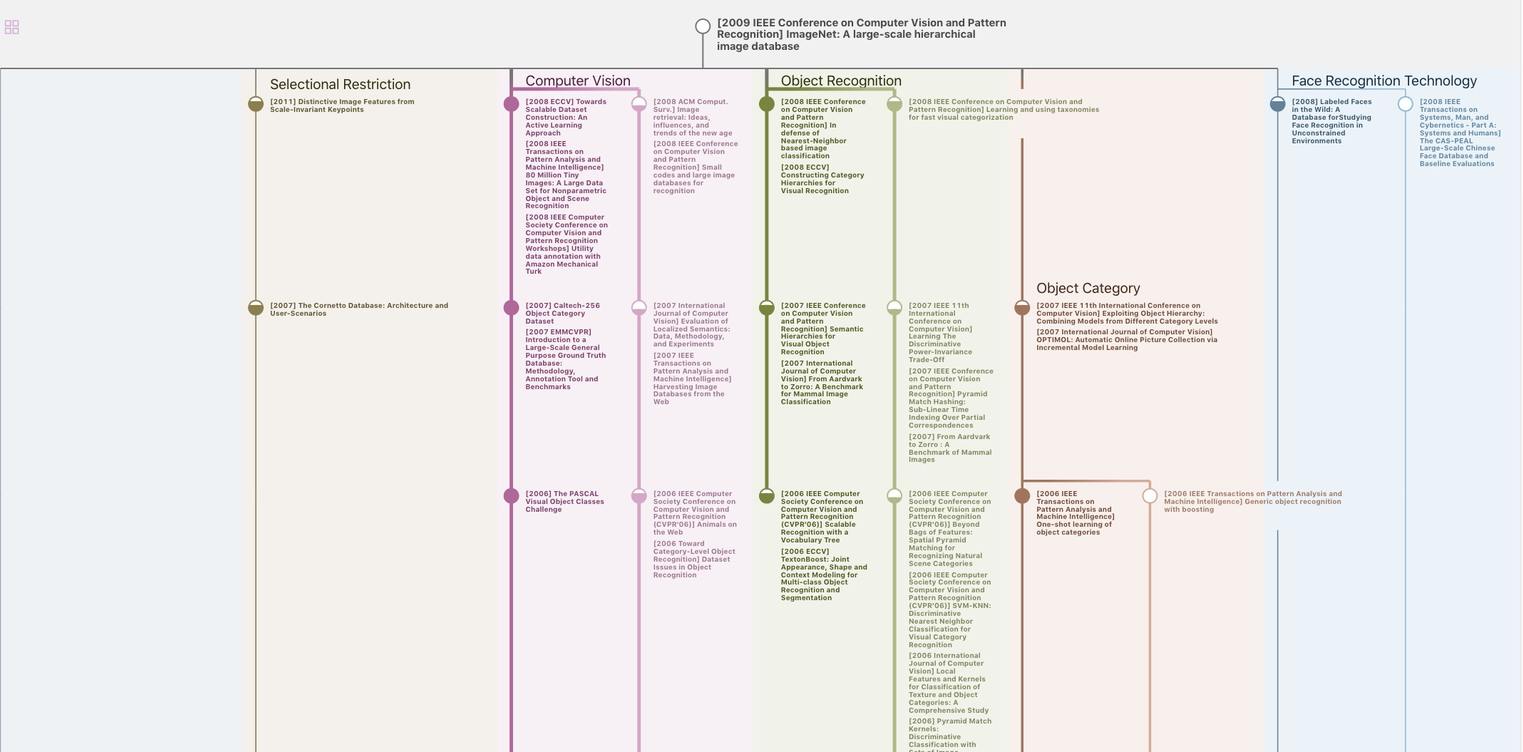
生成溯源树,研究论文发展脉络
Chat Paper
正在生成论文摘要