A Generalized Approach to Protest Event Detection in German Local News.
International Conference on Language Resources and Evaluation (LREC)(2022)
摘要
Protest events provide information about social and political conflicts, the state of social cohesion and democratic conflict management, as well as the state of civil society in general. Social scientists are therefore interested in the systematic observation of protest events. With this paper, we release the first German language resource of protest event related article excerpts published in local news outlets. We use this dataset to train and evaluate transformer-based text classifiers to automatically detect relevant newspaper articles. Our best approach reaches a binary F1-score of 93.3 %, which is a promising result for our goal to support political science research. However, in a second experiment, we show that our model does not generalize equally well when applied to data from time periods and localities other than our training sample. To make protest event detection more robust, we test two ways of alternative article preprocessing. First, we find that letting the classifier concentrate on sentences around protest keywords only slightly improves the performance for in-sample data. For out-of-sample data, in contrast, binary F1-scores improve up to +4 percentage points (pp). Second, against our initial intuition, masking of named entities during preprocessing does not improve the generalization of protest event detection models in terms of F1-scores. However, it leads to a significantly improved recall of the models.
更多查看译文
关键词
protest event detection, protest event analysis, text classification, computational social science
AI 理解论文
溯源树
样例
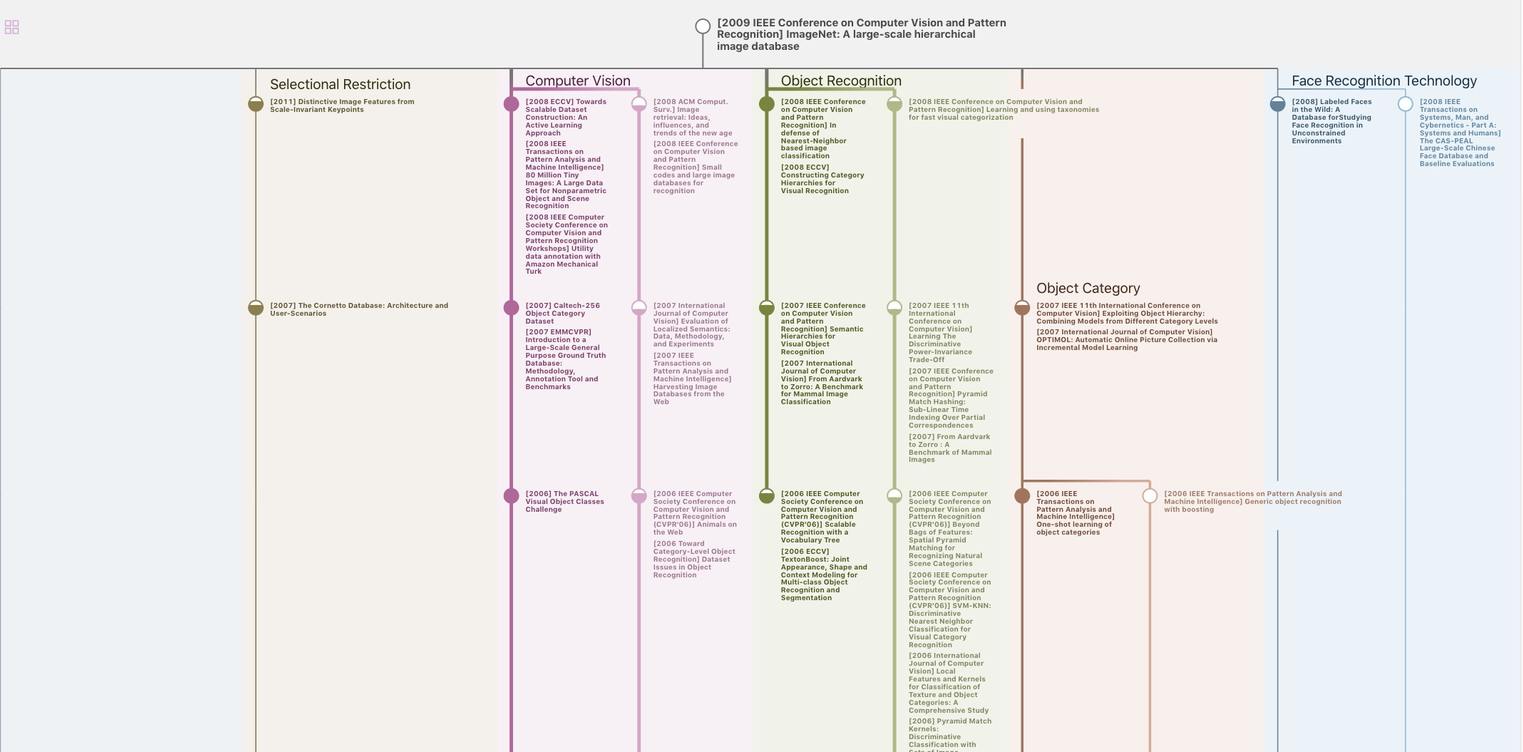
生成溯源树,研究论文发展脉络
Chat Paper
正在生成论文摘要