Dynamic Car-following Model Calibration with Deep Reinforcement Learning.
International Conference on Intelligent Transportation Systems (ITSC)(2022)
摘要
In microscopic traffic simulation, it is apparent that there is a dilemma between physics-based and learning-based models for modelling car-following behaviours. The former can offer analytical insights with low simulation accuracy while the latter functions like a black-box, but offers high simulation accuracy. Thus, a new perspective on combining physics-based car-following models (CFM) and learning-based methods is given in this paper by integrating the two approaches through "model calibration". The CFM calibration is formulated as a sequential decision-making process via deep reinforcement learning (DRL), and a general framework is provided to achieve this purpose. To the best of our knowledge, this is the first time in literature that formulates dynamic CFM calibration using DRL. The experiment results have shown that our DRL-based dynamic calibration method, d-DDPG, has outperformed more conventional method such as Genetic Algorithm with 26.80% and 23.16% reduction in terms of root mean squared spacing error and position trajectory deviation error, respectively. Therefore, our work could serve as a promising initiative towards this new research direction.
更多查看译文
关键词
model calibration,reinforcement learning,car-following
AI 理解论文
溯源树
样例
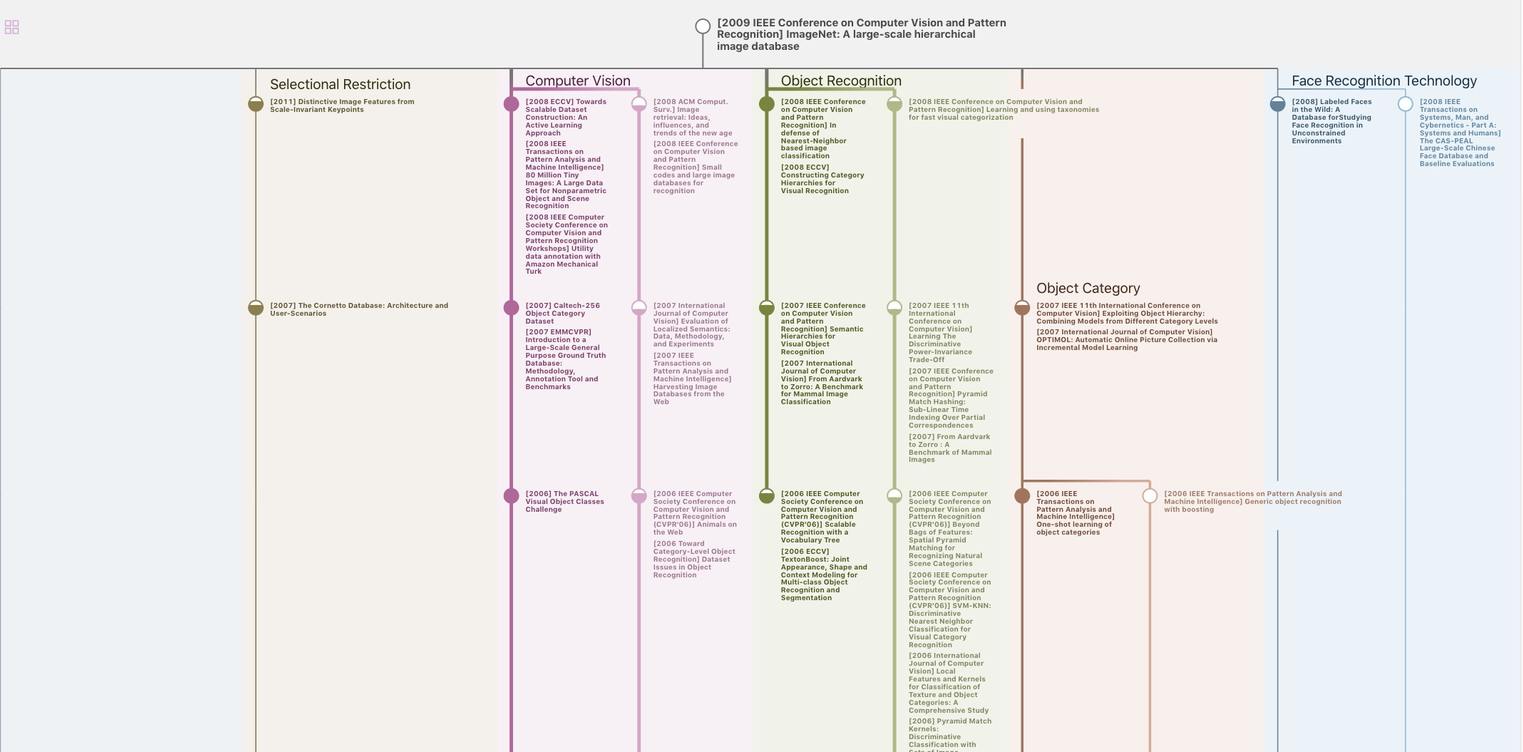
生成溯源树,研究论文发展脉络
Chat Paper
正在生成论文摘要