Hybrid Spatio-Temporal Graph Convolution Network For Short-Term Traffic Forecasting.
International Conference on Intelligent Transportation Systems (ITSC)(2022)
摘要
Urban short-time traffic prediction is one of the most important services in smart city transportation and it is becoming increasingly important as a fundamental service in vehicle navigation systems and intelligent transportation systems. In this paper, a machine learning method for short-time traffic speed prediction based on floating vehicle data is proposed. Firstly, we describe short-time traffic speed prediction as a purely spatio-temporal regression prediction problem based on effective features in multiple dimensions. Secondly, we use three separate temporal feature extractors collect the sequence linkages of weekly, daily, and nearest-neighbor periods to solve the prediction task with multiple time steps. Based on this modelling idea, we propose an Hybrid Spatio-Temporal Graph Convolution Network (HSTGCN) model,which can accurately predict the future traffic speed of individual city roads for a given departure time. We evaluate our solution offline using hundreds of thousands of historical vehicle travel data, and the data results show that our proposed deep learning algorithm significantly outperforms state-of-the-art machine learning algorithms.
更多查看译文
关键词
traffic,graph,network,spatio-temporal,short-term
AI 理解论文
溯源树
样例
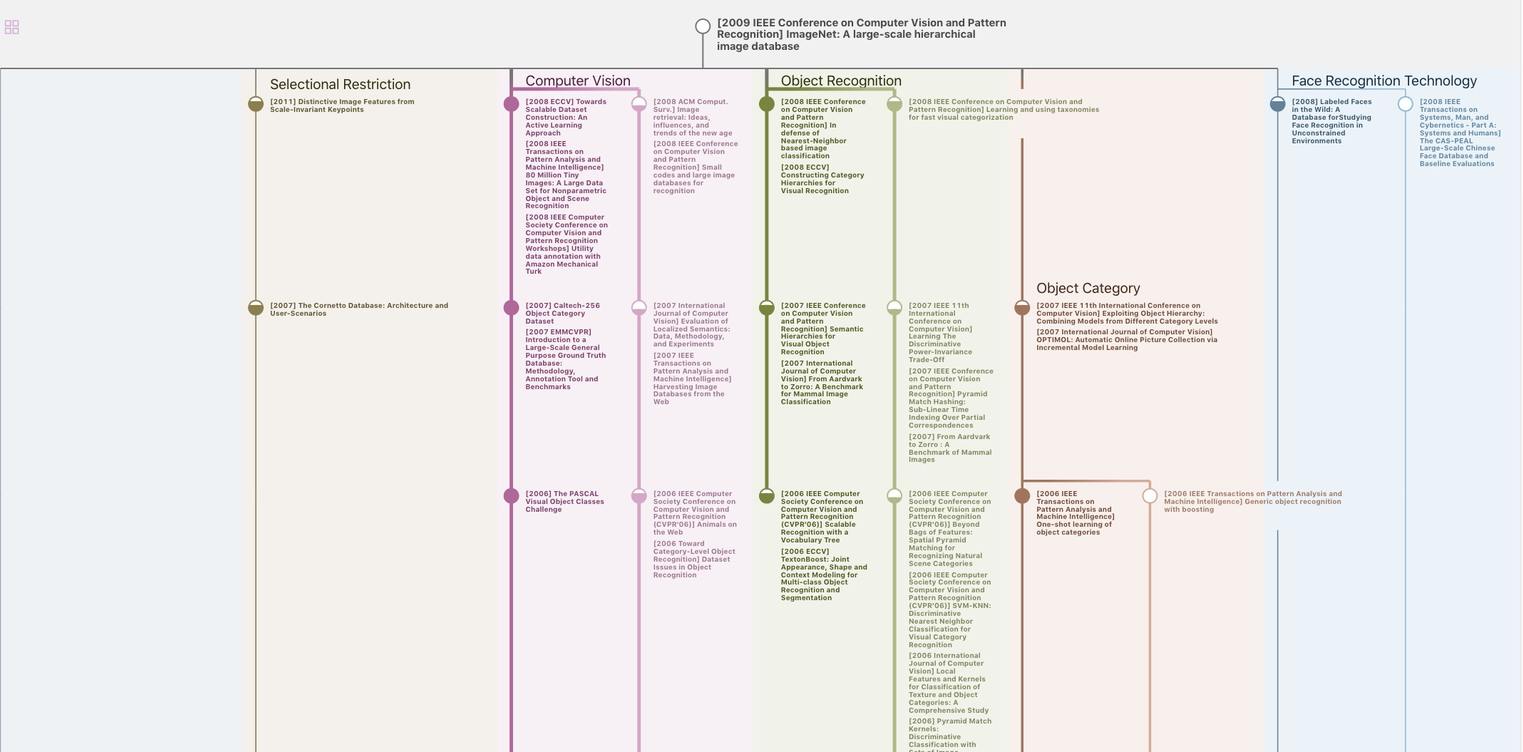
生成溯源树,研究论文发展脉络
Chat Paper
正在生成论文摘要