Exploring the Effect of 3D Object Removal using Deep Learning for LiDAR-based Mapping and Long-term Vehicular Localization.
International Conference on Intelligent Transportation Systems (ITSC)(2022)
摘要
The construction of a 3D point cloud map for long-term vehicle localization has always been a challenge in the autonomous driving community. In a highly dynamic urban environment, Simultaneous Localization and Mapping (SLAM) systems can be heavily affected by non-permanent objects and features during the mapping process, leaving undesired noise in the final map, which is redundant for long-term application. This paper explores applying object detection neural networks in SLAM systems to reduce the effect from dynamic objects (e.g., vehicles, pedestrians, cyclists). Specifically, we use PointPillars to detect and remove objects first and then perform LOAM to achieve a more static mapping. In the experimental section, the proposed method is validated on the public KITTI Odometry dataset. Compared with the original LOAM, the proposed method can provide a static point cloud map, thus improving the robustness of long-term vehicle applications.
更多查看译文
关键词
3d object removal,deep learning,lidar-based,long-term
AI 理解论文
溯源树
样例
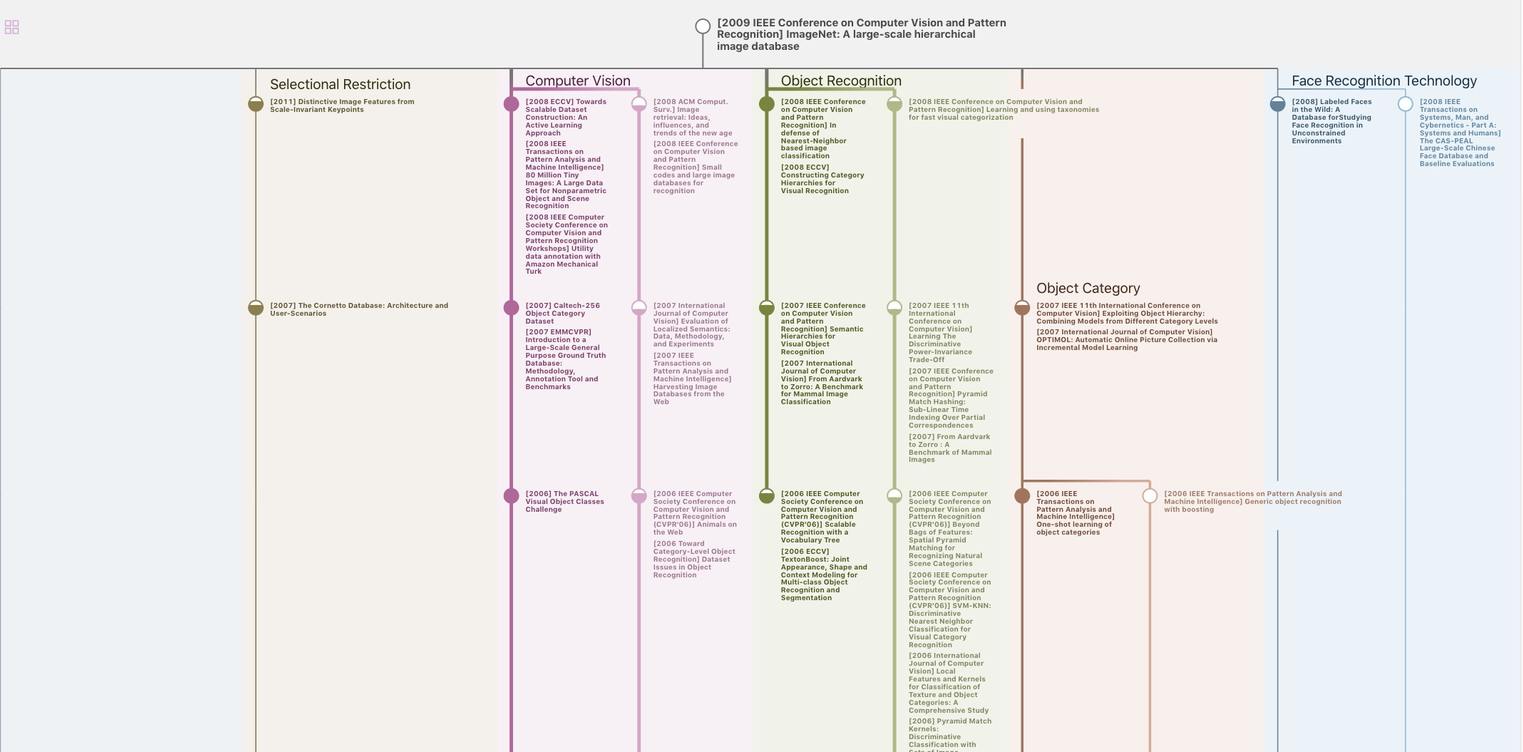
生成溯源树,研究论文发展脉络
Chat Paper
正在生成论文摘要