Land Cover Classification from Street-Level Photos
IEEE International Geoscience and Remote Sensing Symposium (IGARSS)(2022)
摘要
Land cover classification mapping is a process to depict terrestrial surfaces by pre-defined thematic classes across space and is often implemented by a supervised classification approach with satellite and/or aero images. However, due to labor-intensive and time-consuming tasks to identify land cover class from those images manually, it is challenging to build richquantity and highquality reference data sets for the mapping. To capture land covers on the ground, we developed a deep learning model to estimate land covers from street-level photos. A transfer learning from the pre-trained DenseNet was applied, and this model yields an overall accuracy of 0.91. This model contributes to the subsequent study of building a semi-automatic reference database from geo-tagged street-level photos.
更多查看译文
关键词
DenseNet,Mapillary,Reference data
AI 理解论文
溯源树
样例
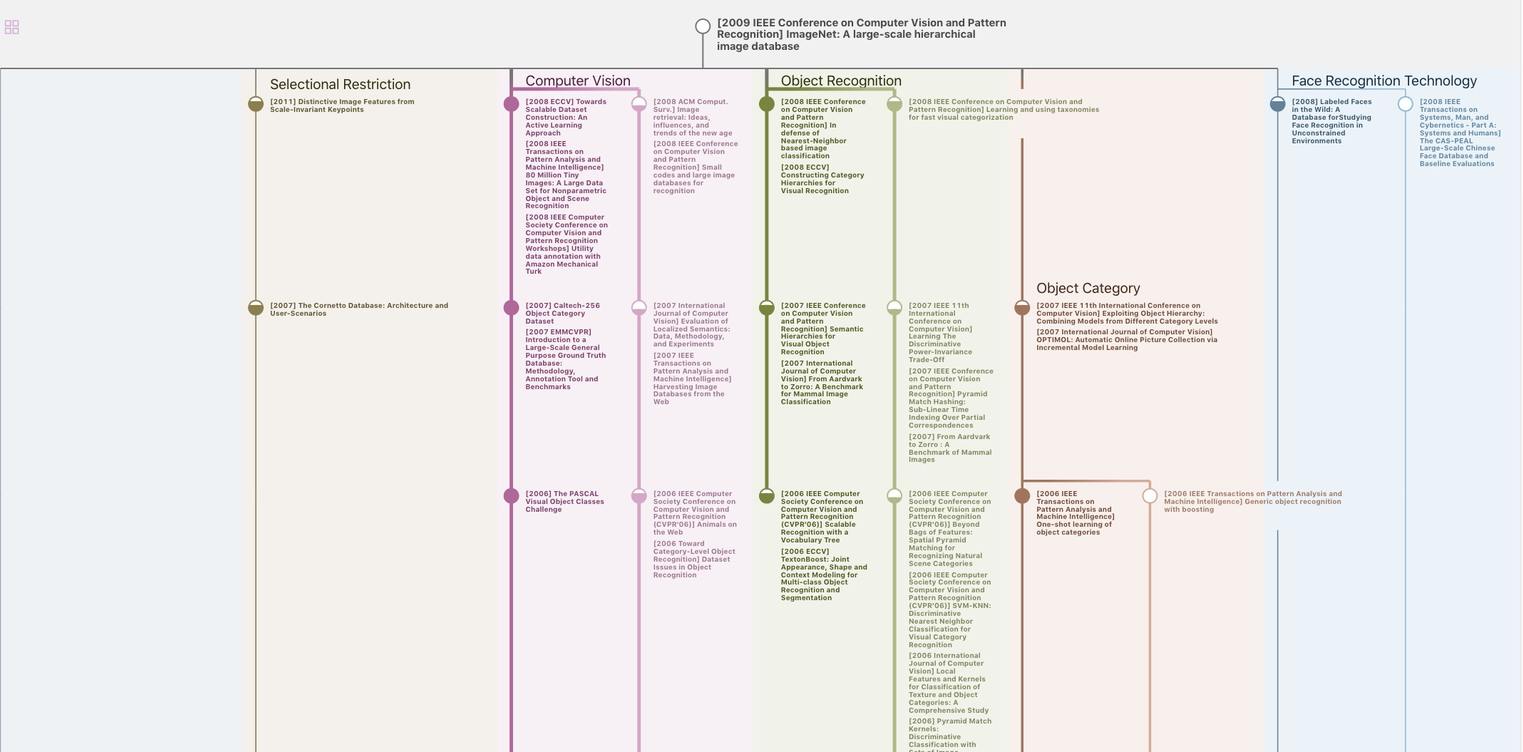
生成溯源树,研究论文发展脉络
Chat Paper
正在生成论文摘要