Toward Faster and Accurate Post-Disaster Damage Assessment: Development of End-to-End Building Damage Detection Framework with Super-Resolution Architecture.
IEEE International Geoscience and Remote Sensing Symposium (IGARSS)(2022)
摘要
Building damage detection ( BDD) with satellite images has been frequently adopted as an essential reference for post-disaster rescue, whereas its timeliness is significantly impacted by the long revisit time of high-resolution remote sensing satellites. Therefore, a reliable super-resolution method which is optimized for accurate and detail BDD is fundamental one for advancing the BDD analysis even when we can use only low-resolution images after a disaster. Based on Super- Resolution Generative Adversarial Network (SRGAN) and U-Net convolutional network, an efficient and novel BDD framework is proposed in this paper for obtaining upsampled BDD results from low-resolution post-disaster images. We trained the framework using two disasters from the xBD dataset and tested three different structures. The results show that our training structure based on an end-to-end framework successfully generated super-resolution BDD maps from low-resolution images, which performed significantly better than those from a two-stage training structure.
更多查看译文
关键词
Super-resolution, building damage, xBD dataset, end-to-end network, deep learning
AI 理解论文
溯源树
样例
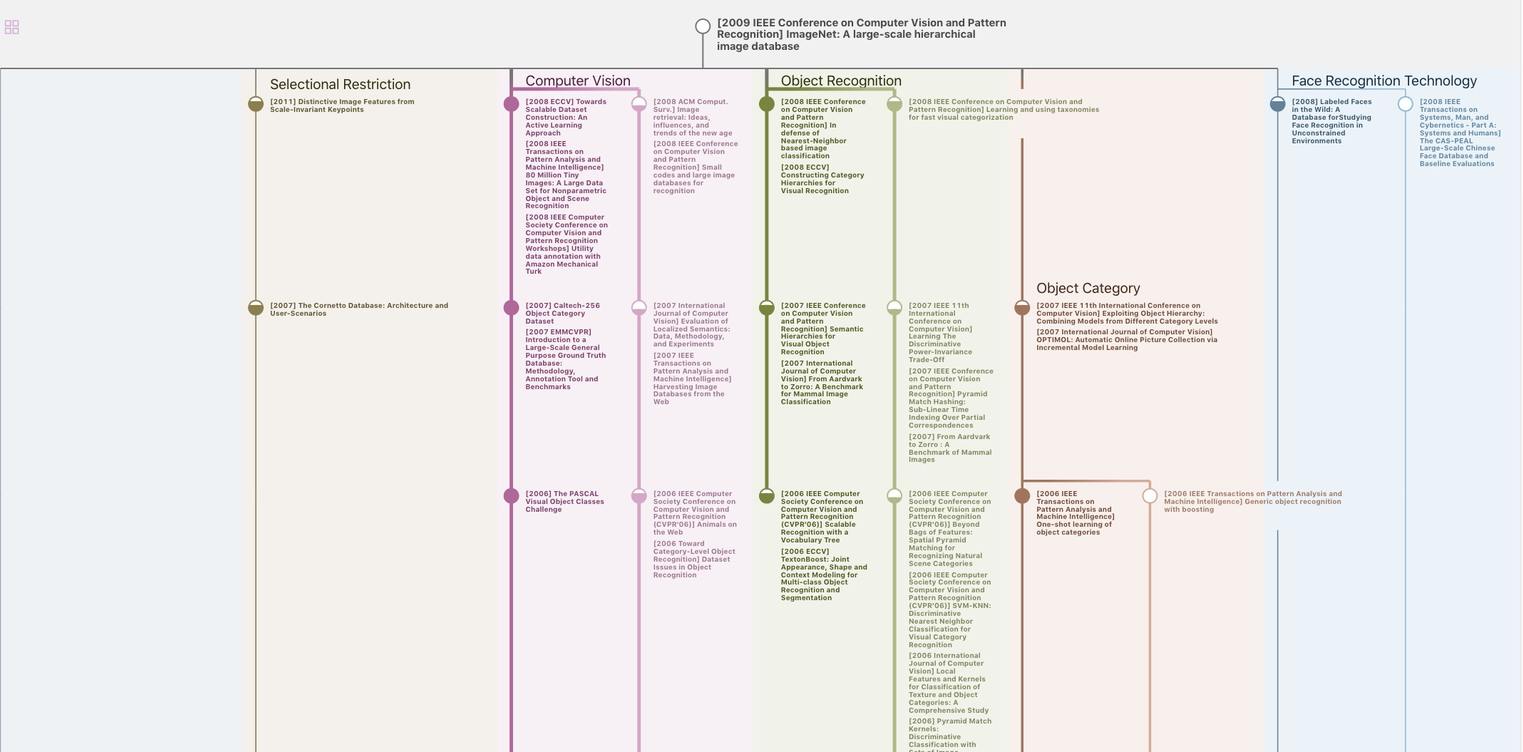
生成溯源树,研究论文发展脉络
Chat Paper
正在生成论文摘要