Estimating Uncertainty of Deep Learning Multi-Label Classifications Using Laplace Approximation.
IEEE International Geoscience and Remote Sensing Symposium (IGARSS)(2022)
摘要
Deep learning methods have become valuable tools in remote sensing for tasks like aerial scene classification or land cover analysis. Dealing with noisy and very varying data, the need for reliable confidence statements becomes apparent. While deep learning models are known to yield overconfident predictions, quantifying the model uncertainty of those classifiers can help mitigating that effect. Although uncertainty estimation methods for multi-class classification have been published, multi-label classification - the task of labelling data with multiple class labels simultaneously - has hardly been considered yet. In this study, we use multi-label Laplace Approximation to estimate the model uncertainty of deep multilabel classifiers and show how this method can improve calibration and out-of-distribution detection in the remote sensing domain.
更多查看译文
关键词
Multi-Label Classification, Bayesian Deep Neural Networks, Uncertainty Estimation, Laplace Approximation, Remote Sensing
AI 理解论文
溯源树
样例
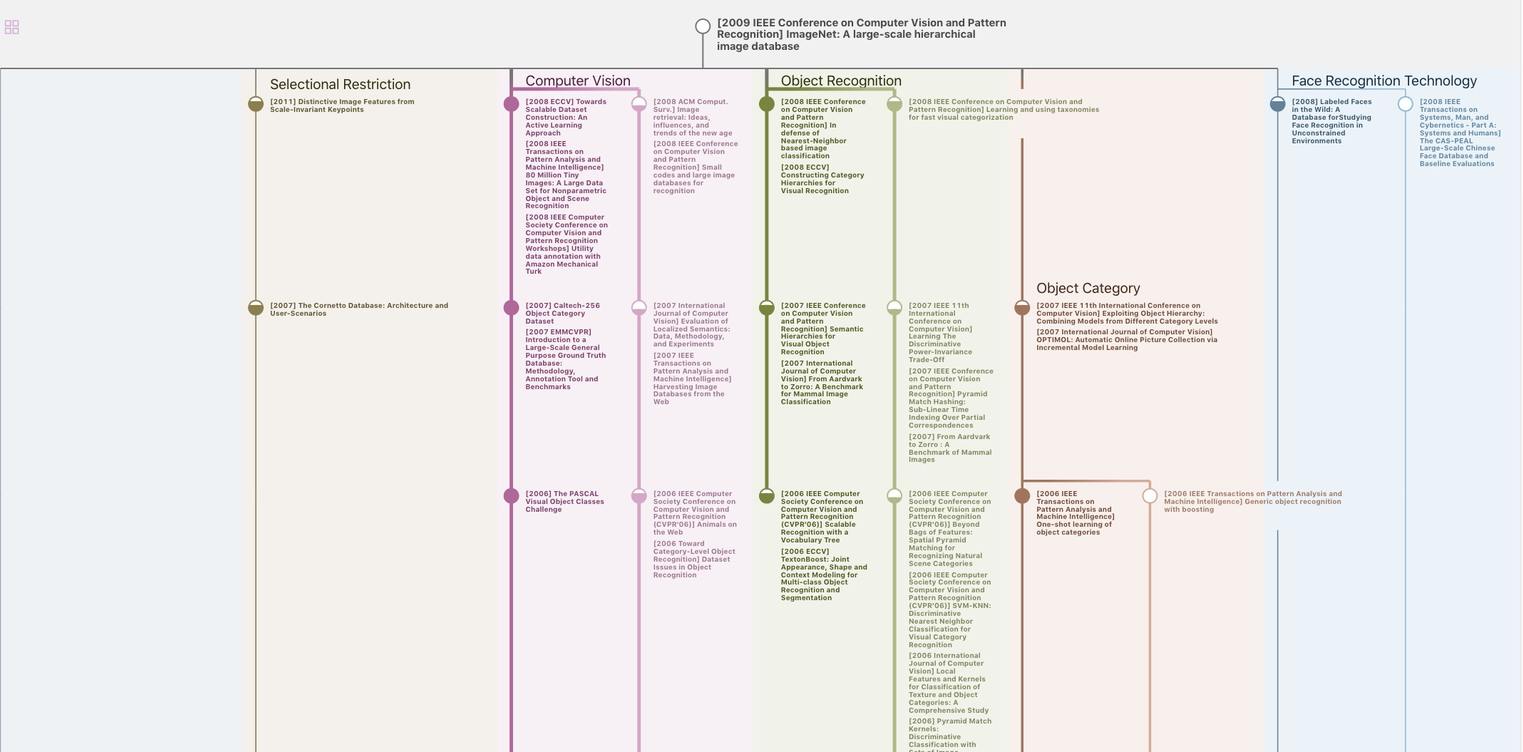
生成溯源树,研究论文发展脉络
Chat Paper
正在生成论文摘要