Change Detection of High-Resolution Remote Sensing Image Based on Semi-Supervised Segmentation and Adversarial Learning.
IEEE International Geoscience and Remote Sensing Symposium (IGARSS)(2022)
摘要
Change detection, which gives a quantitative analysis of the change information for the target area, is an important technology for lots of remote sensing tasks. Supervised change detection methods can achieve satisfactory performance when there are enough labeled samples, which is a harsh requirement for change detection tasks using remote sensing images. To solve this problem, we propose a change detection method based on semi-supervised segmentation and adversarial learning. The proposed method can learn knowledge from both the limited labeled samples and the abundant unlabeled samples to improve the generalization performance of the model. Firstly, the segmentation maps of both labeled samples and unlabeled samples are obtained through a segmentation network. Then, the labeled samples is used to train the discriminator network, which is responsible for distinguishing the segmentation prediction from the ground truth. Finally, the discriminator output is used as a measurement for self-training to minimize the feature difference between the segmentation prediction and the ground truth. Experimental results on the Sun Yat-Sen University (SYSU) dataset show that the proposed method can use unlabeled samples to improve the quality of prediction, which guarantees the proposed method can achieve good performance with few labeled samples.
更多查看译文
关键词
remote sensing,detection,change,high-resolution,semi-supervised
AI 理解论文
溯源树
样例
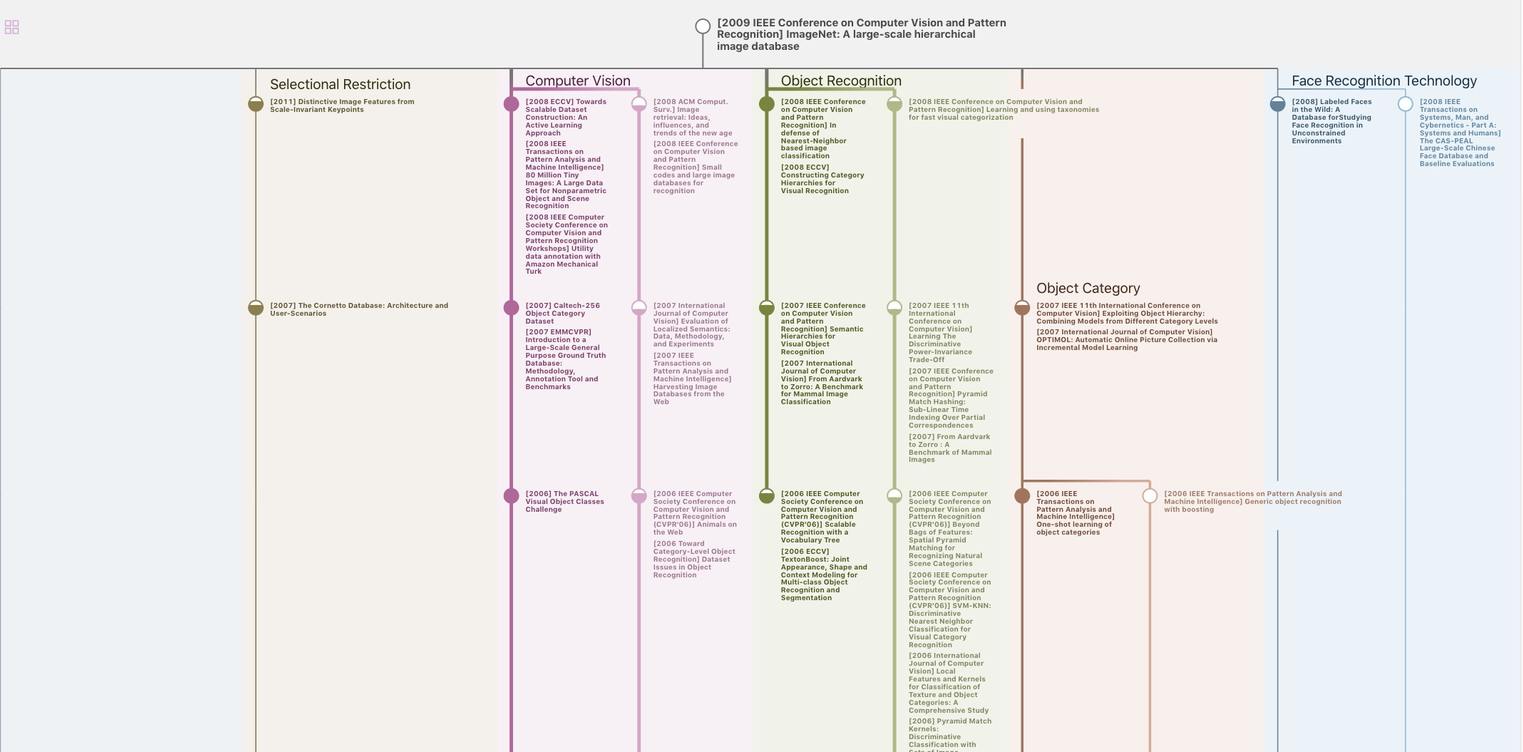
生成溯源树,研究论文发展脉络
Chat Paper
正在生成论文摘要