Compact Feature Representation for Unsupervised Ood Detection
IEEE International Geoscience and Remote Sensing Symposium (IGARSS)(2022)
摘要
Distributional mismatch between training and test data may cause the remote sensing models to behave in unpredictable manner, thus reducing the trustworthiness of such models. Most existing methods for out-of-distribution (OOD) detection rely on availability of OOD samples during training. However, access to OOD data during training is counter intuitive and may be impractical sometimes. Considering this, we propose an unsupervised OOD detection model that does not require training OOD data. The proposed method works by projecting the in-domain samples as a union of 1-dimensional subspaces. Due to the compact feature representation of in-domain samples, OOD samples are less likely to occupy the same feature space, thus they are easily identified. Experimental results demonstrate the capability of the proposed method to detect OOD samples.
更多查看译文
关键词
Uncertainty, out-of-distribution, trustworthiness, deep learning, unsupervised learning, remote sensing
AI 理解论文
溯源树
样例
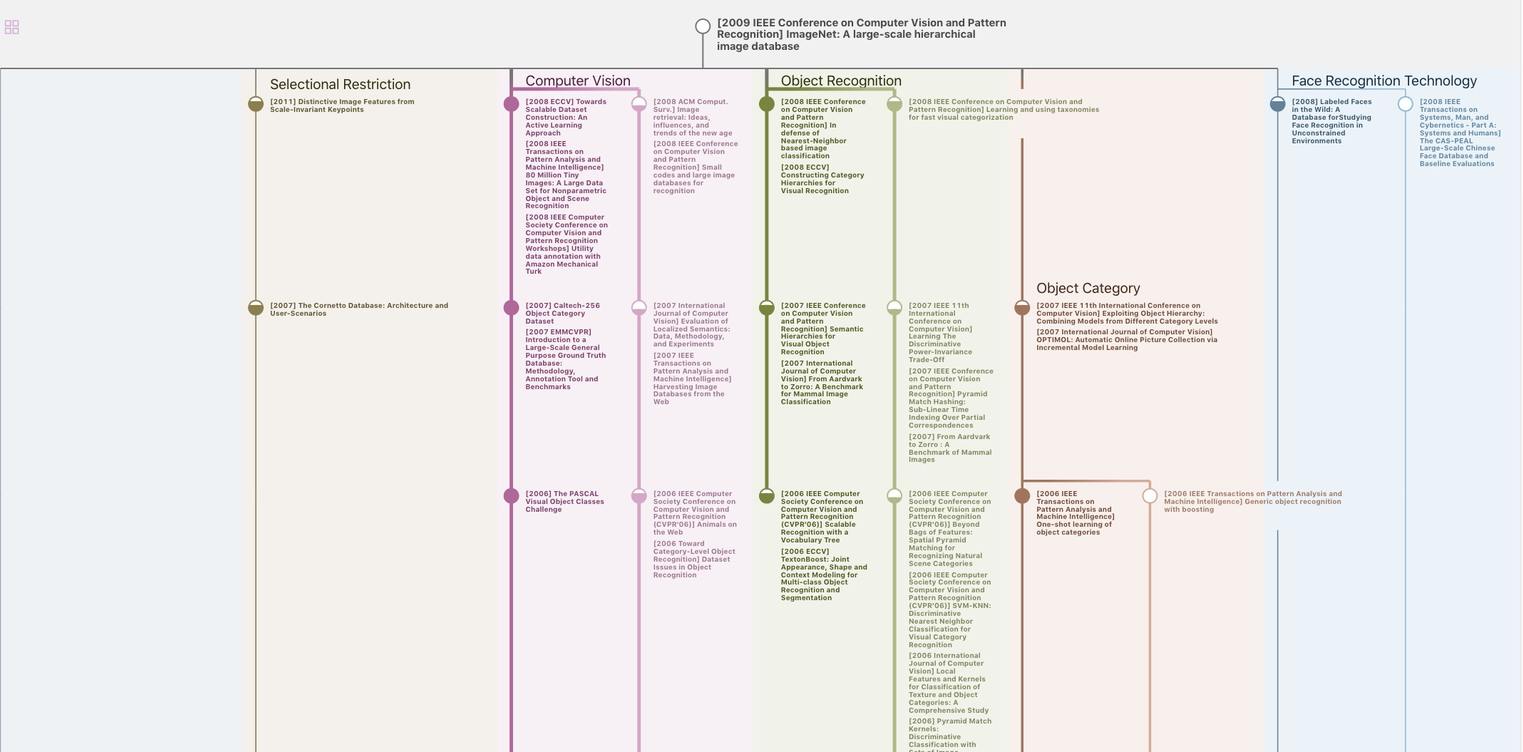
生成溯源树,研究论文发展脉络
Chat Paper
正在生成论文摘要