Serially Disentangled Learning for Multi-Layered Neural Networks.
International Conference on Industrial, Engineering and Other Applications of Applied Intelligent Systems (IEA/AIE)(2022)
摘要
The present paper aims to propose a new type of learning method called "serially disentangled learning." This method is inspired by representation learning, but our focus is not on the production of the disentangled representation but on the computational procedures themselves to make it possible to produce the representation. We try to separate or disentangle several contradictory computational procedures and to force them to perform their tasks serially and independently, meaning that we try to resolve, though seemingly, the contradiction among computational procedures. This concept can be used to disentangle complex information control procedures, where information minimization and maximization procedures are forced to perform each procedure separately, independently, and serially. The method was applied to relations between the mission statements of companies and their profitability, where conventional learning methods could not show better generalization performance. The new method with de-hierarchical disentanglement by network compression produced compressed weights close to the point-biserial correlation coefficients between inputs and targets of the original data set. In addition, we could detect an input related to improved generalization.
更多查看译文
关键词
disentangled learning,neural networks,multi-layered
AI 理解论文
溯源树
样例
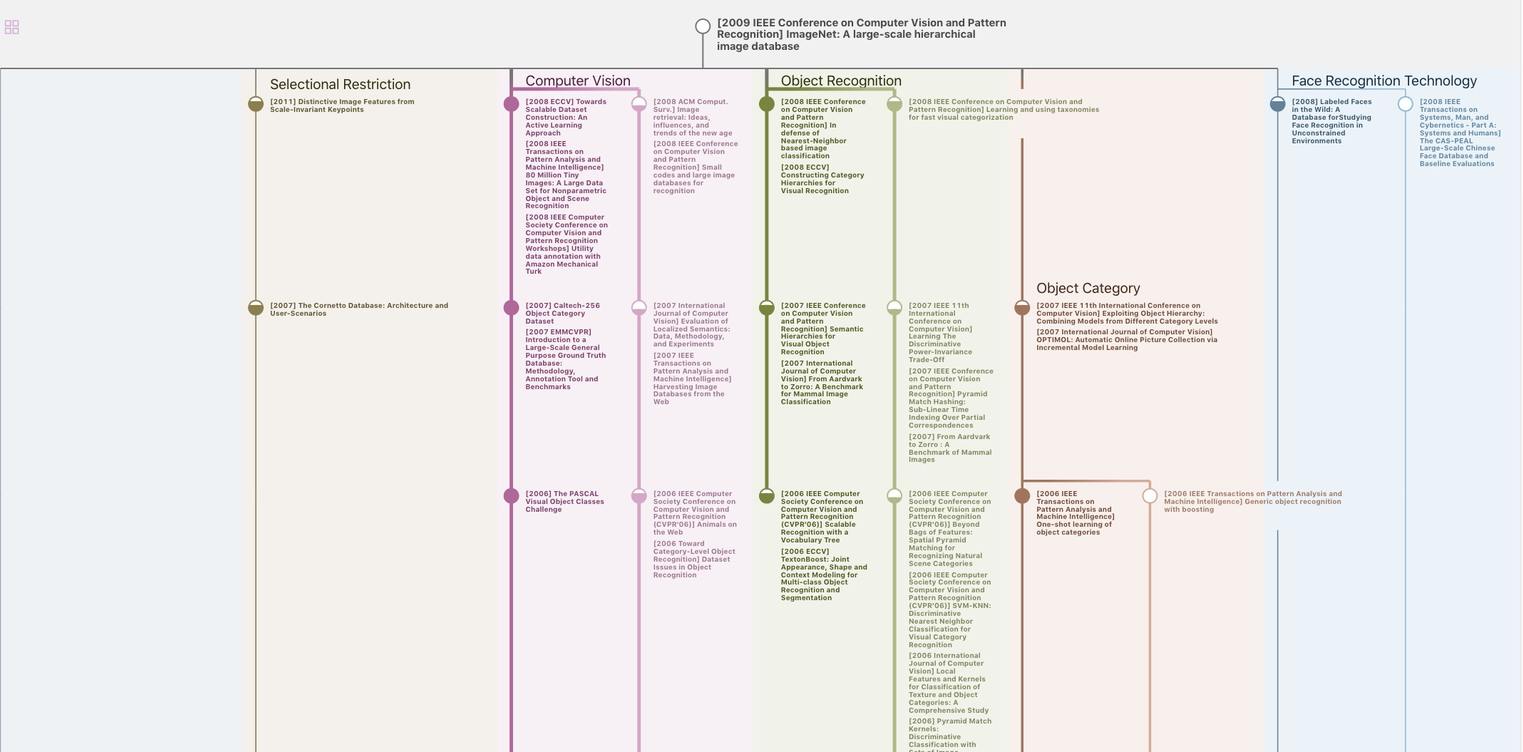
生成溯源树,研究论文发展脉络
Chat Paper
正在生成论文摘要