Unsupervised Cell Segmentation in Fluorescence Microscopy Images via Self-supervised Learning.
International Conferences on Pattern Recognition and Artificial Intelligence (ICPRAI)(2022)
摘要
Cell segmentation in microscopy images is challenging particularly when only few or no annotations available. Existing unsupervised deep learning-based segmentation methods rely on large data sets to train large networks, use synthetic training data, pre-trained networks for domain adaptation, or exploit labels to further train pre-trained networks. We propose an unsupervised deep learning method which is trained from scratch by self-supervised learning without requiring any segmentation labels. Our deep neural network generates an attention map and performs the auxiliary task in one network. The segmentation result is directly obtained from the network, and model selection is performed unsupervised based on the behavior of the loss function during training. We applied our approach to two different fluorescence microscopy data sets and achieved generally better or similar results than classical unsupervised segmentation methods. Furthermore, we compared our method to a supervised method trained with a different number of labels, as well as a semi-supervised version of our method where we select the model based on few annotations.
更多查看译文
关键词
fluorescence microscopy images,cell,learning,self-supervised
AI 理解论文
溯源树
样例
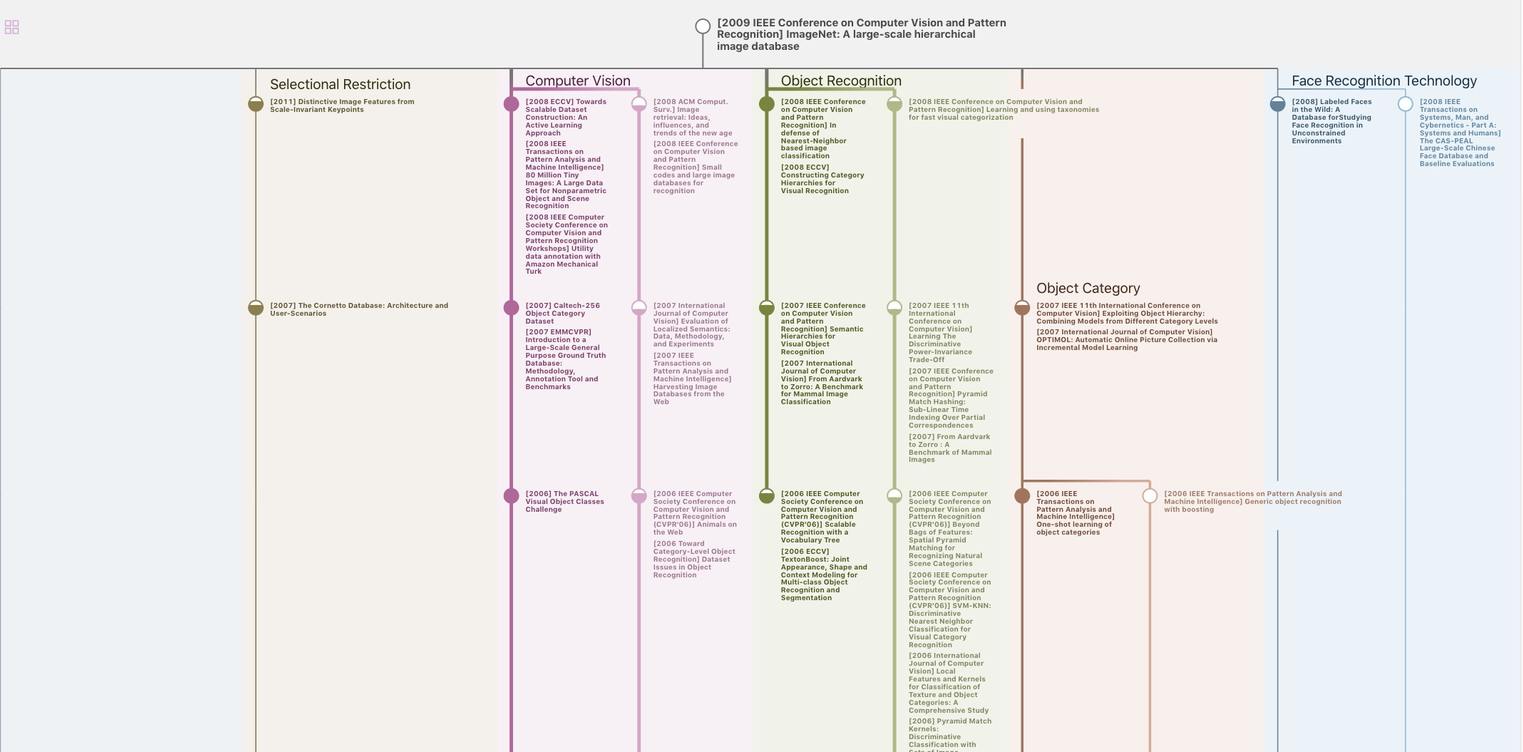
生成溯源树,研究论文发展脉络
Chat Paper
正在生成论文摘要