A general approach to fast online training of modern datasets on real neuromorphic systems without backpropagation
International Conference on Neuromorphic Systems (ICONS)(2022)
摘要
We present parameter-multiplexed gradient descent (PMGD), a perturbative gradient descent framework designed to easily train emergent neuromorphic hardware platforms. We show its applicability to both analog and digital systems. We demonstrate how to use it to train networks with modern machine learning datasets, including Fashion-MNIST and CIFAR-10. Assuming realistic timescales and hardware parameters, our results indicate that PMGD could train a network on emerging hardware platforms orders of magnitude faster than the wall-clock time of training via backpropagation on a standard GPU/CPU.
更多查看译文
关键词
machine learning, neural networks, neuromorphic computing, emerging hardware
AI 理解论文
溯源树
样例
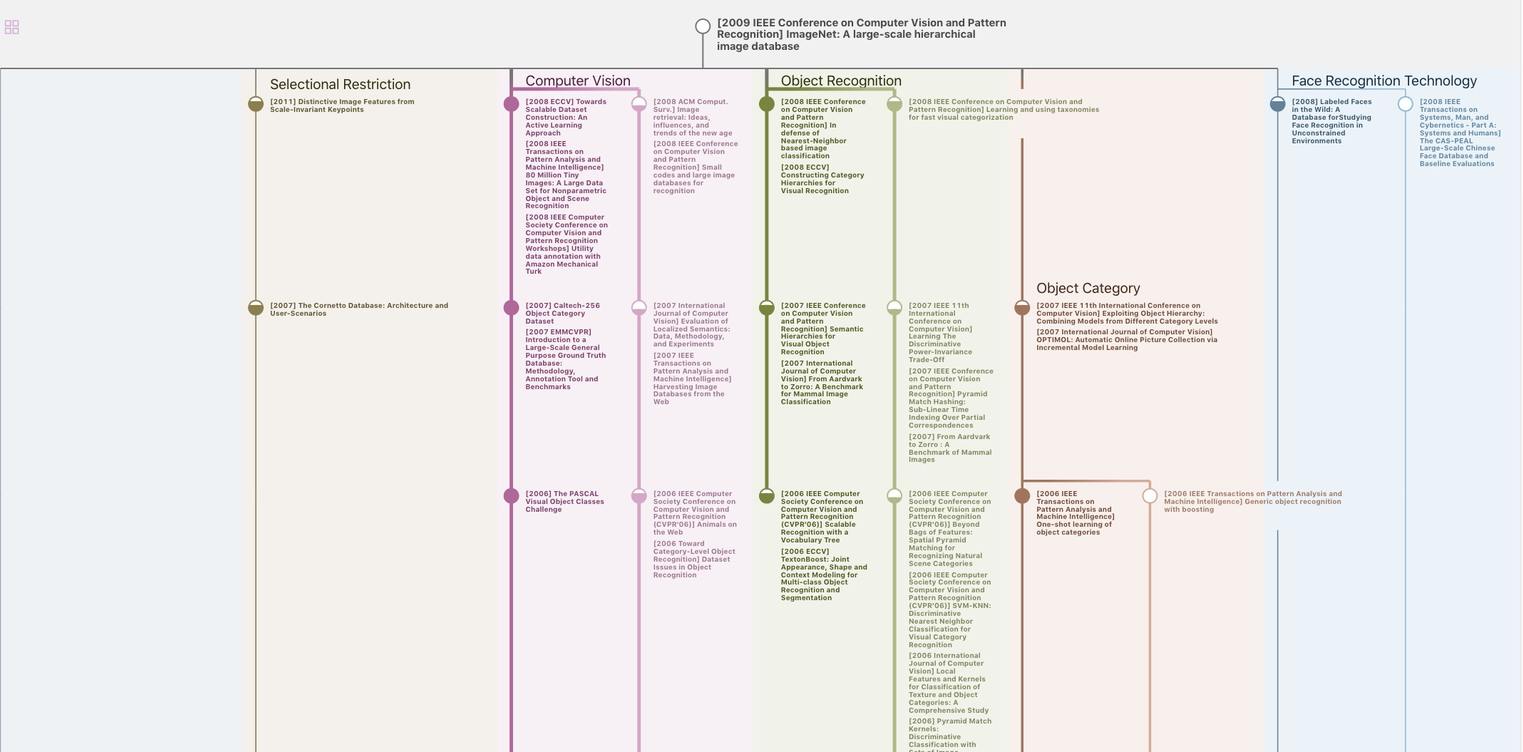
生成溯源树,研究论文发展脉络
Chat Paper
正在生成论文摘要