Investigating Transformer Encoders and Fusion Strategies for Speech Emotion Recognition in Emergency Call Center Conversations.
International Conference on Multimodal Interaction (ICMI)(2022)
摘要
There has been growing interest in using deep learning techniques to recognize emotions from speech. However, real-life emotion datasets collected in call centers are relatively rare and small, making the use of deep learning techniques quite challenging. This research focuses on the study of Transformer-based models to improve the speech emotion recognition of patients’ speech in French emergency call center dialogues. The experiments were conducted on a corpus called CEMO, which was collected in a French emergency call center. It includes telephone conversations with more than 800 callers and 6 agents. Four emotion classes were selected for these experiments: Anger, Fear, Positive and Neutral state. We compare different Transformer encoders based on the wav2vec2 and BERT models, and explore their fine-tuning as well as fusion of the encoders for emotion recognition from speech. Our objective is to explore how to use these pre-trained models to improve model robustness in the context of a real-life application. We show that the use of specific pre-trained Transformer encoders improves the model performance for emotion recognition in the CEMO corpus. The Unweighted Accuracy (UA) of the french pre-trained wav2vec2 adapted to our task is 73.1%, whereas the UA of our baseline model (Temporal CNN-LSTM without pre-training) is 55.8%. We also tested BERT encoders models: in particular FlauBERT obtained good performance for both manual 67.1% and automatic 67.9% transcripts. The late and model-level fusion of the speech and text models also improve performance (77.1% (late) - 76.9% (model-level)) compared to our best speech pre-trained model, 73.1% UA. In order to place our work in the scientific community, we also report results on the widely used IEMOCAP corpus with our best fusion strategy, 70.8% UA. Our results are promising for constructing more robust speech emotion recognition system for real-world applications.
更多查看译文
关键词
emergency call center conversations,speech emotion recognition,transformer encoders
AI 理解论文
溯源树
样例
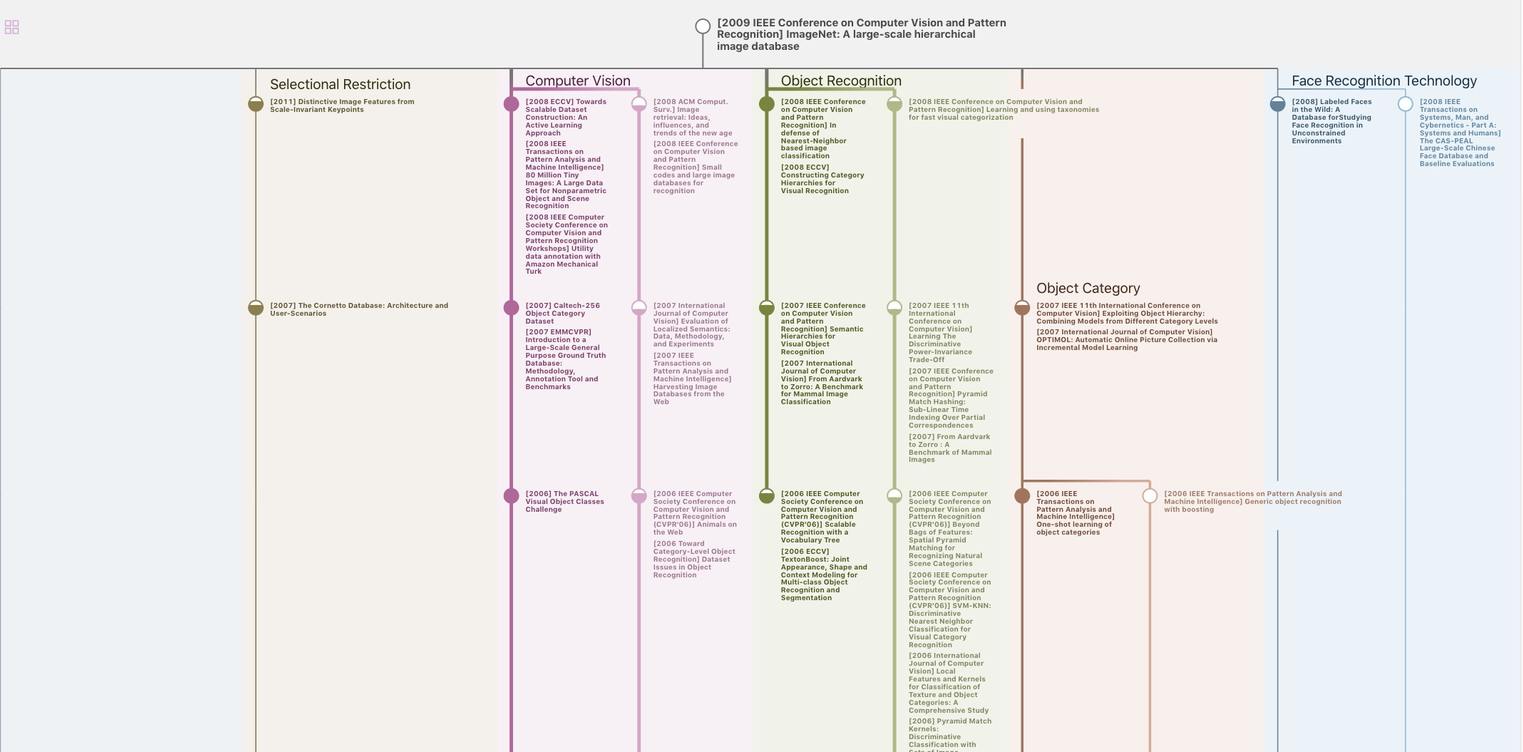
生成溯源树,研究论文发展脉络
Chat Paper
正在生成论文摘要