MAU-Net: A Multiscale Attention Encoder-decoder Network for Liver and Liver-tumor Segmentation.
International Conference on Image and Graphics Processing (ICIGP)(2022)
摘要
U-Net and improved U-Nets suffer from two problems for liver and liver-tumor segmentation. The first is that skip connections in encoder-decoder networks bring interference information. The second is that the convolutional kernel with the fixed receptive field does not match the liver-tumor with changing shape and position. To address the above problems, we propose a multiscale attention encoder-decoder network (MAU-Net) for liver and liver-tumor segmentation. First, MAU-Net employs self-attentive gating guidance module in the skip connection to suppresses irrelevant regions. Secondly, MAU-Net employs a multi-branch feature fusion module to extract multiscale features for the segmentation of liver-tumor. We evaluate the proposed method on the public LiTS dataset. The experimental results show that the average dice of liver and liver-tumor segmentation by MAU-Net are 96.11% and 86.90%, respectively. Experiments demonstrate that MAU-Net is superior to state-of-the-art networks for liver and liver-tumor segmentation.
更多查看译文
AI 理解论文
溯源树
样例
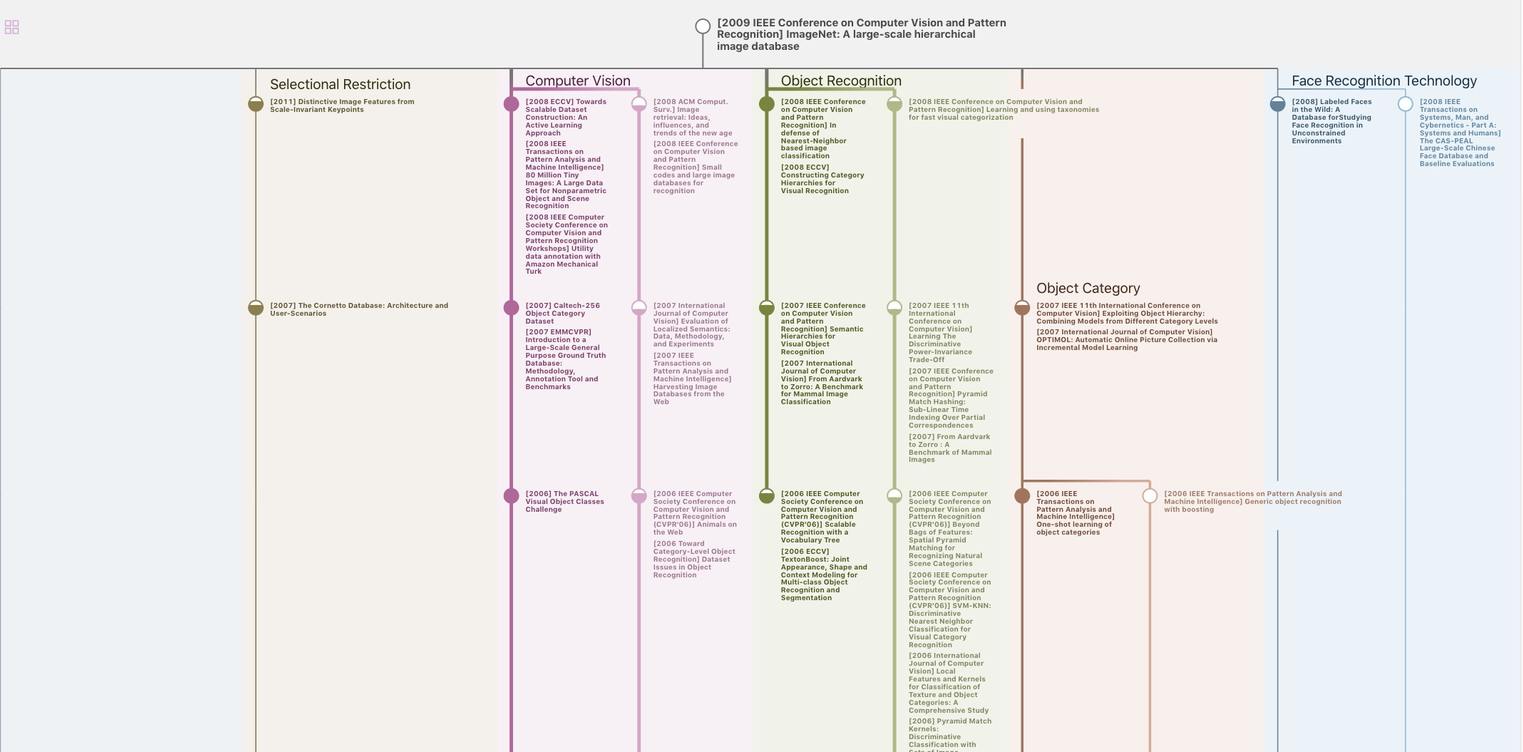
生成溯源树,研究论文发展脉络
Chat Paper
正在生成论文摘要