Compressing Audio Visual Speech Recognition Models With Parameterized Hypercomplex Layers.
Hellenic Conference on Artificial Intelligence (SETN)(2022)
摘要
Audio visual speech recognition has seen remarkable progress in the last few years. This progress is a result, on the one hand, of advances in deep learning-based architectures, such as convolutional and recurrent neural networks, and, on the other hand, due to large-scale public datasets have been introduced that provide a great variety of speakers. Both factors have led authors to develop deep architectures that achieve impressive results that surpass humans in the areas of speech recognition, especially in cases where only the video is present. Nevertheless, these architectures involve millions of parameters that increase their storage and memory demands and also limit their deployment in resource constrained scenarios. An additional issue is the energy expenditure due to the amount of calculations required for training, fine-tuning and testing. In this work, we attempt to mitigate some of these shortcomings in speech recognition models by incorporating parameterized hypercomplex layers that reduce the number of required resources. We present models that are competitive with the state-of-the-art while operating with fewer parameters.
更多查看译文
关键词
parameterized hypercomplex layers,audio,models
AI 理解论文
溯源树
样例
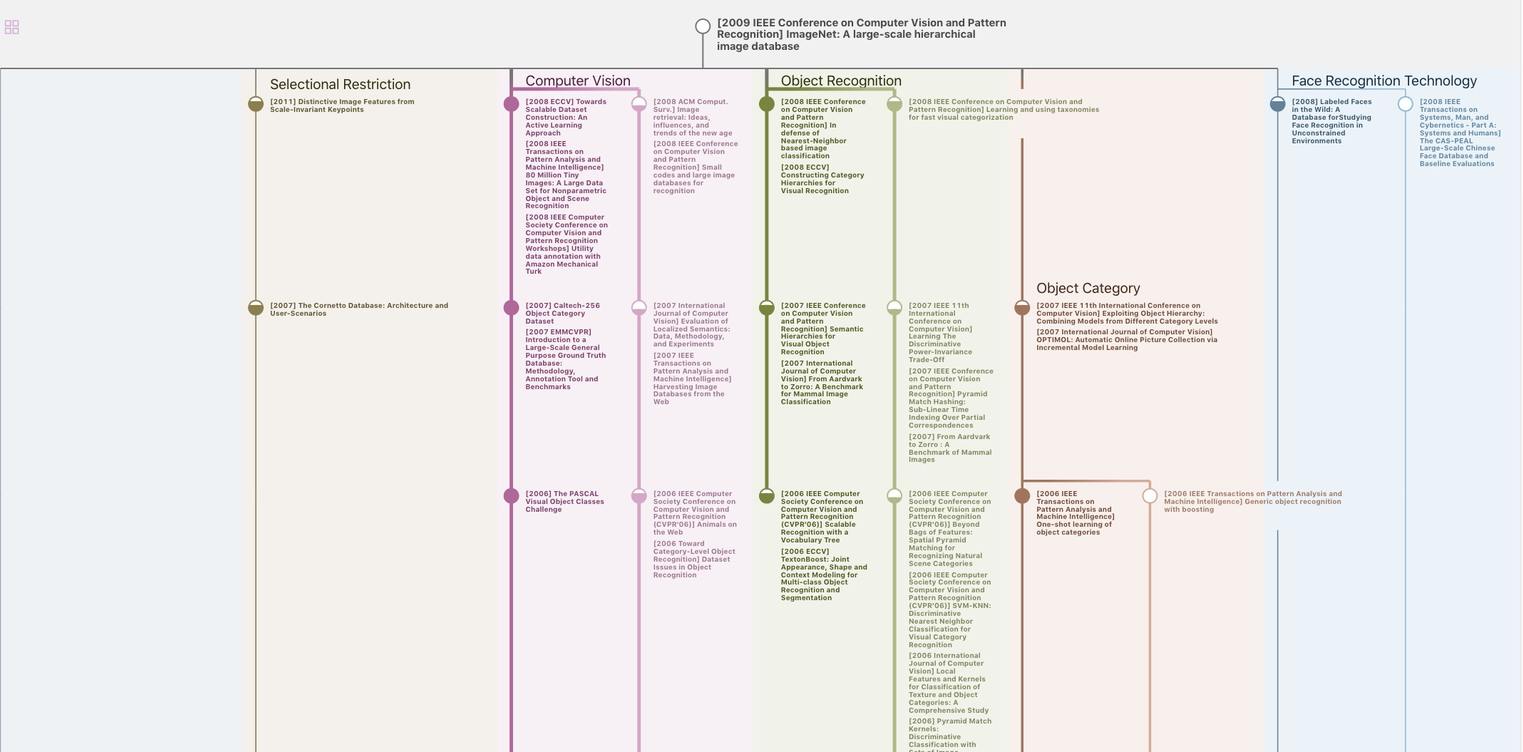
生成溯源树,研究论文发展脉络
Chat Paper
正在生成论文摘要