Improving EEG-based Motor Execution Classification for Robot Control.
International Conference on Human-Computer Interaction (HCI International)(2022)
摘要
Brain Computer Interface (BCI) systems have the potential to provide a communication tool using non-invasive signals which can be applied to various fields including neuro-rehabilitation and entertainment. Interpreting multi-class movement intentions in a real time setting to control external devices such as robotic arms remains to be one of the main challenges in the BCI field. We propose a learning framework to decode upper limb movement intentions before and during the movement execution (ME) with the inclusion of motor imagery (MI) trials. The design of the framework allows the system to evaluate the uncertainty of the classification output and respond accordingly. The EEG signals collected during MI and ME trials are fed into a hybrid architecture consisting of Convolutional Neural Networks (CNN) and Long Short-Term Memory (LSTM) with limited pre-processing. Outcome of the proposed approach shows the potential to anticipate the intended movement direction before the onset of the movement, while waiting to reach a certainty level by potentially observing more EEG data from the beginning of the actual movement before sending control commands to the robot to avoid undesired outcomes. Presented results indicate that both the accuracy and the confidence level of the model improves with the introduction of MI trials right before the movement execution. Our results confirm the possibility of the proposed model to contribute to real-time and continuous decoding of movement directions for robotic applications.
更多查看译文
关键词
motor execution classification,robot control,eeg-based
AI 理解论文
溯源树
样例
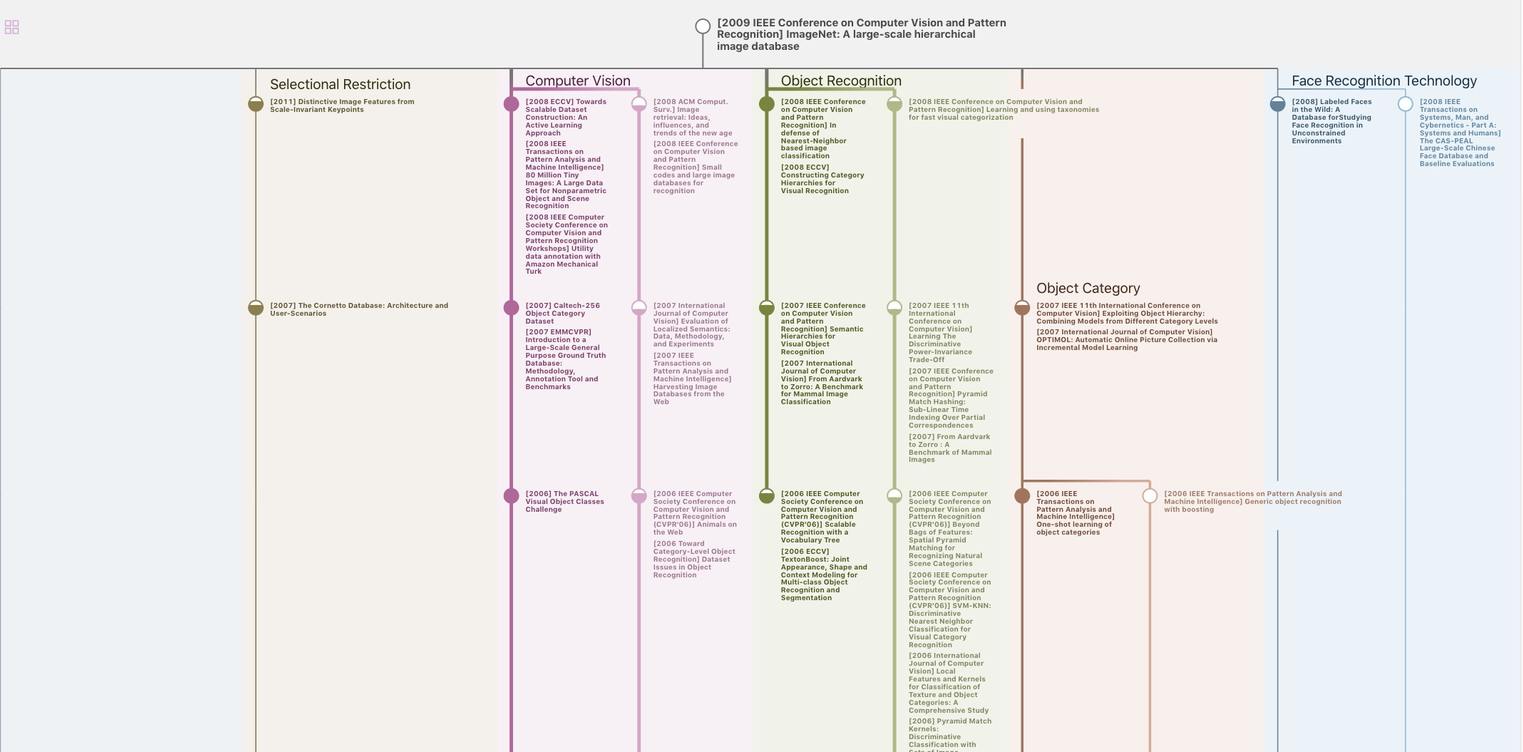
生成溯源树,研究论文发展脉络
Chat Paper
正在生成论文摘要