Image Schemas and Image Schematic Complexes: Enhancing Neural Machine Translation Networks.
International Conference on Computational and Corpus-Based Phraseology (Europhras)(2022)
摘要
Machine Translation (MT) is a Natural Language Processing (NLP) application which has taken off and reported considerable progress in recent years. Most recent applications of MT employ neural networks imitating the principles of human understanding and creation of meaning at conceptual and cognitive levels (Nerlich and Clarke 2000: 141). They are based on techniques which try to simulate the mechanisms of learning in biological organisms carried out through the neurons (Aggarwal 2018: 1; Theordoris 2020: 903). However, human intelligence and the cognitive models which humans use through language should be subject to more examination in order to have the capacity to unveil more basic cognitive features. For this reason, a more holistic understanding of certain aspects of human intelligence based on cognitive models is still required in order to take machine learning a step further (Goertzel et al. 2012: 124). Image schemas and image schematic complexes are among the cognitive issues which would benefit from further studies as its basic structure is fundamental in natural language processing and conceptualisation (Hedblom et al. 2019). They are common in all languages and all cultures but their use is not always universal. This variation influences the quality of MT, as in some cases, this variance is not taken into consideration while feeding the neural networks of MT. This preliminary study has the objective of studying the novel idea of image schemas and image schematic complexes and proposing an applied methodology to use them in MT.
更多查看译文
关键词
Image schemas,Cognitive models,Machine translation
AI 理解论文
溯源树
样例
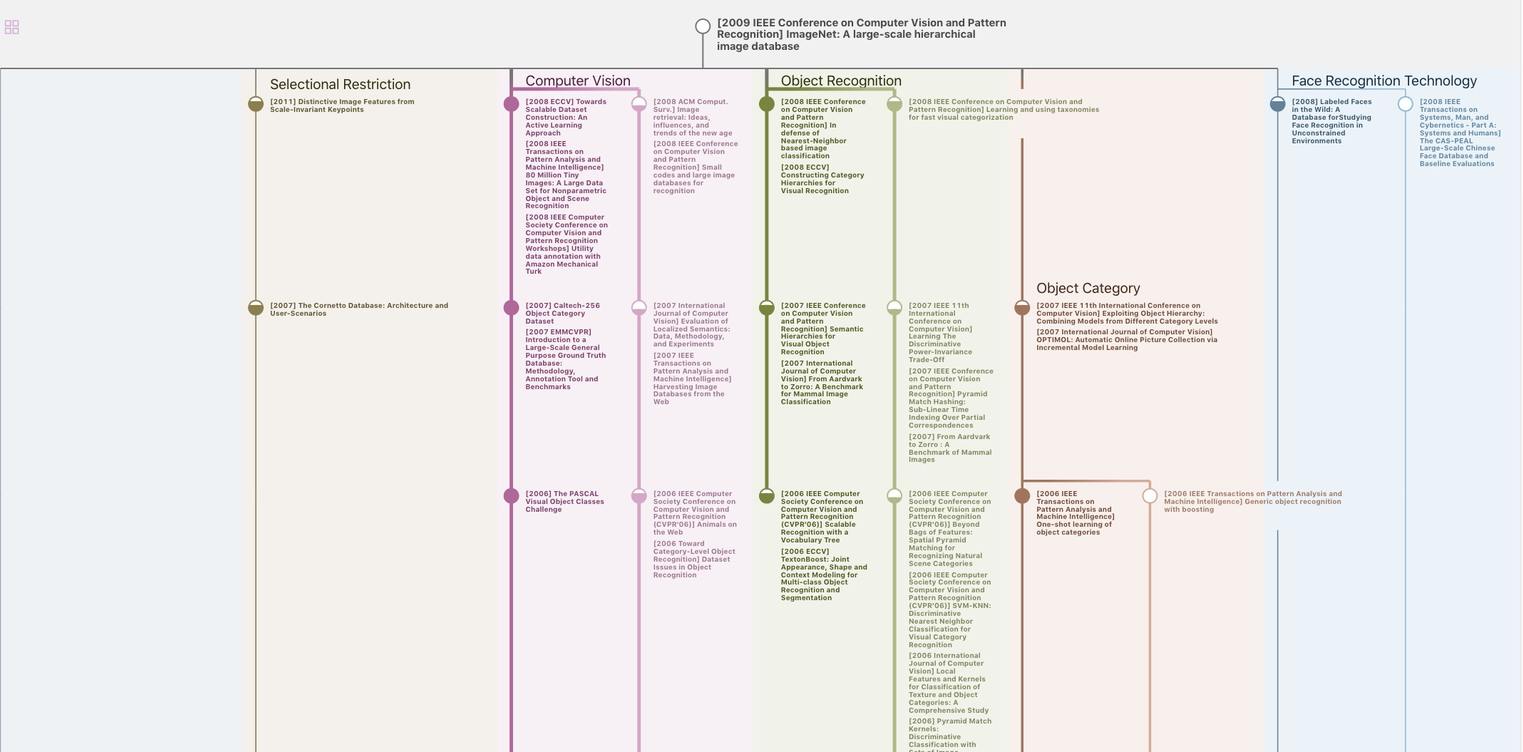
生成溯源树,研究论文发展脉络
Chat Paper
正在生成论文摘要