Fast Sampling for Large-scale Linear Inverse Problems via Enlarging Principle Submatrix.
European Signal Processing Conference (EUSIPCO)(2022)
摘要
Sampling for linear inverse problems is the active selection of partial observations so that the entire physical signal with linear model can be well recovered. In this paper, we will propose a fast sampling algorithm for large-scale linear inverse problems. Specifically, assuming that the field signal f is represented by a linear model f = Phi(g), it can be estimated from partial noisy samples via an unbiased least-squares (LS) method, whose expected mean square error (MSE) depends on chosen samples. First, we formulate an approximate MSE problem, and then prove it is equivalent to an optimization of a principle submatrix of Phi Phi(+) indexed by sample set. By enlarging the submatrix greedily, we solve the proposed combinatorial problem with simple matrix-vector multiplications, leveraging a matrix inverse lemma. To further reduce complexity, we reuse results in the previous greedy step for warm start, so that candidates can be evaluated via lightweight vector-vector multiplications. Extensive experiments show that our proposed sampling method achieved the least execution time and the best performance compared to state-of-the-art schemes, especially for large-size scenarios.
更多查看译文
关键词
Fast sampling, linear inverse problem, greedy approach
AI 理解论文
溯源树
样例
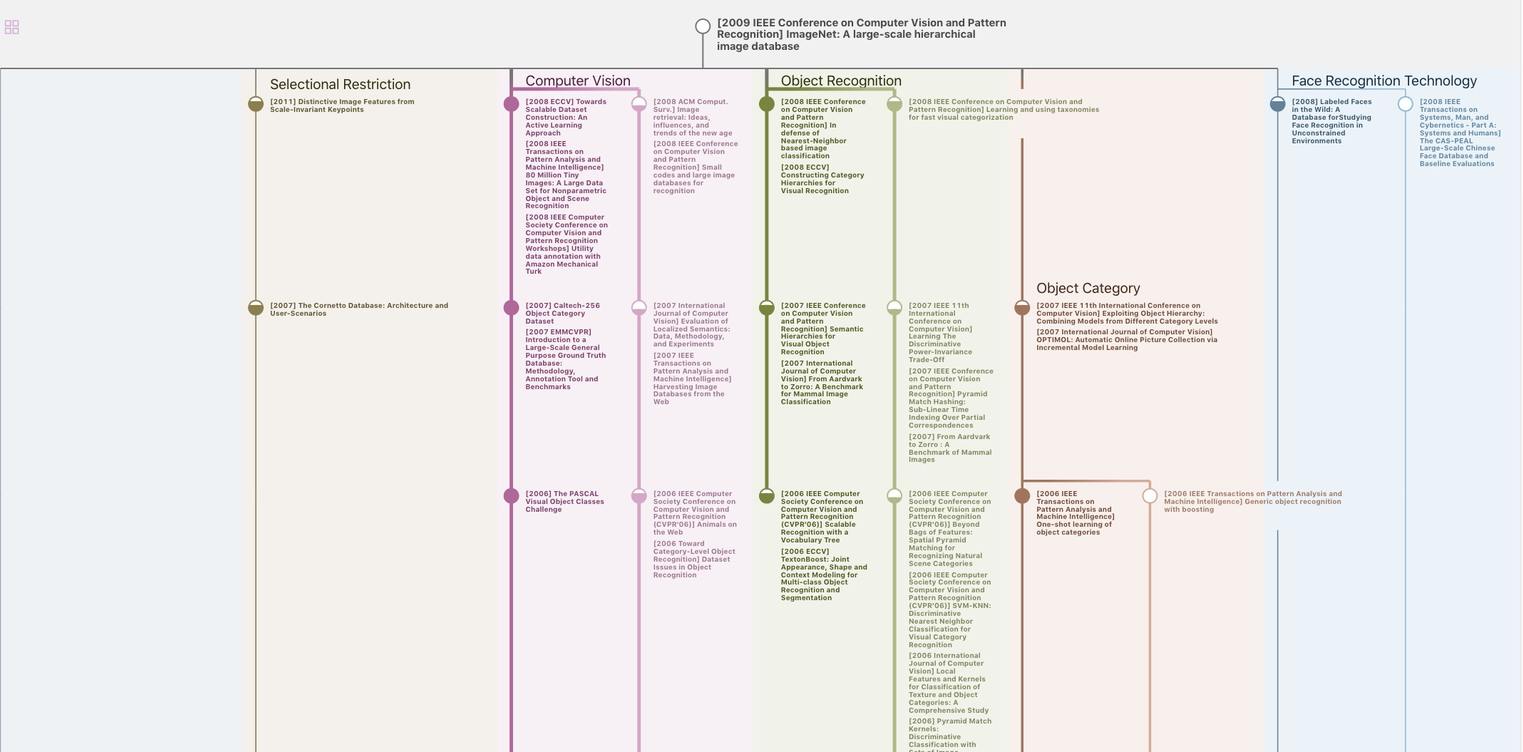
生成溯源树,研究论文发展脉络
Chat Paper
正在生成论文摘要