Fast Quadratic Sensing Via Nonconvex Optimization.
European Signal Processing Conference (EUSIPCO)(2022)
摘要
This paper concerns the problem of recovering an unknown but rank-r signal from quadratic measurements. We formulate the recovery problem as two-stage nonconvex optimization approach where an efficient adaptive accelerated gradient-like iterative refinement starting from a careful initialization. Moreover, an efficient initial scheme is proposed for this problem. The average relative error of our initialization estimator reduces exponentially as the oversampling ratio grows, which can also boost the performance of existing two-stage approach for rank-r matrix recovery from vector measurements. Experimental results not only clearly demonstrate the superiority of introduced initialization estimator but also show the advantages of our adaptive accelerated gradient-like approach in terms of both sample complexity and computational complexity.
更多查看译文
关键词
Adaptive accelerated gradient-like method, low-rank matrix recovery, nonconvex optimization, quadratic sensing
AI 理解论文
溯源树
样例
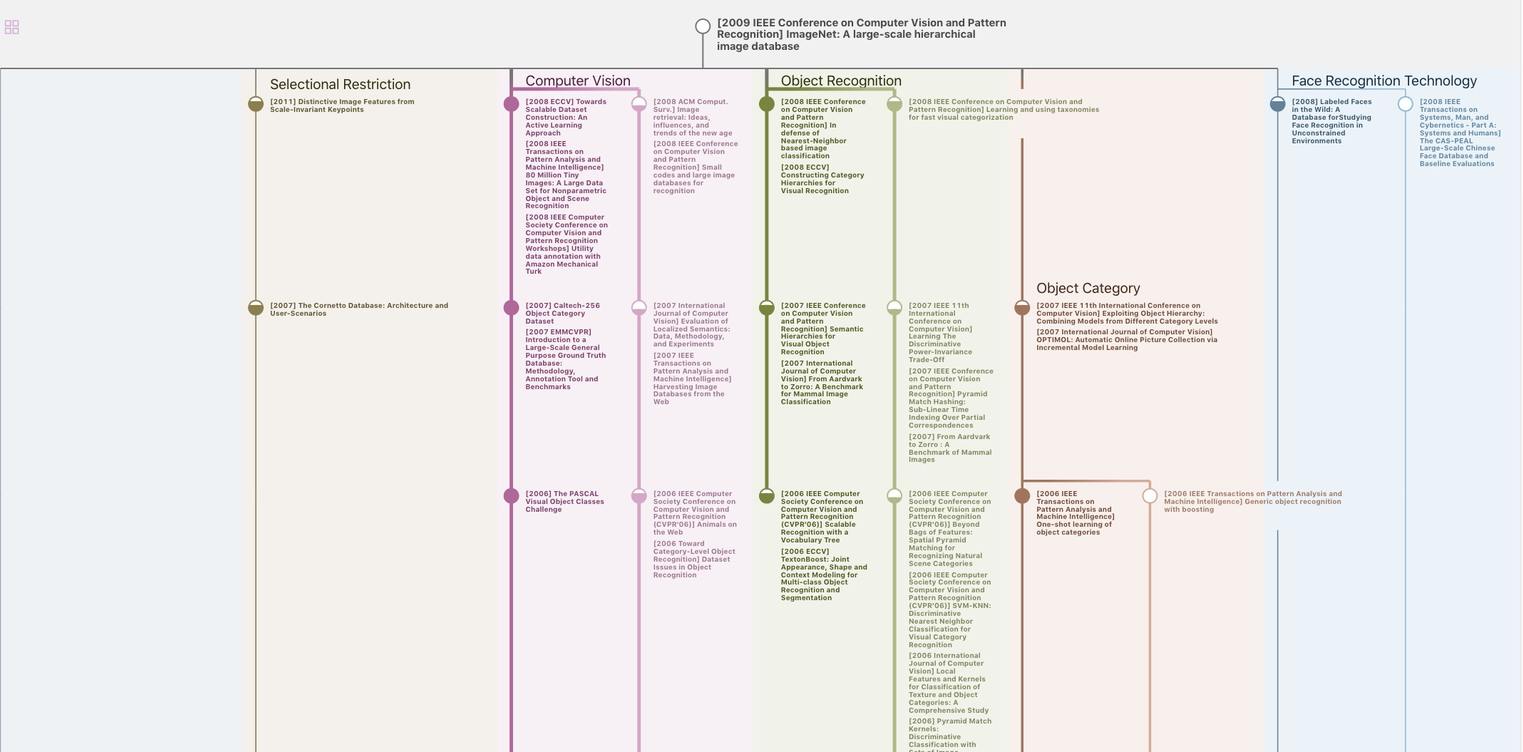
生成溯源树,研究论文发展脉络
Chat Paper
正在生成论文摘要