Secondary Structure Classification of Low-homology Proteins with Graph Neural Networks.
European Signal Processing Conference (EUSIPCO)(2022)
摘要
Acquiring knowledge of the dynamics and properties that force each protein into a unique secondary structure is highly important in medicine and biotechnology. The majority of existing prediction methods rely on complicated processes to extract numerous representative features that are later paired with state-of-the-art classifiers. A recent study proposes a recurrent neural network architecture demonstrating for the first time the potential of deep learning architectures for secondary structure classification of low-homology proteins. According to the latest state-of-the-art, proteins can be successfully described as graphs for the task of classification providing substantial results while at the same time addressing serious challenges including dealing with datasets with different sample sizes, among others. Thus, this study employs an efficient graph representation of low-homology proteins along with graph neural networks which have exhibited cutting-edge performance in a variety of graph-based applications, including protein sequence classification. Overall, this work proposes a novel, simple and fast architecture based on graph neural networks, with a significantly reduced representative feature set, when compared to the literature, that derives directly through the sequence-to-graph procedure. Experimental evaluation on real protein datasets demonstrates the superiority of our proposed architecture, with respect to accuracy and overall complexity when compared against the state-of-the-art.
更多查看译文
关键词
graph neural networks,proteins,low-homology
AI 理解论文
溯源树
样例
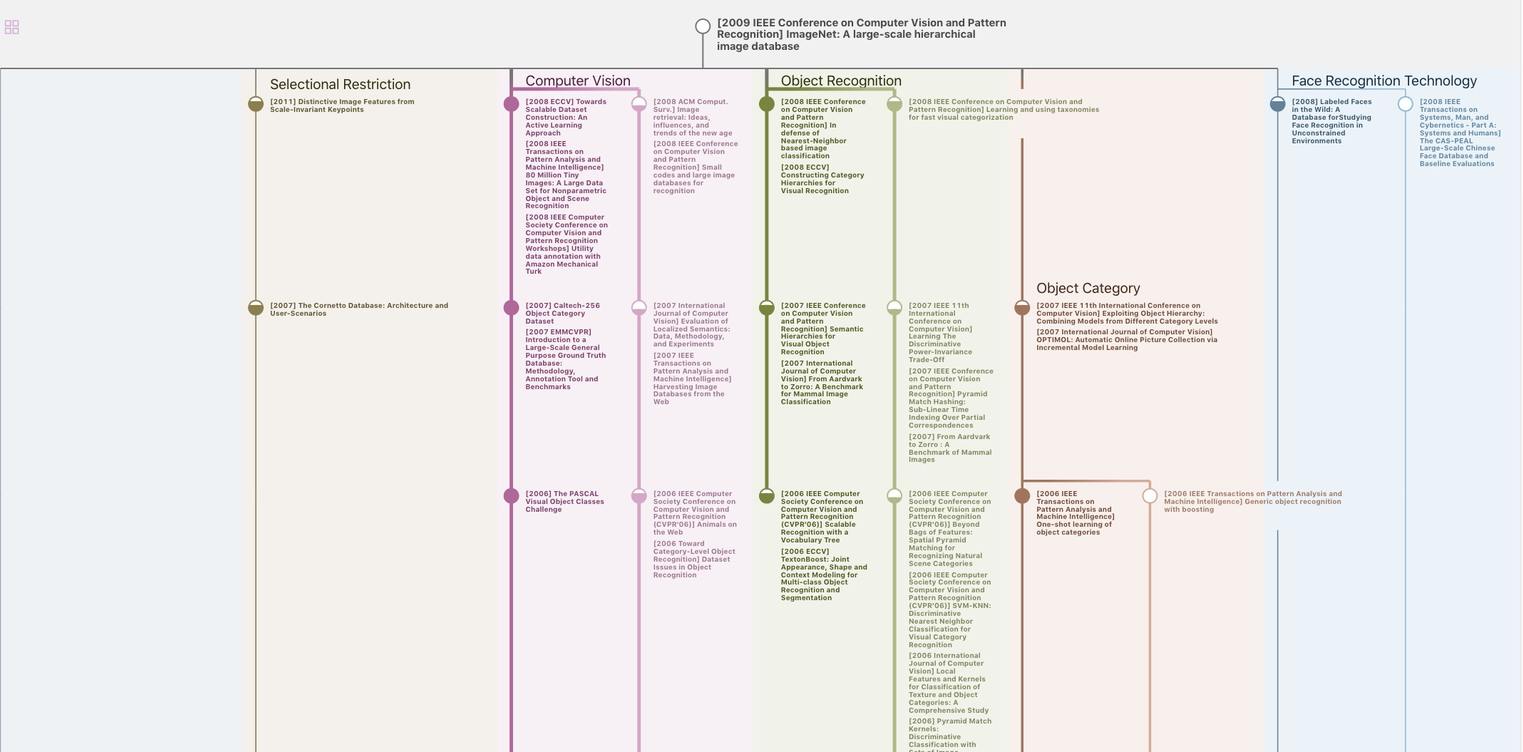
生成溯源树,研究论文发展脉络
Chat Paper
正在生成论文摘要