Transfer Learning Suitability Metric for ANN-based Industrial Controllers.
IEEE International Conference on Emerging Technologies and Factory Automation (ETFA)(2022)
摘要
In the last years, the industrial digitalisation and the Industry 4.0 paradigm is no longer a fairy-tale but a reality. It is becoming more common to find industrial environments relying and adopting data-based approaches to perform some sorts of processes. Some of them are related to the industrial control, where the incursion of Artificial Neural Networks (ANNs) is promoting the usage of data-based solutions to substitute conventional control structures. Besides, one of the greatest issues related to the ANN time-consuming training process has been alleviated by means of Transfer Learning (TL) methods. However, in the industrial control domain TL cannot be freely adopted since the final performance of the transferred control structure cannot be known before substituting the conventional structure. This is an issue that needs to be tackled, especially in critical industrial scenarios where an incorrect control can produce huge disasters. For that reason we present here the Transfer Suitability Metric (TSM). Based on the environments similarities, its main aim is to compute the transference suitability of ANN-based controllers in order to transfer the ANN to the target domain without resorting to new control design and optimization. It provides the plant operators with an insight of the controller behaviour before it is finally substituting the conventional control structure. Results have shown that the metric is highly correlated with the final control behaviour in the sense that the higher the metric, the better the final ANN-based controller performance.
更多查看译文
关键词
Artificial Neural Networks,Industrial Control,Metric,Transfer Learning
AI 理解论文
溯源树
样例
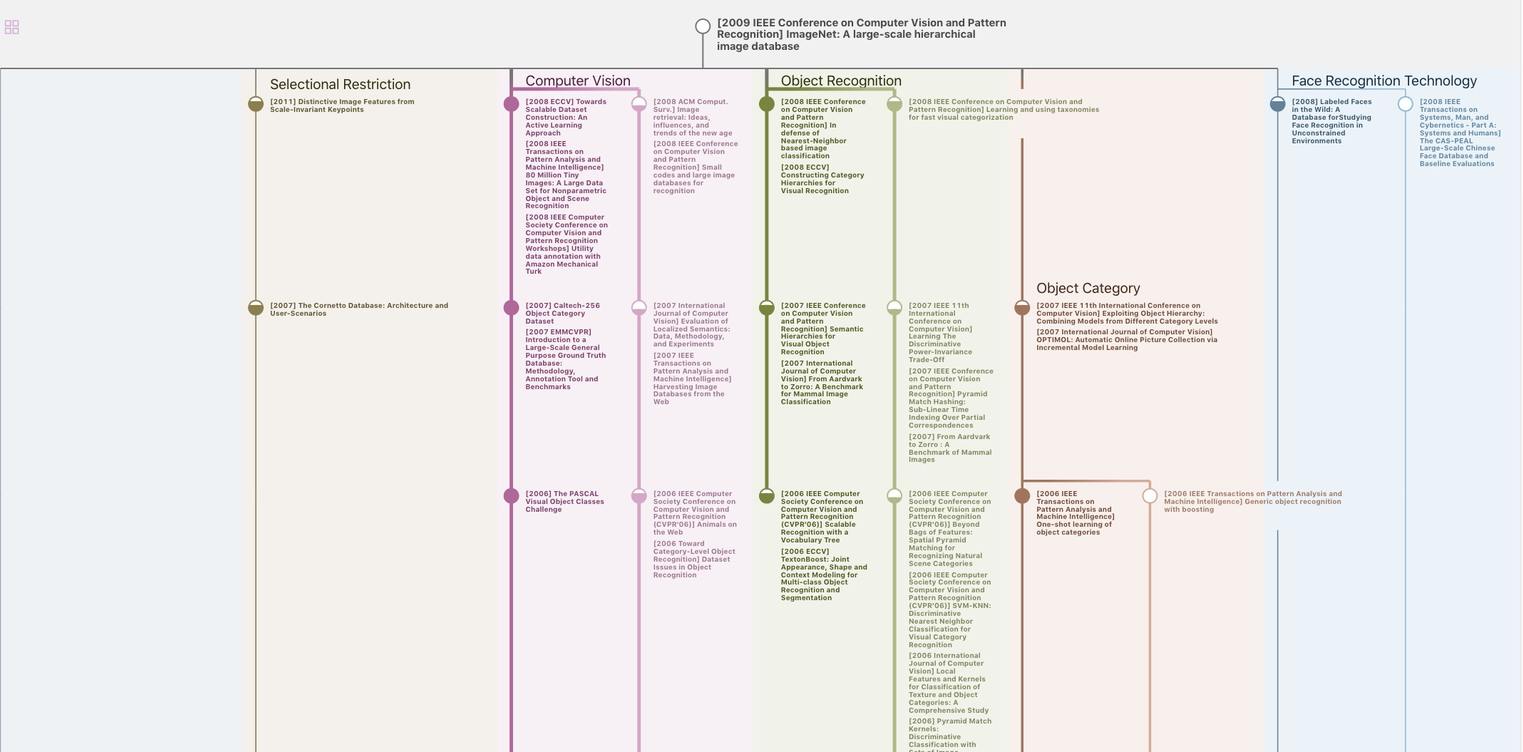
生成溯源树,研究论文发展脉络
Chat Paper
正在生成论文摘要