JUICE: JUstIfied Counterfactual Explanations.
International Conference on Discovery Science (DS)(2022)
摘要
Complex, highly accurate machine learning algorithms support decision-making processes with large and intricate datasets. However, these models have low explainability. Counterfactual explanation is a technique that tries to find a set of feature changes on a given instance to modify the models prediction output from an undesired to a desired class. To obtain better explanations, it is crucial to generate faithful counterfactuals, supported by and connected to observations and the knowledge constructed on them. In this study, we propose a novel counterfactual generation algorithm that provides faithfulness by justification, which may increase developers and users trust in the explanations by supporting the counterfactuals with a known observation. The proposed algorithm guarantees justification for mixed-features spaces and we show it performs similarly with respect to state-of-the-art algorithms across other metrics such as proximity, sparsity, and feasibility. Finally, we introduce the first model-agnostic algorithm to verify counterfactual justification in mixed-features spaces.
更多查看译文
关键词
Machine learning, Interpretability, Counterfactuals, Faithfulness, Justification, Mixed-features space
AI 理解论文
溯源树
样例
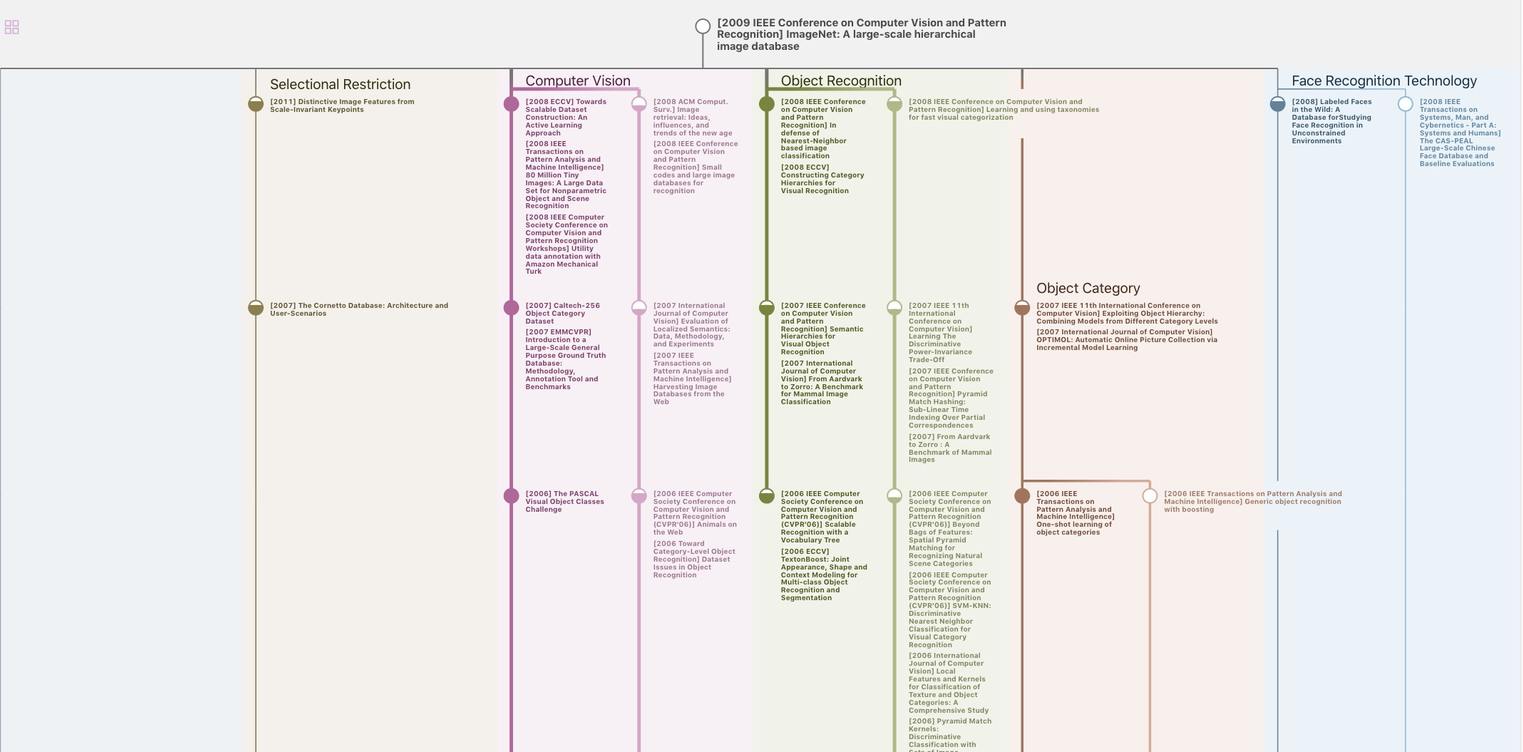
生成溯源树,研究论文发展脉络
Chat Paper
正在生成论文摘要